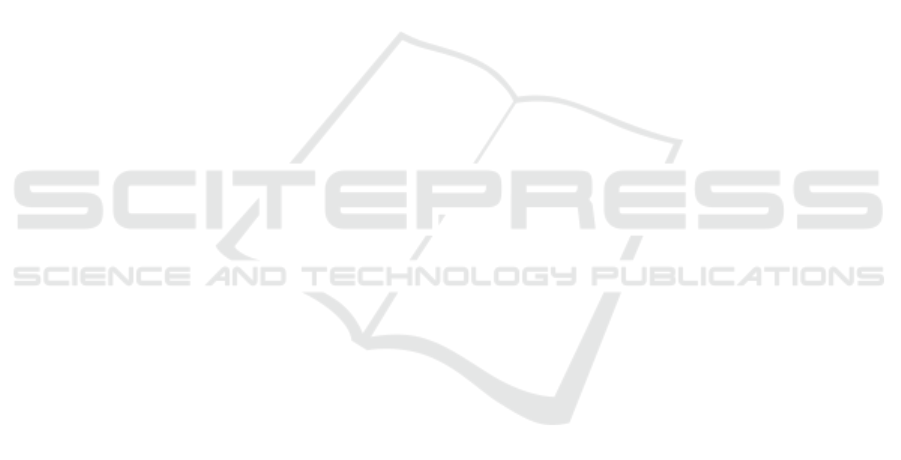
5 CONCLUSIONS AND FUTURE
WORK
In this work, Voronoi diagrams have been used as
the main tool for segmenting indoor scenarios into
rooms. By extracting them from both free and occu-
pied spaces, segmentation results have outperformed
state of the art techniques, with high invariability in
furnished environments. With respect to other meth-
ods, no additional steps have been required to unify
areas after segmenting. Additionally, a method has
been proposed to extract a threshold dependent on the
scenario in which it is being applied, adjusting to the
specific needs of each map.
In future work, it is intended to use the proposed
method for locating doors. By knowing where doors
are, the main challenge is modifying robots behaviour
to facilitate door trespassing.
ACKNOWLEDGEMENTS
This work was supported by the funding from HERO-
ITEA: Heterogeneous Intelligent Multi-Robot Team
for Assistance of Elderly People (RTI2018-095599-
B-C21), funded by Spanish Ministerio de Economia
y Competitividad, and the RoboCity2030 DIH-CM
project (S2018/NMT-4331, RoboCity2030 Madrid
Robotics Digital Innovation Hub).
REFERENCES
Beeson, P., Jong, N. K., and Kuipers, B. (2005). Towards
autonomous topological place detection using the ex-
tended voronoi graph. In Proceedings of the 2005
IEEE ICRA, pages 4373–4379. IEEE.
Bormann, R., Jordan, F., Li, W., Hampp, J., and H
¨
agele,
M. (2016). Room segmentation: Survey, implementa-
tion, and analysis. In 2016 IEEE international confer-
ence on robotics and automation (ICRA), pages 1019–
1026. IEEE.
Buschka, P. and Saffiotti, A. (2002). A virtual sensor for
room detection. In IEEE/RSJ international conference
on intelligent robots and systems, volume 1, pages
637–642. IEEE.
Fabrizi, E. and Saffiotti, A. (2000). Extracting topology-
based maps from gridmaps. In Proceedings 2000
ICRA. Millennium Conference. IEEE International
Conference on Robotics and Automation. Symposia
Proceedings (Cat. No. 00CH37065), volume 3, pages
2972–2978. IEEE.
Fermin-Leon, L., Neira, J., and Castellanos, J. A. (2017).
Incremental contour-based topological segmentation
for robot exploration. In 2017 IEEE ICRA, pages
2554–2561. IEEE.
Friedman, S., Pasula, H., and Fox, D. (2007). Voronoi ran-
dom fields: Extracting topological structure of indoor
environments via place labeling. In IJCAI, volume 7,
pages 2109–2114.
Goeddel, R. and Olson, E. (2016). Learning semantic place
labels from occupancy grids using cnns. In 2016
IEEE/RSJ IROS, pages 3999–4004. IEEE.
Hiller, M., Qiu, C., Particke, F., Hofmann, C., and Thi-
elecke, J. (2019). Learning topometric semantic maps
from occupancy grids. In 2019 IEEE/RSJ Interna-
tional Conference on Intelligent Robots and Systems
(IROS), pages 4190–4197. IEEE.
Hou, J., Yuan, Y., and Schwertfeger, S. (2019). Area graph:
Generation of topological maps using the voronoi di-
agram. In 2019 19th International Conference on Ad-
vanced Robotics (ICAR), pages 509–515. IEEE.
Joo, K., Lee, T.-K., Baek, S., and Oh, S.-Y. (2010). Gener-
ating topological map from occupancy grid-map using
virtual door detection. In IEEE Congress on Evolu-
tionary Computation, pages 1–6. IEEE.
Kleiner, A., Baravalle, R., Kolling, A., Pilotti, P., and
Munich, M. (2017). A solution to room-by-room
coverage for autonomous cleaning robots. In 2017
IEEE/RSJ International Conference on Intelligent
Robots and Systems (IROS), pages 5346–5352. IEEE.
Liu, B., Zuo, L., Zhang, C.-H., and Liu, Y. (2018). An
approach to graph-based grid map segmentation for
robot global localization. In 2018 IEEE International
Conference on Mechatronics and Automation (ICMA),
pages 1812–1817. IEEE.
MathWorks (2022). Morphological operations on binary
images - matlab bwmorph.
Mozos, O. M., Triebel, R., Jensfelt, P., Rottmann, A., and
Burgard, W. (2007). Supervised semantic labeling of
places using information extracted from sensor data.
Robotics and Autonomous Systems, 55(5):391–402.
Myung, H., Jeon, H.-m., Jeong, W.-Y., and Bang, S.-W.
(2009). Virtual door-based coverage path planning for
mobile robot. In FIRA RoboWorld Congress, pages
197–207. Springer.
Ryu, H. (2020). Hierarchical path-planning for mobile
robots using a skeletonization-informed rapidly ex-
ploring random tree. Applied Sciences, 10(21):7846.
Saha, P. K., Borgefors, G., and di Baja, G. S. (2016). A
survey on skeletonization algorithms and their appli-
cations. Pattern recognition letters, 76:3–12.
Thrun, S. (1998). Learning metric-topological maps for in-
door mobile robot navigation. Artificial Intelligence,
99(1):21–71.
Wurm, K. M., Stachniss, C., and Burgard, W. (2008). Coor-
dinated multi-robot exploration using a segmentation
of the environment. In 2008 IEEE/RSJ International
Conference on Intelligent Robots and Systems, pages
1160–1165. IEEE.
Zuo, X., Yang, F., Liang, Y., Gang, Z., Su, F., Zhu, H., and
Li, L. (2020). An improved autonomous exploration
framework for indoor mobile robotics using reduced
approximated generalized voronoi graphs. ISPRS An-
nals of the Photogrammetry, Remote Sensing and Spa-
tial Information Sciences, 1:351–359.
ICINCO 2022 - 19th International Conference on Informatics in Control, Automation and Robotics
402