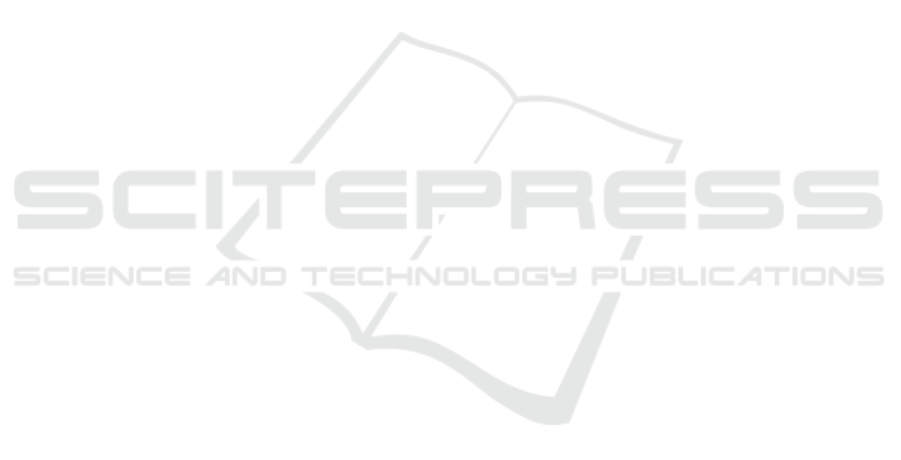
REFERENCES
Behrisch, M., Bieker-Walz, L., Erdmann, J., and Kra-
jzewicz, D. (2011). SUMO – Simulation of Urban
MObility: An overview. In Proceedings of SIMUL,
volume 2011.
Borlea, I.-D., Precup, R.-E., Borlea, A.-B., and Iercan,
D. (2021). A unified form of fuzzy c-means and k-
means algorithms and its partitional implementation.
Knowledge-Based Systems, 214:106731.
Brownlee, J. (2016). Deep Learning With Python. Machine
Learning Mastery.
Chow, A. H., Santacreu, A., Tsapakis, I., Tanasaranond, G.,
and Cheng, T. (2014). Empirical assessment of urban
traffic congestion. Journal of advanced transporta-
tion, 48(8):1000–1016.
D’Andrea, E. and Marcelloni, F. (2017). Detection of traf-
fic congestion and incidents from GPS trace analysis.
Expert Systems with Applications, 73:43–56.
Erdeli
´
c, T. and Ravli
´
c, M. (2016). SORDITO - System for
Route Optimization in Dynamic Transport Environ-
ment. PROMET - Traffic&Transportation, 28(2):193–
194.
He, Z., Qi, G., Lu, L., and Chen, Y. (2019). Network-wide
identification of turn-level intersection congestion us-
ing only low-frequency probe vehicle data. Trans-
portation Research Part C: Emerging Technologies,
108:320–339.
Kan, Z., Tang, L., Kwan, M.-P., Ren, C., Liu, D., and Li,
Q. (2019). Traffic congestion analysis at the turn level
using taxis’ GPS trajectory data. Computers, Environ-
ment and Urban Systems, 74:229–243.
Koukol, M., Zaj
´
ı
ˇ
ckov
´
a, L., Marek, L., and Tu
ˇ
cek, P. (2015).
Fuzzy logic in traffic engineering: a review on sig-
nal control. Mathematical Problems in Engineering,
2015.
Kumm, M. and Schreckenberg, M. (2019). An agent-
based approach to a temporal headway development
statistics in urban traffic using three-phase theory. In
ICAART (1), pages 238–243.
Lee, S., Wong, S., and Li, Y. (2015). Real-time estima-
tion of lane-based queue lengths at isolated signalized
junctions. Transportation Research Part C: Emerging
Technologies, 56:1–17.
Liu, H. X., Wu, X., Ma, W., and Hu, H. (2009). Real-time
queue length estimation for congested signalized in-
tersections. Transportation research part C: emerging
technologies, 17(4):412–427.
Maldonado, S., L
´
opez, J., and Vairetti, C. (2021). Time-
weighted fuzzy support vector machines for classifica-
tion in changing environments. Information Sciences,
559:97–110.
Mileti
´
c, M., Ku
ˇ
si
´
c, K., Greguri
´
c, M., and Ivanjko, E.
(2020). State complexity reduction in reinforcement
learning based adaptive traffic signal control. In 2020
International Symposium ELMAR, pages 61–66.
Papageorgiou, M., Diakaki, C., Dinopoulou, V., Kotsialos,
A., and Wang, Y. (2003). Review of road traffic con-
trol strategies. Proceedings of the IEEE, 91(12):2043–
2067.
Pozna, C. and Precup, R.-E. (2014). Applications of signa-
tures to expert systems modelling. Acta Polytechnica
Hungarica, 11(2):21–39.
Rostami Shahrbabaki, M., Safavi, A. A., Papageorgiou, M.,
and Papamichail, I. (2018). A data fusion approach
for real-time traffic state estimation in urban signal-
ized links. Transportation Research Part C: Emerging
Technologies, 92:525–548.
Seo, T., Bayen, A. M., Kusakabe, T., and Asakura, Y.
(2017). Traffic state estimation on highway: A com-
prehensive survey. Annual reviews in control, 43:128–
151.
Shuttleworth, J. (2019). SAE Standards News: J3016
automated-driving graphic update. https://www.sa
e.org/news/2019/01/sae-updates-j3016-automated-d
riving-graphic, Jan. 2019.
Ti
ˇ
sljari
´
c, L., Cari
´
c, T., Abramovi
´
c, B., and Fratrovi
´
c, T.
(2020). Traffic state estimation and classification on
citywide scale using speed transition matrices. Sus-
tainability, 12(18).
Ti
ˇ
sljari
´
c, L., Erdeli
´
c, T., and Cari
´
c, T. (2018). Analysis of
intersection queue lengths and level of service using
GPS data. In 2018 International Symposium ELMAR,
pages 43–46.
Ti
ˇ
sljari
´
c, L., Fernandes, S., Cari
´
c, T., and Gama, J. (2021).
Spatiotemporal road traffic anomaly detection: A
tensor-based approach. Applied Sciences, 11(24).
Ti
ˇ
sljari
´
c, L., Vrbani
´
c, F., Ivanjko, E., and Cari
´
c, T. (2022).
Motorway bottleneck probability estimation in con-
nected vehicles environment using speed transition
matrices. Sensors, 22.
Vuji
´
c, M. (2013). Dynamic priority systems for public
transport in urban automatic traffic control. PhD the-
sis, Faculty of Transport and Traffic Sciences, Univer-
sity of Zagreb.
Wang, Y., Yang, X., Liang, H., and Liu, Y. (2018). A review
of the self-adaptive traffic signal control system based
on future traffic environment. Journal of Advanced
Transportation, 2018:1–12.
ICINCO 2022 - 19th International Conference on Informatics in Control, Automation and Robotics
200