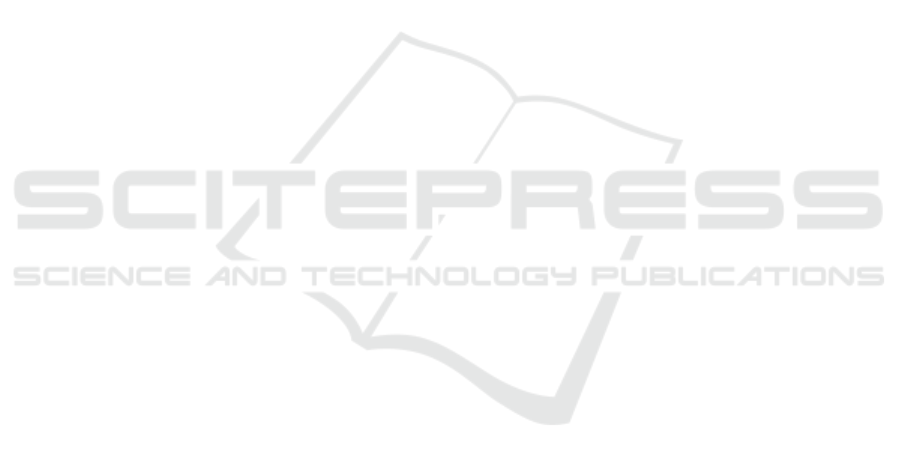
other than black markets or different data sources (e.g.,
Twitter messages) or writing styles.
In summary, our methodology provides a good
entry point to cluster profiles and documents based
on extracted information such as named entities and
to identify further correlations hidden in unstructured
data.
REFERENCES
Akbik, A., Bergmann, T., Blythe, D., Rasul, K., Schweter,
S., and Vollgraf, R. (2019). FLAIR: An Easy-to-Use
Framework for State-of-the-Art NLP. In Proceedings
of the 2019 Conference of the North American Chap-
ter of the Association for Computational Linguistics
(Demonstrations), pages 54–59, Minneapolis, Min-
nesota. Association for Computational Linguistics.
Akbik, A., Blythe, D., and Vollgraf, R. (2018). Contex-
tual String Embeddings for Sequence Labeling. In
Proceedings of the 27th International Conference on
Computational Linguistics, pages 1638–1649, Santa
Fe, New Mexico, USA. Association for Computational
Linguistics.
Baravalle, A., Lopez, M. S., and Lee, S. W. (2016). Mining
the Dark Web: Drugs and Fake Ids. In 2016 IEEE 16th
International Conference on Data Mining Workshops
(ICDMW), pages 350–356.
Benikova, D., Biemann, C., and Reznicek, M. (2014). NoSta-
D Named Entity Annotation for German: Guidelines
and Dataset. In Proceedings of the Ninth International
Conference on Language Resources and Evaluation
(LREC’14), pages 2524–2531, Reykjavik, Iceland. Eu-
ropean Language Resources Association (ELRA).
Bitkom (2018). Neun von zehn Internetnutzern
verwenden Messenger — Bitkom Main.
http://www.bitkom.org/Presse/Presseinformation/Neun-
von-zehn-Internetnutzern-verwenden-
Messenger.html.
Blankers, M., van der Gouwe, D., Stegemann, L., and Smit-
Rigter, L. (2021). Changes in Online Psychoactive
Substance Trade via Telegram during the COVID-19
Pandemic. European Addiction Research, 27(6):469–
474.
Chan, B., Schweter, S., and M
¨
oller, T. (2020). German’s
Next Language Model. arXiv:2010.10906 [cs].
Christin, N. (2013). Traveling the silk road: A measurement
analysis of a large anonymous online marketplace. Pro-
ceedings of the 22nd international conference on World
Wide Web.
Dargahi Nobari, A., Sarraf, M., Neshati, M., and Danesh-
var, F. (2020). Characteristics of viral messages on
Telegram; The world’s largest hybrid public and pri-
vate messenger. Expert Systems with Applications,
168:114303.
DataReportal and GlobalWebIndex (2021). Ger-
many: Top apps categories by reach 2020.
https://www.statista.com/statistics/1274384/top-
apps-reach-germany-by-category/.
Devlin, J., Chang, M.-W., Lee, K., and Toutanova, K. (2019).
BERT: Pre-training of Deep Bidirectional Transform-
ers for Language Understanding. arXiv:1810.04805
[cs].
Fang, W., Zhang, J., Wang, D., Chen, Z., and Li, M. (2016).
Entity Disambiguation by Knowledge and Text Jointly
Embedding. In Proceedings of The 20th SIGNLL Con-
ference on Computational Natural Language Learning,
pages 260–269, Berlin, Germany. Association for Com-
putational Linguistics.
Frisoni, G., Moro, G., and Carbonaro, A. (2020). Learning
Interpretable and Statistically Significant Knowledge
from Unlabeled Corpora of Social Text Messages: A
Novel Methodology of Descriptive Text Mining. In 9th
International Conference on Data Science, Technology
and Applications, pages 121–132.
GlobalWebIndex (2021). Coronavirus impact:
Global device usage increase by country 2020.
https://www.statista.com/statistics/1106607/device-
usage-coronavirus-worldwide-by-country/.
Gomathi, C. (2018). Social Tagging System for Community
Detecting using NLP Technique. International Jour-
nal for Research in Applied Science and Engineering
Technology, 6:1665–1671.
Grave, E., Bojanowski, P., Gupta, P., Joulin, A., and Mikolov,
T. (2018). Learning Word Vectors for 157 Languages.
arXiv:1802.06893 [cs].
Griffith, V., Xu, Y., and Ratti, C. (2017). Graph Theoretic
Properties of the Darkweb. arXiv:1704.07525 [cs].
Huang, Z., Xu, W., and Yu, K. (2015). Bidirectional LSTM-
CRF Models for Sequence Tagging. arXiv:1508.01991
[cs].
Kl
¨
oser, L., Kohl, P., Kraft, B., and Z
¨
undorf, A. (2021). Multi-
Attribute Relation Extraction (MARE) – Simplifying
the Application of Relation Extraction. Proceedings
of the 2nd International Conference on Deep Learning
Theory and Applications, pages 148–156.
Krippendorff, K. (2012). Chapter 12. Reliability. In Content
Analysis: An Introduction To Its Methodology. Sage
Publications, Inc, Los Angeles ; London, revised edi-
tion.
Krishnan, V. and Ganapathy, V. (2005). Named Entity Recog-
nition. Stanford Lecture CS229.
Lacoste, A., Luccioni, A., Schmidt, V., and Dandres, T.
(2019). Quantifying the Carbon Emissions of Machine
Learning. arXiv:1910.09700 [cs].
Landis, J. R. and Koch, G. G. (1977). The Measurement of
Observer Agreement for Categorical Data. Biometrics,
33(1):159.
Li, M., Lu, S., Zhang, L., Zhang, Y., and Zhang, B. (2021).
A Community Detection Method for Social Network
Based on Community Embedding. IEEE Transactions
on Computational Social Systems, 8(2):308–318.
Newman, M. E. J. (2006). Finding community structure in
networks using the eigenvectors of matrices. Physical
Review E, 74(3):036104.
Pedregosa, F., Varoquaux, G., Gramfort, A., Michel, V.,
Thirion, B., Grisel, O., Blondel, M., Prettenhofer, P.,
Weiss, R., Dubourg, V., Vanderplas, J., Passos, A.,
DATA 2022 - 11th International Conference on Data Science, Technology and Applications
92