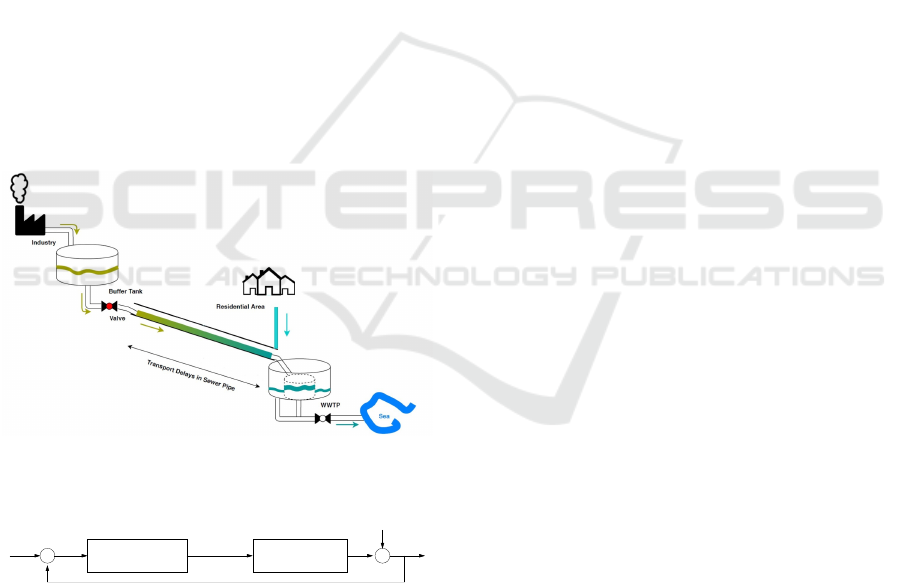
flow, as the biological processes needs time for scal-
ing. Smoothing the input flow will improve the qual-
ity of the WWTP processes.
A case study covering the north part of the area
is considered; it comprises the industrial area north
to the city, a buffer tank added close to the industrial
area, the sewer network from the buffer tank to the
WWTP and the residential areas indicated in Fig. 2.
A controller is optimising the output flow from the
tank.
3 CONTROL CONCEPT
The main goal for the control system is to reduce the
COD fluctuations in the inlet, Y , to the WWTP. It
is assumed that the only measurement is Y and the
only controllable variable is the outlet flow U from
the buffer tank. The inlet flow from industries to the
buffer tank is Q
i
. Y
re f
is the WWTP inlet flow refer-
ence. The household flows is added to one flow di-
rectly to the WWTP inlet. In this work, we look at
one buffer tank. The concept for controlling this may
easily be extended to more detention tanks. Fig. 3
shows a sketch of the simplified system.
Figure 3: Simplified sewer system with the main compo-
nents buffer tank, households, sewer pipe and WWTP.
+
ref
Q
h
U Y
Controller Main pipe
+
−
+
Y
Figure 4: Classical control concept for the problem showing
inputs, outputs and disturbances.
A classical control concept is illustrated in Fig. 4.
Q
h
is the total household flow disturbance and is seen
as a flow directly to the WWTP. The model of the
main pipe may include a transport delay; therefore, a
classic controller will result in a low bandwidth and
poor disturbance rejection (Aastrom and Haegglund,
2006; ?). The disturbance is periodic, see Fig. 1, this
periodicity is difficult to take into account in a clas-
sic control concept. It is well known from the clas-
sical control theory that cascading can improve the
performance. More flow measurements in the main
pipe will make this possible and up-stream measure-
ments of the household flow could be used as feed
forwards. Iterative learning control could be another
way to improve a classic controller. A third concept
is to use a neural network. In this work it is cho-
sen to use a Model Predictive controller (MPC); the
method is based on optimisation from prediction via
a plant model. The model can include time delays
and it is possible to incorporate knowledge of future
disturbances (household flow and potentially precipi-
tation) in the optimisation.
4 SWMM MODEL OF
FREDERICIA NORD
A realistic simulation model of the main pipes in
Fredericia Nord is developed using the EPA’s Storm
Water Management Model (SWMM) (ProtectionA-
gency, 2016). SWMM is used for simulations of wa-
ter runoff quantity and quality. It provides an environ-
ment for hydraulic and water quality simulations. The
main sewer system in the northern part of Fredericia
Fig. 2 is modelled based on information on dimen-
sions and location of pipes, channels, drains, man-
holes, storage’s and pumps. Dominating residential
and industrial areas are connected to the main pipe
Fig. 5, the household flow from these areas are con-
structed from typical data from households (Schlutter,
1999) and scaled by the number of inhabitants. In ad-
dition to the existing sewer system elements a buffer
tank are added close to the industrial area.
The purpose of the model is twofold. Test se-
quences of flow and concentrations can be applied
in the network and corresponding data sets for flow
and concentration e.g. at the inlet to the WWTP can
be used to investigate propagation rates and filtration
for flow and concentration. Additionally evaluation of
the control concept can be carried on in the simulation
environment.
5 MODELLING THE SEWER
SYSTEM
A model predictive controller requires development
of an appropriate model. The model may consists of a
description of the industrial wastewater flow and con-
centration, a tank model combined with a model of
the sewer network from the tank to the WWTP and a
ICINCO 2022 - 19th International Conference on Informatics in Control, Automation and Robotics
224