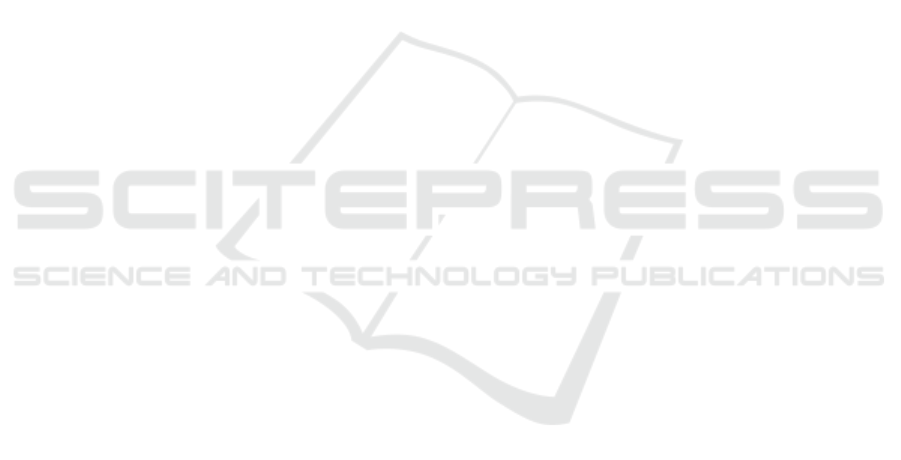
CrowdFlower (2016). https://www.figure-
eight.com/data/sentiment-analysis-emotion-text/.
Daniel, M., Neves, R. F., and Horta, N. (2017). Company
event popularity for financial markets using twitter
and sentiment analysis. Expert Systems with Appli-
cations, 71:111–124.
Deepa, D., Tamilarasi, A., et al. (2019). Sentiment anal-
ysis using feature extraction and dictionary-based ap-
proaches. In 2019 Third International conference on I-
SMAC (IoT in Social, Mobile, Analytics and Cloud)(I-
SMAC), pages 786–790. IEEE.
Deriu, J. M., Gonzenbach, M., Uzdilli, F., Lucchi, A.,
De Luca, V., and Jaggi, M. (2016). Swisscheese at
semeval-2016 task 4: Sentiment classification using
an ensemble of convolutional neural networks with
distant supervision. In Proceedings of the 10th inter-
national workshop on semantic evaluation (SemEval-
2016), pages 1124–1128.
Dimitrakakis, C. and Savu-Krohn, C. (2008). Cost-
minimising strategies for data labelling: optimal stop-
ping and active learning. In International Symposium
on Foundations of Information and Knowledge Sys-
tems, pages 96–111. Springer.
Hasan, A., Moin, S., Karim, A., and Shamshirband, S.
(2018). Machine learning-based sentiment analysis
for twitter accounts. Mathematical and Computa-
tional Applications, 23(1):11.
Hutto, C. and Gilbert, E. (2014). Vader: A parsimonious
rule-based model for sentiment analysis of social me-
dia text. In Proceedings of the international AAAI
conference on web and social media, volume 8, pages
216–225.
Lei, X., Qian, X., and Zhao, G. (2016). Rating prediction
based on social sentiment from textual reviews. IEEE
transactions on multimedia, 18(9):1910–1921.
Liao, S., Wang, J., Yu, R., Sato, K., and Cheng, Z. (2017).
Cnn for situations understanding based on sentiment
analysis of twitter data. Procedia computer science,
111:376–381.
Lindstrom, P., Delany, S. J., and Mac Namee, B. (2010).
Handling concept drift in a text data stream con-
strained by high labelling cost. In Twenty-Third In-
ternational FLAIRS Conference.
Mohammad, S., Kiritchenko, S., Sobhani, P., Zhu, X., and
Cherry, C. (2016). Semeval-2016 task 6: Detecting
stance in tweets. In Proceedings of the 10th inter-
national workshop on semantic evaluation (SemEval-
2016), pages 31–41.
Mohammad, S. M., Sobhani, P., and Kiritchenko, S. (2017).
Stance and sentiment in tweets. ACM Transactions on
Internet Technology (TOIT), 17(3):1–23.
Nielsen, F.
˚
A. (2011). A new anew: Evaluation of a
word list for sentiment analysis in microblogs. arXiv
preprint arXiv:1103.2903.
Pennington, J., Socher, R., and Manning, C. D. (2014).
Glove: Global vectors for word representation. In
Proceedings of the 2014 conference on empirical
methods in natural language processing (EMNLP),
pages 1532–1543.
Poria, S., Cambria, E., and Gelbukh, A. (2015). Deep con-
volutional neural network textual features and multi-
ple kernel learning for utterance-level multimodal sen-
timent analysis. In Proceedings of the 2015 confer-
ence on empirical methods in natural language pro-
cessing, pages 2539–2544.
Saad, E., Din, S., Jamil, R., Rustam, F., Mehmood, A.,
Ashraf, I., and Choi, G. S. (2021). Determining the ef-
ficiency of drugs under special conditions from users’
reviews on healthcare web forums. IEEE Access,
9:85721–85737.
Sheng, V. S., Provost, F., and Ipeirotis, P. G. (2008). Get
another label? improving data quality and data min-
ing using multiple, noisy labelers. In Proceedings of
the 14th ACM SIGKDD international conference on
Knowledge discovery and data mining, pages 614–
622.
Snow, R., O’Connor, B., Jurafsky, D., and Ng, A. Y. (2008).
Cheap and fast–but is it good? evaluating non-expert
annotations for natural language tasks. In Proceed-
ings of the 2008 conference on empirical methods in
natural language processing, pages 254–263.
Turney, P. D. (1994). Cost-sensitive classification: Empiri-
cal evaluation of a hybrid genetic decision tree induc-
tion algorithm. Journal of artificial intelligence re-
search, 2:369–409.
Wadera, M., Mathur, M., and Vishwakarma, D. K. (2020).
Sentiment analysis of tweets-a comparison of classi-
fiers on live stream of twitter. In 2020 4th Interna-
tional Conference on Intelligent Computing and Con-
trol Systems (ICICCS), pages 968–972. IEEE.
Whitehill, J., Wu, T.-f., Bergsma, J., Movellan, J., and Ru-
volo, P. (2009). Whose vote should count more: Op-
timal integration of labels from labelers of unknown
expertise. Advances in neural information processing
systems, 22.
Xu, G., Meng, Y., Qiu, X., Yu, Z., and Wu, X. (2019). Senti-
ment analysis of comment texts based on bilstm. IEEE
Access, 7:51522–51532.
Yoon, J. and Kim, H. (2017). Multi-channel lexicon inte-
grated cnn-bilstm models for sentiment analysis. In
Proceedings of the 29th conference on computational
linguistics and speech processing (ROCLING 2017),
pages 244–253.
A Comparison of Automatic Labelling Approaches for Sentiment Analysis
319