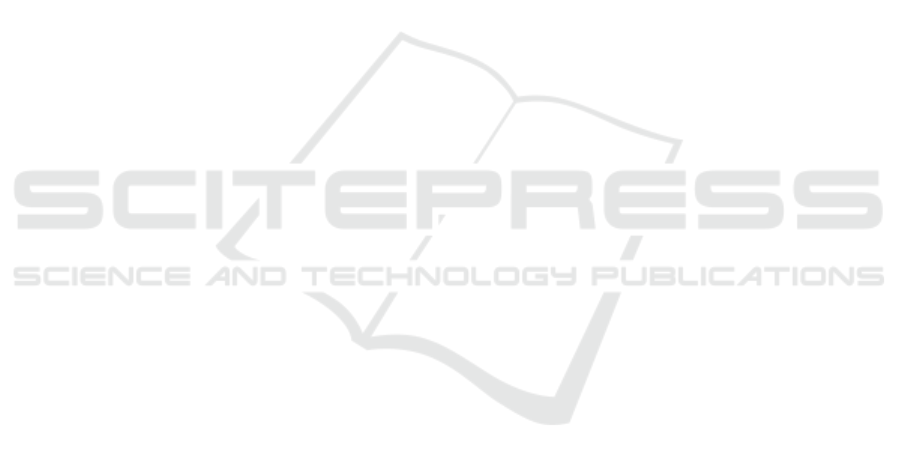
imitation and safe driving which still limits practical
applications.
REFERENCES
Bergamini, L., Ye, Y., Scheel, O., Chen, L., Hu, C.,
Del Pero, L., Osi
´
nski, B., Grimmett, H., and On-
druska, P. (2021). Simnet: Learning reactive self-
driving simulations from real-world observations. In
2021 IEEE International Conference on Robotics and
Automation (ICRA), pages 5119–5125. IEEE.
Bhattacharyya, R. P., Phillips, D. J., Liu, C., Gupta, J. K.,
Driggs-Campbell, K., and Kochenderfer, M. J. (2019).
Simulating emergent properties of human driving be-
havior using multi-agent reward augmented imita-
tion learning. In 2019 International Conference on
Robotics and Automation (ICRA), pages 789–795.
IEEE.
Brantley, K., Sun, W., and Henaff, M. (2019).
Disagreement-regularized imitation learning. In
International Conference on Learning Representa-
tions.
Cao, Z., Bıyık, E., Wang, W. Z., Raventos, A., Gaidon, A.,
Rosman, G., and Sadigh, D. (2020). Reinforcement
learning based control of imitative policies for near-
accident driving. arXiv preprint arXiv:2007.00178.
Chen, J., Li, S. E., and Tomizuka, M. (2021). Interpretable
end-to-end urban autonomous driving with latent deep
reinforcement learning. IEEE Transactions on Intelli-
gent Transportation Systems.
Codevilla, F., M
¨
uller, M., L
´
opez, A., Koltun, V., and Doso-
vitskiy, A. (2018). End-to-end driving via conditional
imitation learning. In 2018 IEEE international confer-
ence on robotics and automation (ICRA), pages 4693–
4700. IEEE.
De Haan, P., Jayaraman, D., and Levine, S. (2019). Causal
confusion in imitation learning. Advances in Neural
Information Processing Systems, 32.
Ho, J. and Ermon, S. (2016). Generative adversarial imi-
tation learning. Advances in neural information pro-
cessing systems, 29.
Kesting, A., Treiber, M., and Helbing, D. (2007). General
lane-changing model mobil for car-following models.
Transportation Research Record, 1999(1):86–94.
Knox, W. B., Allievi, A., Banzhaf, H., Schmitt, F., and
Stone, P. (2021). Reward (mis) design for autonomous
driving. arXiv preprint arXiv:2104.13906.
Kostrikov, I., Agrawal, K. K., Dwibedi, D., Levine,
S., and Tompson, J. (2018). Discriminator-actor-
critic: Addressing sample inefficiency and reward
bias in adversarial imitation learning. arXiv preprint
arXiv:1809.02925.
