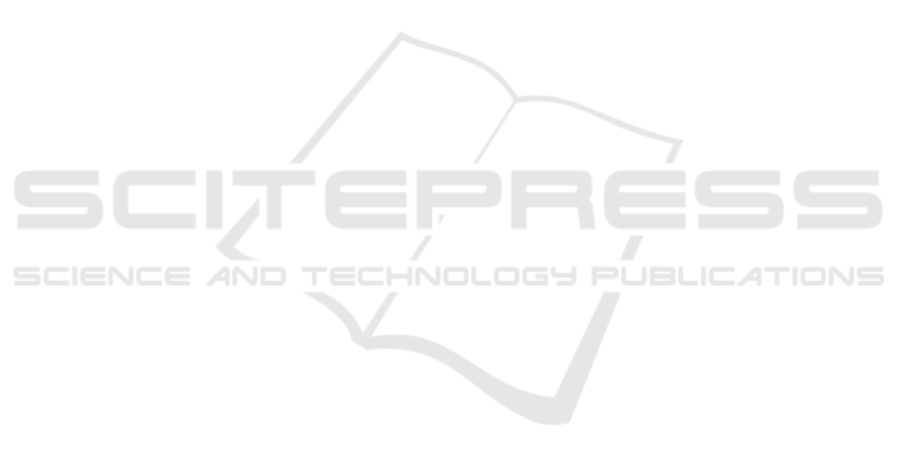
Bratus, S., Cornelius, C., Kotz, D., and Peebles, D. (2008).
Active behavioral fingerprinting of wireless devices.
In Proceedings of the first ACM conference on Wire-
less network security, pages 56–61.
Cai, D., He, X., Wu, X., and Han, J. (2008). Non-negative
matrix factorization on manifold. In 2008 eighth IEEE
international conference on data mining, pages 63–
72. IEEE.
Comon, P. (1994). Independent component analysis, a new
concept? Signal processing, 36(3):287–314.
Garc
´
ıa, S., Luengo, J., and Herrera, F. (2015). Data prepro-
cessing in data mining, volume 72. Springer.
Guerra-Urzola, R., Van Deun, K., Vera, J. C., and Sijtsma,
K. (2021). A guide for sparse pca: Model comparison
and applications. psychometrika, 86(4):893–919.
Gustavsson, V. (2019). Machine learning for a network-
based intrusion detection system: An application us-
ing zeek and the cicids2017 dataset.
Hosseinabadi, A. A. R., Sadeghilalimi, M., Shareh, M. B.,
Mouhoub, M., and Sadaoui, S. (2022). Whale
optimization-based prediction for medical diagnostic.
In Proceedings of the 14th International Conference
on Agents and Artificial Intelligence, ICAART 2022,
Volume 3, Online Streaming, February 3-5, 2022,
pages 211–217. SCITEPRESS.
Miettinen, M., Marchal, S., Hafeez, I., Asokan, N., Sadeghi,
A.-R., and Tarkoma, S. (2017). Iot sentinel: Auto-
mated device-type identification for security enforce-
ment in iot. In 2017 IEEE 37th International Con-
ference on Distributed Computing Systems (ICDCS),
pages 2177–2184. IEEE.
Msadek, N., Soua, R., and Engel, T. (2019). Iot device fin-
gerprinting: Machine learning based encrypted traffic
analysis. In 2019 IEEE wireless communications and
networking conference (WCNC), pages 1–8. IEEE.
Pedregosa, F., Varoquaux, G., Gramfort, A., Michel, V.,
Thirion, B., Grisel, O., Blondel, M., Prettenhofer, P.,
Weiss, R., Dubourg, V., et al. (2011). Scikit-learn:
Machine learning in python. the Journal of machine
Learning research, 12:2825–2830.
Perez, F. and Granger, B. E. (2015). Project jupyter: Com-
putational narratives as the engine of collaborative
data science. Retrieved September, 11(207):108.
Rao, K. R. and Yip, P. C. (2018). The transform and data
compression handbook. CRC press.
Reddy, G. T., Reddy, M. P. K., Lakshmanna, K., Kaluri, R.,
Rajput, D. S., Srivastava, G., and Baker, T. (2020).
Analysis of dimensionality reduction techniques on
big data. IEEE Access, 8:54776–54788.
Rennie, J. D., Shih, L., Teevan, J., and Karger, D. R. (2003).
Tackling the poor assumptions of naive bayes text
classifiers. In Proceedings of the 20th international
conference on machine learning (ICML-03), pages
616–623.
Said, A. B., Mohammed, E. A., and Mouhoub, M. (2021).
An implicit learning approach for solving the nurse
scheduling problem. In Mantoro, T., Lee, M., Ayu,
M. A., Wong, K. W., and Hidayanto, A. N., editors,
Neural Information Processing - 28th International
Conference, ICONIP 2021, Sanur, Bali, Indonesia,
December 8-12, 2021, Proceedings, Part II, volume
13109 of Lecture Notes in Computer Science, pages
145–157. Springer.
Sakurada, M. and Yairi, T. (2014). Anomaly detection
using autoencoders with nonlinear dimensionality re-
duction. In Proceedings of the 2nd workshop on ma-
chine learning for sensory data analysis, pages 4–11.
Schreiber, J. B. (2021). Issues and recommendations for
exploratory factor analysis and principal component
analysis. Research in Social and Administrative Phar-
macy, 17(5):1004–1011.
Silva, V. and Tenenbaum, J. (2002). Global versus local
methods in nonlinear dimensionality reduction. Ad-
vances in neural information processing systems, 15.
Thangavelu, V., Divakaran, D. M., Sairam, R., Bhunia,
S. S., and Gurusamy, M. (2018). Deft: A distributed
iot fingerprinting technique. IEEE Internet of Things
Journal, 6(1):940–952.
Van den Broeck, J., Argeseanu Cunningham, S., Eeckels,
R., and Herbst, K. (2005). Data cleaning: detect-
ing, diagnosing, and editing data abnormalities. PLoS
medicine, 2(10):e267.
Wang, Y., Yao, H., and Zhao, S. (2016). Auto-encoder
based dimensionality reduction. Neurocomputing,
184:232–242.
Yulianto, A., Sukarno, P., and Suwastika, N. A. (2019).
Improving adaboost-based intrusion detection system
(ids) performance on cic ids 2017 dataset. In Jour-
nal of Physics: Conference Series, volume 1192, page
012018. IOP Publishing.
Zhang, S., Wang, Z., Yang, J., Bai, D., Li, F., Li, Z., Wu,
J., and Liu, X. (2021). Unsupervised iot fingerprint-
ing method via variational auto-encoder and k-means.
In ICC 2021-IEEE International Conference on Com-
munications, pages 1–6. IEEE.
Efficient IoT Device Fingerprinting Approach using Machine Learning
533