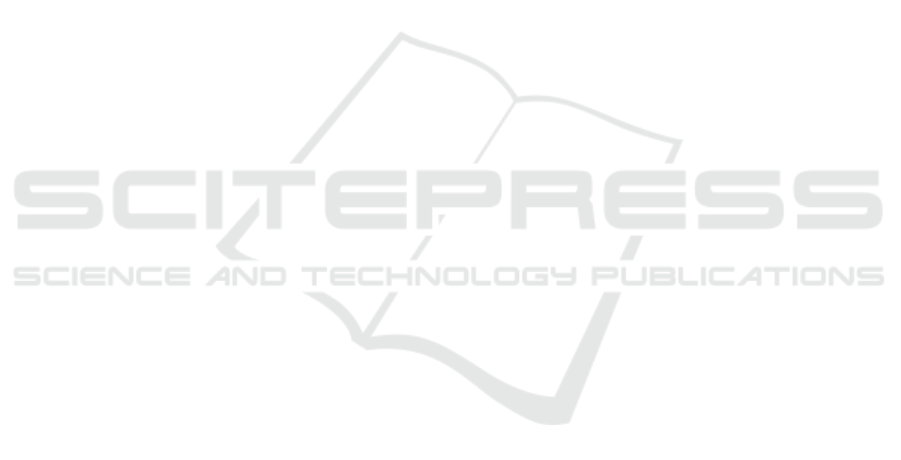
response accuracy, intelligence testing, and data la-
beling tasks. In this section, we review literature
across these different dimensions, but focus on rele-
vant data labeling studies.
Across multiple studies that collected self-
reported behavioral data, when Mechanical Turk
workers were compared to other populations, Me-
chanical Turk workers had a lower reliability in most
cases (Hamby and Taylor, 2016; Rouse, 2015), and
higher reliability for behavioral responses when it
was integrated with attention checks (Goodman et al.,
2013). In general, when checking for attentiveness
through additional survey questions, Mechanical Turk
workers tended to be more attentive (Adams et al.,
2020; Hauser and Schwarz, 2016).
To better understand population differences across
multiple dimensions, Weigold and colleagues com-
pared a traditional convenience sample (college stu-
dents) to different Mechanical Turk samples (a gen-
eral Mechanical Turk worker and college students on
Mechanical Turk)(Weigold and Weigold, 2021). They
found that the most significant differences were their
demographic characteristics, task completion time,
and task attention to detail. Mechanical Turk workers
completed tasks more quickly than college students,
but did not sacrifice detail. The authors suggested
that researchers should use Mechanical Turk college
students for data collection because they are more di-
verse and more reliable.
Kees and colleagues compare labeling reliability
of Professional Panels, Student Subject Pools, and
Mechanical Turk workers for a survey about academic
advertising of research studies (Kees et al., 2017). La-
belers were exposed to an advertisement involving a
health-related goal and completed a survey of mostly
scale questions measuring their attitude towards the
advertisements. Again, attention checks were in-
cluded and Mechanical Turk workers performed as
well or better than the other groups in terms of at-
tention and multi-tasking measures.
Researchers have also compared different crowd-
sourced worker responses to intelligence questions.
For example, Buchheit and colleagues compared stu-
dent and Mechanical Turk workers for different ac-
counting intelligence questions, e.g. profit prediction,
risk assessment, and fluid intelligence assessments
(Buchheit et al., 2019). They found that graduate stu-
dents outperformed both undergraduate and Mechani-
cal Turk workers on common accounting related intel-
ligence questions. However, on two reasonably com-
plex tasks that did not require as much accounting
knowledge, they found that Mechanical Turk work-
ers performed similarly to undergraduate accounting
students, indicating that Mechanical Turk workers are
a reasonable option when accounting expertise is not
explicitly required.
A number of studies investigate the reliability of
using Mechanical Turk for data labeling tasks. Zhou
and colleagues compare label agreement between stu-
dents for academic credit, Mechanical Turk workers,
and Master Mechanical Turk workers (Zhou et al.,
2018). The task was to place bounding boxes around
tassels. The results indicated that Mechanical Turk
workers had significantly better labeling reliability
than the for-credit students.
For summarization tasks, Mechanical Turk work-
ers produced considerably noisier, less reliable output
than expert labelers when rating the quality of a piece
of text. In other words, when expert knowledge is
necessary, the Mechanical Turk workers have much
more variability in their responses (Gillick and Liu,
2010). This finding is similar to those related to in-
telligence questions. Finally, Mechanical Turk work-
ers were also compared to citizen scientists (volunteer
scientists) (Van Horn et al., 2015). The task involved
labeling birds (bird recognition). In this study, citizen
scientists provided significantly higher quality labels
than Mechanical Turk workers, especially when an-
notating finer grain details.
This prior literature suggests that when domain or
other specialized expertise is not required, Mechan-
ical Turk workers are fairly reliable, but if the task
requires substantial background knowledge, students
will tend to perform better. While this is an impor-
tant finding, it does not provide insight into labeling
of social media data.
3 METHODOLOGY
Understanding social media posts can be difficult
given their short length, the abbreviations used, and
the informal language. Some labeling tasks that
require less interpretation may be straightforward,
while those that attempt to summarize or judge con-
tent may be more difficult. For example, suppose we
want to label the following post. Biden is an okay
president. If the task is to determine whether or not
the post is about Biden, less background knowledge
and cognitive effort are needed to answer the ques-
tion. If the task is to determine whether or not the
post shows support for Biden, more cognitive effort
is needed since interpretation of the poster’s intent is
required. Therefore, in this analysis, we consider two
dimensions, overall reliability and reliability based on
task difficulty.
For this study, we ask Mechanical Turk workers
(MTurk labelers) and university students (student la-
Students or Mechanical Turk: Who Are the More Reliable Social Media Data Labelers?
409