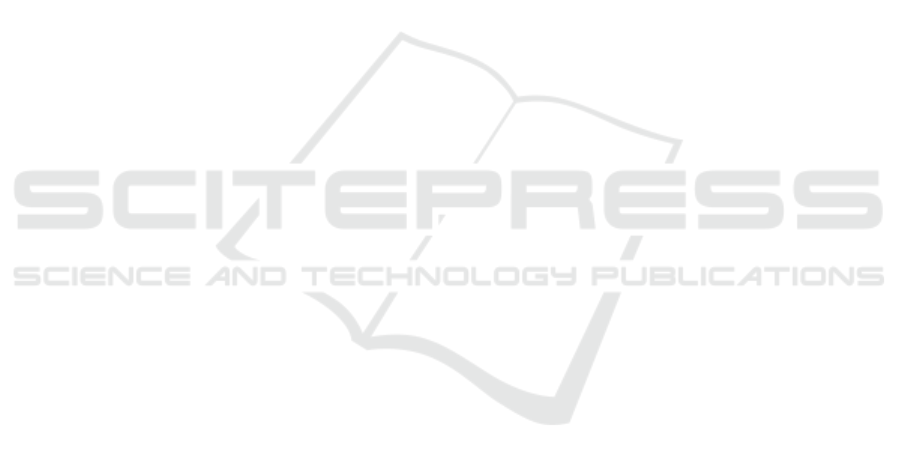
the batch estimation mode. The method was validated
by experimental tests with a real vehicle. The main
contribution is that when both improvements are ap-
plied, the calibration accuracy is improved.
Finally, the authors consider that with the develop-
ment of a more complex weighting, the bias-free cal-
ibration of every batch can be obtained. In the future
we would like to integrate a learning-based weight-
ing, however, the generation of training data is com-
plicated, since it is an open question what would be
the optimal weights that result in the true value of the
parameters.
ACKNOWLEDGEMENTS
The research was supported by the Ministry of Inno-
vation and Technology NRDI Office within the frame-
work of the Autonomous Systems National Labora-
tory Program. The paper was partially funded by the
National Research, Development and Innovation Of-
fice (NKFIH) under OTKA Grant Agreement No. K
135512. The work of M
´
at
´
e Fazekas was supported by
the
´
UNKP-21-3 New National Excellence Program of
the Ministry for Innovation and Technology from the
source of the National Research, Development and In-
novation Fund.
REFERENCES
Antonelli, G. and Chiaverini, S. (2007). Linear estimation
of the physical odometric parameters for differential-
drive mobile robots. Autonomous Robots, 23:59–68.
Antonelli, G., Chiaverini, S., and Fusco, G. (2005). A cal-
ibration method for odometry of mobile robots based
on the least-squares technique: theory and experi-
mental validation. IEEE Transactions on Robotics,
21(5):994–1004.
Baker, J. E. (1987). Reducing bias and inefficiency in the
selection algorithm. In 2nd International Conference
on Genetic Algorithms, volume 206, pages 14–21.
Bevly, D. M., Ryu, J., and Gerdes, J. C. (2006). Integrat-
ing ins sensors with gps measurements for continuous
estimation of vehicle sideslip. IEEE Transactions on
Intelligent Transportation Systems, 7(4):483–493.
Brunker, A., Wohlgemuth, T., Frey, M., and Gau-
terin, F. (2017). GNSS-shortages-resistant and self-
adaptive rear axle kinematic parameter estimator (SA-
RAKPE). In 28th IEEE Intelligent Vehicles Sympo-
sium.
Caron, F., Duflos, E., Pomorski, D., and Vanheeghe, P.
(2006). GPS/IMU data fusion using multisensor
Kalman-filtering: Introduction of contextual aspects.
Information Fusion, 7(2):221–230.
Censi, A., Franchi, A., Marchionni, L., and Oriolo, G.
(2013). Simultaneous calibration of odometry and
sensor parameters for mobile robots. IEEE Transac-
tions on Robotics, 29(2):475–492.
Fazekas, M., G
´
asp
´
ar, P., and N
´
emeth, B. (2021). Odome-
try Model Calibration for Self-Driving Vehicles with
Noise Correction. In IEEE/RSJ International Confer-
ence on Intelligent Robots and Systems (IROS).
Fazekas, M., N
´
emeth, B., G
´
asp
´
ar, P., and Sename, O.
(2020). Vehicle odometry model identification consid-
ering dynamic load transfers. In 28th Mediterranean
Conference on Control and Automation (MED), pages
19–24.
Funk, N., Alatur, N., and Deuber, R. (2017). https:
//arxiv.org/abs/1711.00548Autonomous Electric Race
Car Design. In International Electric Vehicle Sympo-
sium.
Goldberg, D. E. (1989). Genetic Algorithms in Search, Op-
timization, and Machine Learning. Addison-Wesley.
Jung, D., Seong, J., bae Moon, C., Jin, J., , and Chung, W.
(2016). Accurate calibration of systematic errors for
car-like mobile robots using experimental orientation
errors. International Journal of Precision Engineering
and Manufacturing, 17(9):1113–1119.
Ljung, L. (1987). System Identification:Theory for the User.
PTR Prentice Hall.
Ljung, L. (1994). Modeling of Dynamic Systems. PTR Pren-
tice Hall.
Ljung, L. (2010). Perspectives on system identification. An-
nual Reviews in Control, 34(1):1–12.
Martinelli, A. and Siegwart, R. (2006). Observability prop-
erties and optimal trajectories for on-line odometry
self-calibration. In IEEE Conference on Decision and
Control, pages 3065–3070.
Mathworks (2021a). Matlab global optimization toolbox:
Genetic algorithm. https://www.mathworks.com/help/
gads/genetic-algorithm.html.
Mathworks (2021b). Matlab statistics and machine learning
toolbox: k-means. https://www.mathworks.com/help/
stats/kmeans.html.
Maye, J., Sommer, H., Agamennoni, G., Siegwart, R., and
Furgale, P. (2016). Online self-calibration for robotic
systems. The International Journal of Robotics Re-
search, 35(4):357–380.
Schoukens, J. and Ljung, L. (2019). Nonlinear system iden-
tification: A user-oriented roadmap. IEEE Control
Systems Magazine, 39(6):28–99.
Seegmiller, N., Rogers-Marcovitz, F., Miller, G., and Kelly,
A. (2013). Vehicle model identification by integrated
prediction error minimization. The International Jour-
nal of Robotics Research, 32(8).
Tangirala, A. K. (2015). Principles of System Identification.
CRC.
ICINCO 2022 - 19th International Conference on Informatics in Control, Automation and Robotics
648