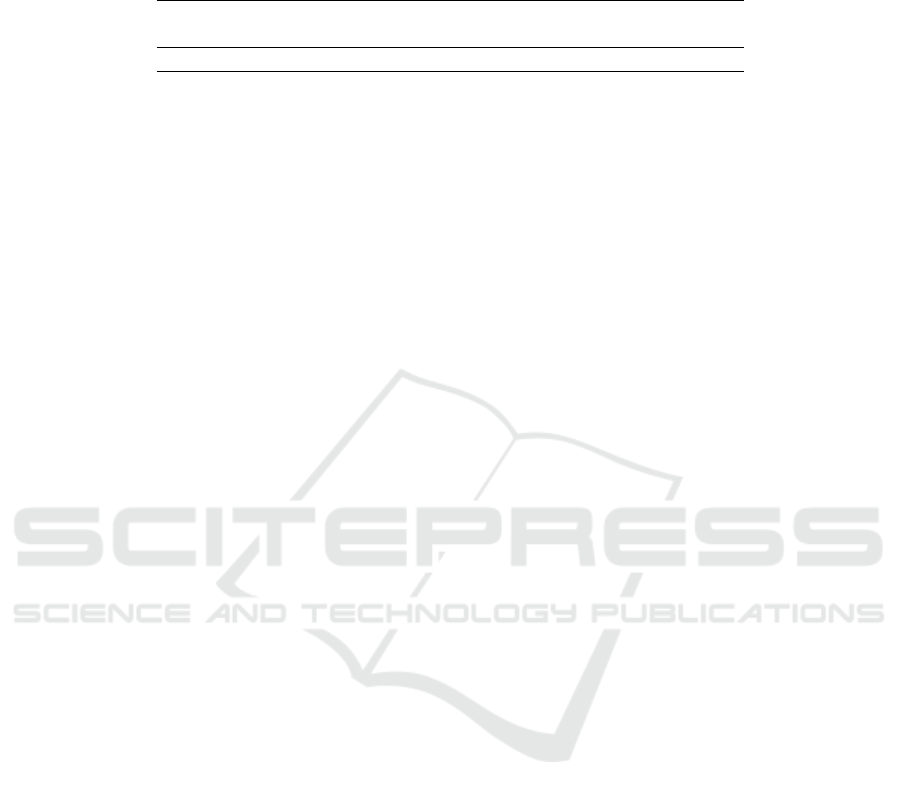
Table 2: Experimental results of CNN with New Augmentation are in terms of the means of the achieved f1 measures during
10-fold Cross-Validation.
EMOVO SAVEE EMO-DB average
(Papakostas et al., 2017) 0.57 0.60 0.67 0.61
proposed 0.70 0.51 0.72 0.64
difference +22.8% -15.0% +7.5% +4.9%
Our future goals are focused on various directions.
Firstly, we would like to increase the robustness of the
proposed method in the given datasets, by further op-
timizing the learning process of the CNN. We also be-
lieve that speaker-dependent and speaker-independent
experimental setups will lead to further improvement
of results as recent research has shown. Two such
examples are (Huang et al., 2014) and (Zhao et al.,
2019). Within a speaker-dependent setup, samples
from multiple speakers are used for training; testing
takes place on different samples which belong to the
same set of speakers. Moreover, within a speaker-
independent setup, samples from multiple speakers
are used for training and testing takes place on sam-
ples that belong to a different set of speakers. In con-
clusion, another future goal could be to experiment
with models targeting at language or cultural infor-
mation of the speech or with models that use transfer
learning, which may provide another possible solu-
tion to language independence issues.
ACKNOWLEDGEMENTS
This research has been co-financed by the European
Union and Greek national funds through the Oper-
ational Program Competitiveness, Entrepreneurship
and Innovation, under the call RESEARCH – CRE-
ATE – INNOVATE (project code: 1EDK-02070).
REFERENCES
Akc¸ay, M. B. and O
˘
guz, K. (2020). Speech emotion recog-
nition: Emotional models, databases, features, pre-
processing methods, supporting modalities, and clas-
sifiers. Speech Communication, 116:56–76.
Atmaja, B. T., Shirai, K., and Akagi, M. (2019). Speech
emotion recognition using speech feature and word
embedding. In 2019 Asia-Pacific Signal and Informa-
tion Processing Association Annual Summit and Con-
ference (APSIPA ASC), pages 519–523. IEEE.
Bhaykar, M., Yadav, J., and Rao, K. S. (2013). Speaker
dependent, speaker independent and cross language
emotion recognition from speech using gmm and
hmm. In 2013 National conference on communica-
tions (NCC), pages 1–5. IEEE.
Bitouk, D., Verma, R., and Nenkova, A. (2010). Class-
level spectral features for emotion recognition. Speech
communication, 52(7-8):613–625.
Burkhardt, F., Paeschke, A., Rolfes, M., Sendlmeier, W. F.,
Weiss, B., et al. (2005). A database of german emo-
tional speech. In Interspeech, volume 5, pages 1517–
1520.
Busso, C., Bulut, M., Lee, C.-C., Kazemzadeh, A., Mower,
E., Kim, S., Chang, J. N., Lee, S., and Narayanan,
S. S. (2008). Iemocap: Interactive emotional dyadic
motion capture database. Language resources and
evaluation, 42(4):335–359.
Chenchah, F. and Lachiri, Z. (2016). Speech emotion recog-
nition in noisy environment. In 2016 2nd Interna-
tional Conference on Advanced Technologies for Sig-
nal and Image Processing (ATSIP), pages 788–792.
IEEE.
Chollet, F. et al. (2018). Keras: The python deep learning
library. Astrophysics source code library, pages ascl–
1806.
Costantini, G., Iaderola, I., Paoloni, A., and Todisco,
M. (2014). Emovo corpus: an italian emotional
speech database. In International Conference on Lan-
guage Resources and Evaluation (LREC 2014), pages
3501–3504. European Language Resources Associa-
tion (ELRA).
Cowie, R. and Cornelius, R. R. (2003). Describing the emo-
tional states that are expressed in speech. Speech com-
munication, 40(1-2):5–32.
Cowie, R., Douglas-Cowie, E., Tsapatsoulis, N., Votsis,
G., Kollias, S., Fellenz, W., and Taylor, J. G. (2001).
Emotion recognition in human-computer interaction.
IEEE Signal processing magazine, 18(1):32–80.
Darwin, C. (2015). The expression of the emotions in man
and animals. University of Chicago press.
Ekman, P. and Oster, H. (1979). Facial expressions of emo-
tion. Annual review of psychology, 30(1):527–554.
Frantzidis, C. A., Lithari, C. D., Vivas, A. B., Papadelis,
C. L., Pappas, C., and Bamidis, P. D. (2008). To-
wards emotion aware computing: A study of arousal
modulation with multichannel event-related poten-
tials, delta oscillatory activity and skin conductivity
responses. In 2008 8th IEEE International Confer-
ence on BioInformatics and BioEngineering, pages 1–
6. IEEE.
Giannakopoulos, T. (2015). pyaudioanalysis: An open-
source python library for audio signal analysis. PloS
one, 10(12):e0144610.
Han, J., Ji, X., Hu, X., Guo, L., and Liu, T. (2015).
Arousal recognition using audio-visual features and
SIGMAP 2022 - 19th International Conference on Signal Processing and Multimedia Applications
68