Kuefler, A., Morton, J., Wheeler, T., and Kochenderfer, M.
(2017). Imitating driver behavior with generative ad-
versarial networks. In 2017 IEEE Intelligent Vehicles
Symposium (IV), pages 204–211. IEEE.
Liu, R., Gao, J., Zhang, J., Meng, D., and Lin, Z. (2021).
Investigating bi-level optimization for learning and vi-
sion from a unified perspective: A survey and beyond.
IEEE Transactions on Pattern Analysis and Machine
Intelligence.
Lopez, P. A., Behrisch, M., Bieker-Walz, L., Erdmann, J.,
Fl
¨
otter
¨
od, Y.-P., Hilbrich, R., L
¨
ucken, L., Rummel,
J., Wagner, P., and Wießner, E. (2018). Microscopic
traffic simulation using sumo. In 2018 21st inter-
national conference on intelligent transportation sys-
tems (ITSC), pages 2575–2582. IEEE.
Lyu, X., Xiao, Y., Daley, B., and Amato, C. (2021).
Contrasting centralized and decentralized critics in
multi-agent reinforcement learning. arXiv preprint
arXiv:2102.04402.
Peng, X. B., Kanazawa, A., Toyer, S., Abbeel, P., and
Levine, S. (2018). Variational discriminator bottle-
neck: Improving imitation learning, inverse rl, and
gans by constraining information flow. arXiv preprint
arXiv:1810.00821.
Poggenhans, F., Pauls, J.-H., Janosovits, J., Orf, S., Nau-
mann, M., Kuhnt, F., and Mayr, M. (2018). Lanelet2:
A high-definition map framework for the future of
automated driving. In 2018 21st International Con-
ference on Intelligent Transportation Systems (ITSC),
pages 1672–1679. IEEE.
Ross, S., Gordon, G., and Bagnell, D. (2011). A reduc-
tion of imitation learning and structured prediction
to no-regret online learning. In Proceedings of the
fourteenth international conference on artificial intel-
ligence and statistics, pages 627–635. JMLR Work-
shop and Conference Proceedings.
Scheel, O., Bergamini, L., Wolczyk, M., Osi
´
nski, B., and
Ondruska, P. (2022). Urban driver: Learning to drive
from real-world demonstrations using policy gradi-
ents. In Conference on Robot Learning, pages 718–
728. PMLR.
Schulman, J., Moritz, P., Levine, S., Jordan, M., and
Abbeel, P. (2015). High-dimensional continuous con-
trol using generalized advantage estimation. arXiv
preprint arXiv:1506.02438.
Schulman, J., Wolski, F., Dhariwal, P., Radford, A., and
Klimov, O. (2017). Proximal policy optimization al-
gorithms. arXiv preprint arXiv:1707.06347.
Sharma, A. and Sharma, S. (2021). Wad: A deep rein-
forcement learning agent for urban autonomous driv-
ing. arXiv preprint arXiv:2108.12134.
Suo, S., Regalado, S., Casas, S., and Urtasun, R. (2021).
Trafficsim: Learning to simulate realistic multi-agent
behaviors. In Proceedings of the IEEE/CVF Confer-
ence on Computer Vision and Pattern Recognition,
pages 10400–10409.
Treiber, M., Hennecke, A., and Helbing, D. (2000). Con-
gested traffic states in empirical observations and mi-
croscopic simulations. Physical review E, 62(2):1805.
Wang, P., Liu, D., Chen, J., Li, H., and Chan, C.-Y. (2021).
Decision making for autonomous driving via aug-
mented adversarial inverse reinforcement learning. In
2021 IEEE International Conference on Robotics and
Automation (ICRA), pages 1036–1042. IEEE.
Zhan, W., Sun, L., Wang, D., Shi, H., Clausse, A., Nau-
mann, M., Kummerle, J., Konigshof, H., Stiller, C.,
de La Fortelle, A., et al. (2019). Interaction dataset:
An international, adversarial and cooperative motion
dataset in interactive driving scenarios with semantic
maps. arXiv preprint arXiv:1910.03088.
ICINCO 2022 - 19th International Conference on Informatics in Control, Automation and Robotics
426