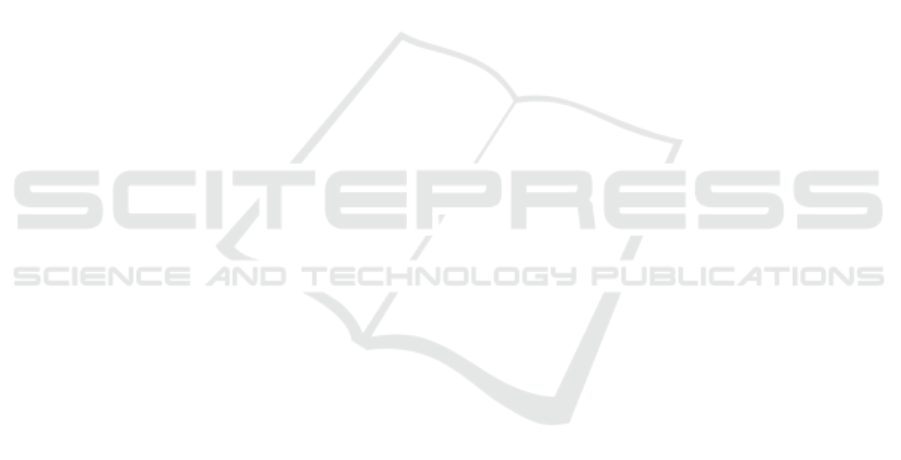
cal classification model and developing a similar clas-
sifier for multiple languages.
ACKNOWLEDGEMENTS
The authors thank MSc student Theodora Tzagkaraki
for her valuable job, and Prof. W.J. van den Heuvel
and Prof. D.A. Tamburri for providing feedback to
improve the quality of the paper.
REFERENCES
Al Nabki, W., Fidalgo, E., Alegre, E., and Fern
´
andez-
Robles, L. (2019). Torank: Identifying the most influ-
ential suspicious domains in the tor network. Expert
Systems with Applications, 123.
Al Nabki, W., Fidalgo, E., Alegre, E., and Paz, I. (2017).
Classifying illegal activities on tor network based on
web textual contents. pages 35–43.
Appendix (2022). Illicit darkweb classification via
natural-language processing: Classifying illicit con-
tent of webpages based on textual information https:
//figshare.com/s/54a17898301e2c9f7ca9.
Bhaskar, V., Linacre, R., and Machin, S. (2017). The eco-
nomic functioning of online drugs markets. Journal
of Economic Behavior & Organization.
Bird, S., Klein, E., and Loper, E. (2009). Natural language
processing with Python: analyzing text with the natu-
ral language toolkit.
Biryukov, A., Pustogarov, I., Thill, F., and Weinmann, R.
(2014). Content and popularity analysis of tor hid-
den services. In IEEE 34th International Conference
on Distributed Computing Systems Workshops (ICD-
CSW), pages 188–193.
Blei, D. M., Ng, A. Y., and Jordan, M. I. (2003). Latent
dirichlet allocation. Journal of machine Learning re-
search, 3(Jan):993–1022.
Cascavilla, G., Tamburri, D. A., and Van Den Heuvel, W.-J.
(2021). Cybercrime threat intelligence: A systematic
multi-vocal literature review. Computers & Security,
105:102258.
Celestini, A., Me, G., and Mignone, M. (2016). Tor mar-
ketplaces exploratory data analysis: The drugs case.
pages 218–229.
Choi, D., Ko, B., Kim, H., and Kim, P. (2014). Text
analysis for detecting terrorism-related articles on the
web. Journal of Network and Computer Applications,
38:16–21.
Dalins, J., Wilson, C., and Carman, M. (2018). Criminal
motivation on the dark web: A categorisation model
for law enforcement. Digital Investigation.
D
´
ecary-H
´
etu, D., Mousseau, V., and Vidal, S. (2018). Six
years later: Analyzing online black markets involved
in herbal cannabis drug dealing in the united states.
Contemporary Drug Problems, 45(4):366–381.
Engebretson, P. (2013). Chapter 2 - reconnaissance. In En-
gebretson, P., editor, The Basics of Hacking and Pen-
etration Testing (Second Edition), pages 19 – 51.
Graczyk, M. and Kinningham, K. (2015). Automatic prod-
uct categorization for anonymous marketplaces.
Hajba, G. L. (2018). Using beautiful soup. In Website
Scraping with Python, pages 41–96. Springer.
Mansfield-Devine, S. (2014). Tor under attack. Computer
Fraud & Security, 2014(8):15 – 18.
Minnaar, A. (2017). Online ‘underground’ marketplaces
for illicit drugs: The prototype case of the dark web
website ‘silk road’. page 2017.
Sabbah, T., Selamat, A., Selamat, M. H., Ibrahim, R., and
Fujita, H. (2015). Hybridized term-weighting method
for dark web classification. Neurocomputing, 173.
Spitters, M., Klaver, F., Koot, G., and Staalduinen, M.
(2015). Authorship analysis on dark marketplace fo-
rums.
Tavabi, N., Bartley, N., Abeliuk, A., Soni, S., Ferrara, E.,
and Lerman, K. (2019). Characterizing activity on the
deep and dark web. In WWW ’19.
Tsai, C.-F. (2012). Bag-of-words representation in image
annotation: A review. International Scholarly Re-
search Notices, 2012.
Uysal, A. K. and Gunal, S. (2014). The impact of prepro-
cessing on text classification. Information Processing
& Management, 50(1):104–112.
Vijayarani, S., Ilamathi, M. J., and Nithya, M. (2015). Pre-
processing techniques for text mining-an overview.
International Journal of Computer Science & Com-
munication Networks, 5(1):7–16.
Yang, L., Liu, F., Kizza, J., and Ege, R. (2009). Discovering
topics from dark websites. pages 175 – 179.
Yun-tao, Z., Ling, G., and Yong-cheng, W. (2005). An im-
proved tf-idf approach for text classification. Journal
of Zhejiang University-Science A, 6(1):49–55.
SECRYPT 2022 - 19th International Conference on Security and Cryptography
626