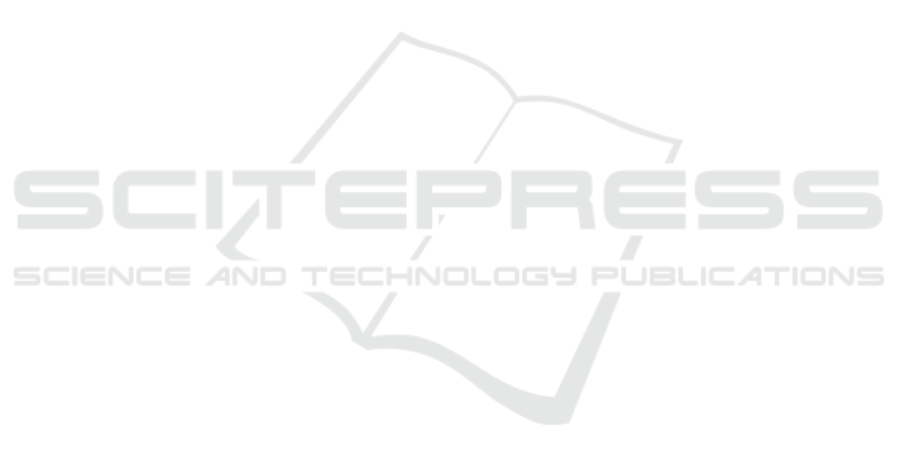
5 CONCLUSION AND FUTURE
WORK
In conclusion, this research investigates various
guidelines for compiling data into open-source data
with a focus on organizational data, data transfor-
mation, and anonymization methods. Relevant tasks
have been identified, adapted, and synthesized into a
framework to transform organizational data into open
and sharable data. To evaluate the newly created
framework, it was applied and evaluated on CERN
data. The value of the data before and after the ap-
plication of the framework has been discussed. Even
though creating a framework that encompasses all
necessary steps needed to convert sensitive organiza-
tional information into open data is a hard task, this
framework takes advantage of a diverse set of na-
tional and organizational frameworks. It provides a
generic framework that can be adapted for organiza-
tional use cases easily and provides the initial solu-
tion for the generalization of the process for compil-
ing data to open data. Based on the evaluation of the
framework, more detailed descriptions of individual
steps, improved methods for anonymization can be a
way to improve the initial framework in the future.
REFERENCES
Adar, E. (2007). User 4xxxxx9: Anonymizing query logs.
Aggarwal, C. C. and Yu, P. S. (2008). A General Survey
of Privacy-Preserving Data Mining Models and Algo-
rithms, pages 11–52. Springer US, Boston, MA.
Alexandra, S. and Brian, K. (2020). Data anonymisation:
legal, ethical, and strategic considerations.
Antony, S. and Salian, D. (2021). Usability of Open Data
Datasets, pages 410–422.
Carrara, W., Enzerink, S., Oudkerk, F., Radu, C., and van
Steenbergen, E. (2018). Open data goldbook for data
managers and data holders - europa.
Costa, P., Cordeiro, A., and OliveiraJr, E. (2021). Compar-
ing open data repositories. pages 60–69.
Data.Gov.IE (2015). Open data technical framework.
De Bie, T., De Raedt, L., Hern
´
andez-Orallo, J., Hoos, H. H.,
Smyth, P., and Williams, C. K. I. (2022). Automating
data science. Commun. ACM, 65(3):76–87.
Erg
¨
uner
¨
Ozkoc¸, E. (2021). Privacy Preserving Data Min-
ing.
European Commission (2016). Regulation (eu) 2016/679 of
the european parliament and of the council of 27 april
2016.
European Commission and Directorate-General for the In-
formation Society and Media (2002). Commercial ex-
ploitation of Europe’s public sector information : ex-
ecutive summary. Publications Office.
Grace, P., Patsakis, C., Zigomitros, A., Papageorgiou, A.,
and Pocs, M. (2016). Operando.
Jakovljevic, I., Wagner, A., and Christia, G. (2022). Cern
anonymized mattermost data.
Jakovljevic, I., Wagner, A., and G
¨
utl, C. (2020). Open
search use cases for improving information discov-
ery and information retrieval in large and highly con-
nected organizations.
Kaya, G. (2018). Good risk assessment practice in hospi-
tals. PhD thesis.
Krotova, A., Mertens, A., and Scheufen, M. (2020). Open
data and data sharing.
Ku
ˇ
cera, J., Chlapek, D., Klmek, J., and Ne
ˇ
cask
´
y, M. (2015).
Methodologies and best practices for open data publi-
cation. CEUR Workshop Proceedings, 1343:52–64.
Lee, D. (2021). Open data publication guidelines.
Lee, D., Cyganiak, R., and Decker, S. (2014). Open data
ireland: Best practice handbook.
Li, N., Li, T., and Venkatasubramanian, S. (2007).
t-closeness: Privacy beyond k-anonymity and l-
diversity. In 2007 IEEE 23rd International Confer-
ence on Data Engineering, pages 106–115.
Loenen, B., Welle Donker, F., Eijk, A., Tutic, D., and Alex-
opoulos, C. (2020). Towards an open data research
ecosystem in croatia. pages 59–70.
Lopez-Vega, H., Tell, F., and Vanhaverbeke, W. (2016).
Where and how to search? search paths in open in-
novation. Research Policy, 45:125–136.
Narayanan, A. and Shmatikov, V. (2006). How to
break anonymity of the netflix prize dataset. CoRR,
abs/cs/0610105.
Navarro-Arribas, G., Torra, V., Erola, A., and Castell
`
a-
Roca, J. (2012). User k-anonymity for privacy pre-
serving data mining of query logs. Inf. Process.
Manag., 48(3):476–487.
Ontario Human Rights Commission (2022). What is in-
volved in collecting data – six steps to success.
Open Knowledge, editor (2015). The open data handbook.
Open Knowledge.
Personal Data Protection Commission Singapore (2018).
Guide to basic data anonymisation techniques.
Pramanik, I., Lau, R., Hossain, M., Rahoman, M., Deb-
nath, S., Rashed, M. G., and Uddin, M. (2021). Pri-
vacy preserving big data analytics: A critical analysis
of state-of-the-art. Wiley Interdisciplinary Reviews:
Data Mining and Knowledge Discovery, 11.
Redman, T. C. (2022). Seizing opportunity in data quality.
Runeson, P., Olsson, T., and Lin
˚
aker, J. (2021). Open
data ecosystems — an empirical investigation into an
emerging industry collaboration concept. Journal of
Systems and Software, 182:111088.
Samarati, P. and Sweeney, L. (1998). Protecting privacy
when disclosing information: k-anonymity and its en-
forcement through generalization and suppression.
Sousa, S., Guetl, C., and Kern, R. (2021). Privacy in open
search: A review of challenges and solutions.
The Federal Assembly of the Swiss Confederation (2019).
Regulation (eu) 2016/679 of the european parliament
and of the council of 27 april 2016.
DATA 2022 - 11th International Conference on Data Science, Technology and Applications
310