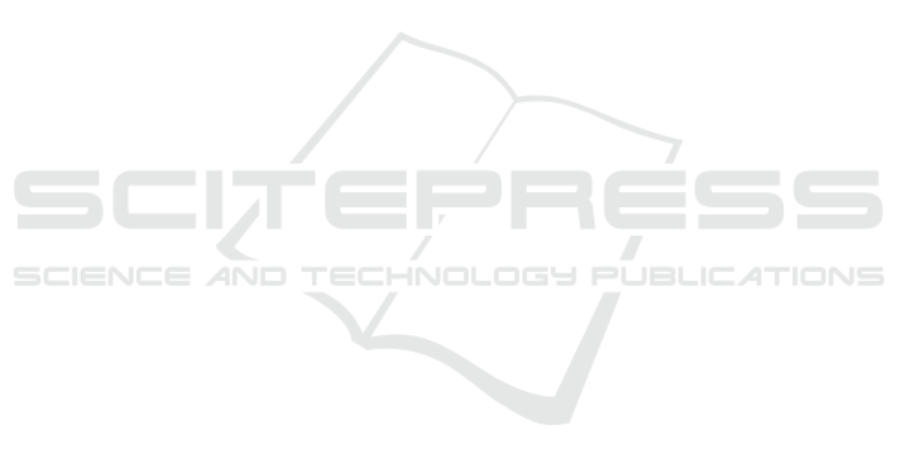
when coupled with a time-series heatmap visualiza-
tion approach. The structure of the data was investi-
gated through clustering with k means and DBSCAN,
revealing that the structure of the data in both Biopac
and E4 were more suitable for DBSCAN, which is
robust to noise.
This demonstrates the ability of clustering to be
used to discover and characterize data structure even
when labels pertaining to activity descriptions are
missing, as was the case with this data.
Clustering with different sized window lengths
had a stark impact on the structure of 81 the data in
both Biopac and E4; at a higher window length (90
and 120 seconds), the data of two participants was
flagged (the entire signal was clustered in a single
cluster, or was visually very different than the remain-
ing participants) and determined unusable.
ACKNOWLEDGEMENTS
We thank Dr. Kristal Thomassin and her students
for the use of the data from the Child Emotion
and Mental Health Lab at the University of Guelph
(https://www.childemotionlab.ca).
REFERENCES
Benedek, M. and Kaernbach, C. (2010). Decomposition of
skin conductance data by means of nonnegative de-
convolution. Psychophysiology.
Biopac (2019). EDA introductory guide. Technical Report
EDA Guide, Biopac Systems Inc., Goleta, California,
USA.
Bizzego, A., Battisti, A., Gabrieli, G., Esposito, G., and
Furlanello, C. (2019). pyphysio: A physiological sig-
nal processing library for data science approaches in
physiology. SoftwareX, 10:100287.
Boucsein, W. (2012). Electrodermal Activity. Springer Sci-
ence & Business Media.
Cecchi, S., Piersanti, A., Poli, A., and Spinsante, S. (2020).
Physical stimuli and emotions: EDA features analysis
from a wrist-worn measurement sensor. In 25th Int.
Workshop on Computer Aided Modeling and Design
(CAMAD). IEEE.
Charmandari, E., Tsigos, C., and Chrousos, G. (2005). Neu-
roendocrinology of the stress response. Annual Re-
view of Physiology, 67(1):259–284.
Chen, Y.-L., Kuan, W.-H., and Liu, C.-L. (2020). Compar-
ative study of the composition of sweat from eccrine
and apocrine sweat glands during exercise and in heat.
International Journal of Environmental Research and
Public Health, 17(10):3377.
Cho, D., Ham, J., Oh, J., Park, J., Kim, S., Lee, N.-K., and
Lee, B. (2017). Detection of stress levels from biosig-
nals measured in virtual reality environments using
a kernel-based extreme learning machine. Sensors,
17(10):2435.
Du, Q., Faber, V., and Gunzburger, M. (1999). Centroidal
voronoi tessellations: Applications and algorithms.
SIAM Review, 41(4):637–676.
Empatica (2020). E4 wristband: Real-time physiological
signals: Wearable PPG, EDA, temperature, motion
sensors. https://www.empatica.com/research/e4/.
Ester, M., Kriegel, H.-P., Sander, J., Xu, X., et al. (1996).
A density-based algorithm for discovering clusters in
large spatial databases with noise. In KDD-96 Pro-
ceedings, pages 226–231.
Greco, A., Valenza, G., Lanata, A., Scilingo, E., and Citi, L.
(2016). cvxEDA: a convex optimization approach to
electrodermal activity processing. IEEE Transactions
on Biomedical Engineering, pages 1–1.
Iveta, B., Igor, O., Michal, M., and Ingrid, T. (2015). Au-
tonomic nervous system in children with autism spec-
trum disorder. Cognitive Remediation Journal, 4.
Kirschbaum, C., Pirke, K.-M., and Hellhammer, D. H.
(1993). The ‘Trier Social Stress Test’ – a tool for in-
vestigating psychobiological stress responses in a lab-
oratory setting. Neuropsychobiology, 28(1-2):76–81.
Lowry, R. (1977). Active circuits for direct linear measure-
ment of skin resistance and conductance. Psychophys-
iology, 14(3):329–331.
MacQueen, J. (1965). Some methods for classification and
analysis of multivariate observations. In Proceed-
ings of the Fifth Berkeley Symposium on Mathematical
Statistics and Probability, volume 1, pages 281–297.
University of California Press.
Makowski, D., Pham, T., Zen, Brammer, J. C., Le, D., Hung
Pham, Lesspinasse, F., Chuan-Peng Hu, and Sch
¨
olzel,
C. (2020). Neurokit2: A python toolbox for neuro-
physiological signal processing.
Millington, G. W. M. and Graham-Brown, R. A. C. (2010).
Skin and skin disease throughout life. In Rook's Text-
book of Dermatology, pages 1–29. Wiley-Blackwell.
Posada-Quintero, H. F. and Chon, K. H. (2020). Innovations
in electrodermal activity data collection and signal
processing: A systematic review. Sensors, 20(2):479.
Sander, J., Ester, M., Kriegel, H.-P., and Xu, X. (1998).
Density-based clustering in spatial databases: The al-
gorithm gdbscan and its applications. Data Mining
and Knowledge Discovery, 2:169–194.
Schmelz, M., Schmidt, R., Bickel, A., Torebj
¨
ork, H. E., and
Handwerker, H. O. (1998). Innervation territories of
single sympathetic c fibers in human skin. Journal of
Neurophysiology, 79(4):1653–1660.
Tufte, E. R. (1991). Envisioning Information. Graphics
Press, Cheshire, Conneticut.
Watson, D., Clark, L. A., and Tellegen, A. (1988). Develop-
ment and validation of brief measures of positive and
negative affect: the PANAS scales. Journal of person-
ality and social psychology, 54(6):1063.
DATA 2022 - 11th International Conference on Data Science, Technology and Applications
352