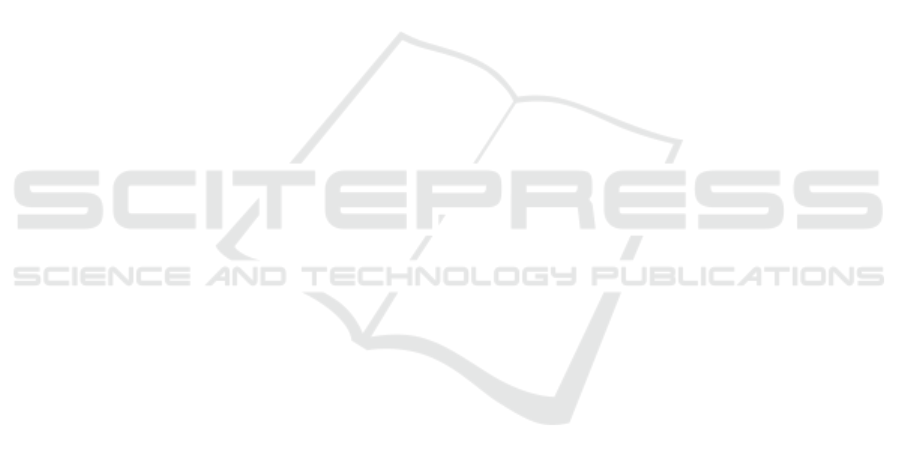
seeking to obtain a broader and more balanced repre-
sentation of the possible behaviors of the distributed
application.
Finally, the construction of intersection-based mod-
els presented a significant constraint, the flexibility
during the combination of these subsets is an oppor-
tunity to circumvent this limitation and to build less
restricted models. Another approach is to introduce
the notion of generalization in the representation of
the
n
-grams, in order to obtain a more comprehensive
(and at the same time more compact) representation of
the normal behavior of the system.
ACKNOWLEDGEMENTS
This study was financed in part by the Coordena
c¸
˜
ao de
Aperfei
c¸
oamento de Pessoal de N
´
ıvel Superior - Brazil
(CAPES) - Finance Code 001. The authors also thank
the UFPR and UTFPR Computer Science departments.
REFERENCES
Angiulli, F., Argento, L., and Furfaro, A. (2015). Exploiting
n
-gram location for intrusion detection. In Intl Conf on
Tools with Artificial Intelligence, Vietri sul Mare, Italy.
IEEE.
Arlot, S. and Celisse, A. (2010). A survey of cross-validation
procedures for model selection. Statistics Surveys, 4.
Benmoussa, H., Abou El Kalam, A., and Ait Ouahman,
A. (2015). Distributed intrusion detection system
based on anticipation and prediction approach. In Intl
Conf on Security and Cryptography, Colmar, France.
SciTePress.
Beschastnikh, I., Brun, Y., Ernst, M. D., and Krishnamurthy,
A. (2014). Inferring models of concurrent systems
from logs of their behavior with CSight. In Intl Conf
on Software Engineering, Hyderabad, India. ACM.
Borkar, A., Donode, A., and Kumari, A. (2017). A survey on
intrusion detection system (IDS) and internal intrusion
detection and protection system (IIDPS). In Intl Conf
on Inventive Computing and Informatics, Coimbatore,
India. IEEE.
Broder, A. Z., Glassman, S. C., Manasse, M. S., and Zweig,
G. (1997). Syntactic clustering of the web. Computer
Networks and ISDN Systems, 29(8).
Callegari, C., Pagano, M., Giordano, S., and Berizzi, F.
(2016). A novel histogram-based network anomaly
detection. In Intl Conf on Security and Cryptography,
Lisbon, Portugal. SciTePress.
Fu, Q., Lou, J.-G., Wang, Y., and Li, J. (2009). Execution
anomaly detection in distributed systems through un-
structured log analysis. In Intl Conf on Data Mining,
Miami, FL, USA. IEEE.
Jiang, G., Chen, H., Ungureanu, C., and Yoshihira, K. (2006).
Multiresolution abnormal trace detection using varied-
length
n
-grams and automata. IEEE Transactions on
Systems, Man, and Cybernetics, 37(1).
Jose, S., Malathi, D., Reddy, B., and Jayaseeli, D. (2018).
A survey on anomaly based host intrusion detection
system. Journal of Physics: Conference Series, 1000.
Khraisat, A., Gondal, I., Vamplew, P., and Kamruzzaman,
J. (2019). Survey of intrusion detection systems: tech-
niques, datasets and challenges. Cybersecurity, 2(1).
Lamport, L. (1978). Time, clocks, and the ordering of events
in a distributed system. Communications of the ACM,
21(7).
Lano
¨
e, D., Hurfin, M., Totel, E., and Maziero, C. (2019).
An efficient and scalable intrusion detection system
on logs of distributed applications. In Intl Conf on
ICT Systems Security and Privacy Protection, Lisbon,
Portugal. Springer.
Liao, H.-J., Lin, C.-H. R., Lin, Y.-C., and Tung, K.-Y. (2013).
Intrusion detection system: A comprehensive review.
Journal of Network and Computer Applications, 36(1).
Lorenzoli, D., Mariani, L., and Pezz
`
e, M. (2006). Inferring
state-based behavior models. In Intl Wksp on Dynamic
Systems Analysis, Shanghai, China. ACM.
Mishra, P., Pilli, E. S., Varadharajan, V., and Tupakula, U.
(2017). Intrusion detection techniques in cloud envi-
ronment: A survey. Journal of Network and Computer
Applications, 77.
Quobyte Inc (2020). XtreemFS - fault-tolerant distributed
file system. http://www.xtreemfs.org.
Stillerman, M., Marceau, C., and Stillman, M. (1999). Intru-
sion detection for distributed applications. Communi-
cations of the ACM, 42(7).
Totel, E., Hkimi, M., Hurfin, M., Leslous, M., and Labiche,
Y. (2016). Inferring a distributed application behav-
ior model for anomaly based intrusion detection. In
European Dependable Computing Conf, Gothenburg,
Sweden. IEEE.
Vartouni, A. M., Kashi, S. S., and Teshnehlab, M. (2018).
An anomaly detection method to detect web attacks
using stacked auto-encoder. In Iranian Joint Congress
on Fuzzy and Intelligent Systems, Kerman, Iran. IEEE.
Wressnegger, C., Schwenk, G., Arp, D., and Rieck, K.
(2013). A close look on
n
-grams in intrusion detec-
tion: anomaly detection vs. classification. In Wksp on
Artificial Intelligence and Security, Berlin, Germany.
ACM.
Zolotukhin, M., H
¨
am
¨
al
¨
ainen, T., and Juvonen, A. (2012).
Online anomaly detection by using n-gram model and
growing hierarchical self-organizing maps. In Intl Wire-
less Communications and Mobile Computing Conf, Li-
massol, Cyprus. IEEE.
SECRYPT 2022 - 19th International Conference on Security and Cryptography
340