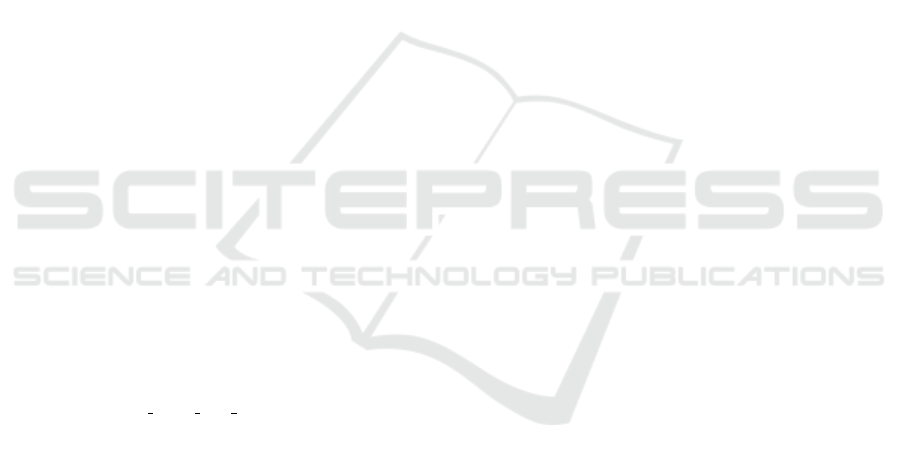
Active fault diagnosis is lacking practical exam-
ples. The design of appropriate auxiliary signals for a
broad range of FD applications is still open. Further
research in this direction has the potential to enable
safe and economical FD in real-world applications.
ACKNOWLEDGEMENTS
The authors of the Institute of Mechatronic Systems
would like to thank Lenze SE for enabling the coop-
erative project.
REFERENCES
AlShorman, O., Irfan, M., Saad, N., Zhen, D., Haider, N.,
Glowacz, A., and AlShorman, A. (2020). A Review
of Artificial Intelligence Methods for Condition Mon-
itoring and Fault Diagnosis of Rolling Element Bear-
ings for Induction Motor.
Bagnall, A., Lines, J., Bostrom, A., Large, J., and Keogh,
E. (2016). The great time series classification bake
off: a review and experimental evaluation of recent
algorithmic advances. Data Mining and Knowledge
Discovery.
Breiman, L. (2001). Random forests. Machine Learning,
45(1):5–32.
Bzinkowski, D., Ryba, T., Siemiatkowski, Z., and Rucki,
M. (2022). Real-time monitoring of the rubber belt
tension in an industrial conveyor. Reports in Mechan-
ical Engineering, 3(1):1–10.
Dau, H. A., Keogh, E., Kamgar, K., Yeh, C.-C. M., Zhu,
Y., Gharghabi, S., Ratanamahatana, C. A., Yanping,
Hu, B., Begum, N., Bagnall, A., Mueen, A., Batista,
G., and Hexagon-ML (2018). The ucr time series
classification archive. URL:https://www.cs.ucr.edu/
∼
eamonn/time series data 2018/.
Dempster, A., Schmidt, D. F., and Webb, G. I. (2021).
MINIROCKET: A Very Fast (Almost) Determinis-
tic Transform for Time Series Classification. Pro-
ceedings of the 27th ACM SIGKDD Conference on
Knowledge Discovery & Data Mining, pages 248–
257. arXiv: 2012.08791.
Fehsenfeld, M., K
¨
uhn, J., Wielitzka, M., and Ortmaier,
T. (2020). Tension Monitoring of Toothed Belt
Drives Using Interval-Based Spectral Features. IFAC-
PapersOnLine, 53(2):738–743.
Fulcher, B. D. and Jones, N. S. (2014). Highly comparative
feature-based time-series classification. IEEE Trans-
actions in Knowledge and Data Engineering, pages
3026–3037.
Gangsar, P. and Tiwari, R. (2017). Comparative investiga-
tion of vibration and current monitoring for prediction
of mechanical and electrical faults in induction mo-
tor based on multiclass-support vector machine algo-
rithms. Mechanical Systems and Signal Processing,
94:464–481.
Heirung, T. A. N. and Mesbah, A. (2019). Input design
for active fault diagnosis. Annual Reviews in Control,
47:35–50.
Hu, Y., Yan, Y., Wang, L., and Qian, X. (2016). Non-
contact vibration monitoring of power transmission
belts through electrostatic sensing. IEEE Sensors
Journal, 16(10):3541–3550.
James, G., Witten, D., Hastie, T., and Tibshirani, R. (2014).
An Introduction to Statistical Learning: With Applica-
tions in R. Springer, New York, NY, 1 edition.
Kande, M., Isaksson, A., Thottappillil, R., and Taylor, N.
(2017). Rotating electrical machine condition moni-
toring automation—a review. Machines, 5(4):24.
Kang, T., Yang, C., Park, Y., Hyun, D., Lee, S. B., and
Teska, M. (2018). Electrical monitoring of mechanical
defects in induction motor-driven v-belt–pulley speed
reduction couplings. IEEE Transactions on Industry
Applications, 54(3):2255–2264.
Khazaee, M., Banakar, A., Ghobadian, B., Mirsalim, M. A.,
Minaei, S., and Jafari, S. M. (2017). Detection of
inappropriate working conditions for the timing belt
in internal-combustion engines using vibration signals
and data mining. Proceedings of the Institution of Me-
chanical Engineers, Part D: Journal of Automobile
Engineering, 231(3):418–432.
Musselman, M. and Djurdjanovic, D. (2012). Tension mon-
itoring in a belt-driven automated material handling
system. CIRP Journal of Manufacturing Science and
Technology, 5(1):67 – 76.
Nandi, S., Toliyat, H. A., and Li, X. (2005). Condition
monitoring and fault diagnosis of electrical motors—a
review. IEEE Transactions on Energy Conversion,
20(4):719–729.
Perneder, R. and Osborne, I. (2012). Handbook Timing
Belts. Springer Berlin Heidelberg.
Picot, A., Fournier, E., R
´
egnier, J., TientcheuYamdeu, M.,
Andr
´
ejak, J., and Maussion, P. (2017). Statistic-
based method to monitor belt transmission looseness
through motor phase currents. IEEE Transactions on
Industrial Informatics, 13(3):1332–1340.
Schroeder, M. (1970). Synthesis of low-peak-factor sig-
nals and binary sequences with low autocorrelation
(corresp.). IEEE Transactions on Information Theory,
16(1):85–89.
Sharma, V. and Parey, A. (2016). A Review of Gear Fault
Diagnosis Using Various Condition Indicators. Pro-
cedia Engineering, 144:253–263.
Thoppil, N. M., Vasu, V., and Rao, C. S. P. (2021). Deep
Learning Algorithms for Machinery Health Prognos-
tics Using Time-Series Data: A Review. Journal of
Vibration Engineering & Technologies.
Toma, R. N., Prosvirin, A. E., and Kim, J.-M. (2020).
Bearing Fault Diagnosis of Induction Motors Using a
Genetic Algorithm and Machine Learning Classifiers.
Sensors, 20(7):1884.
ICINCO 2022 - 19th International Conference on Informatics in Control, Automation and Robotics
184