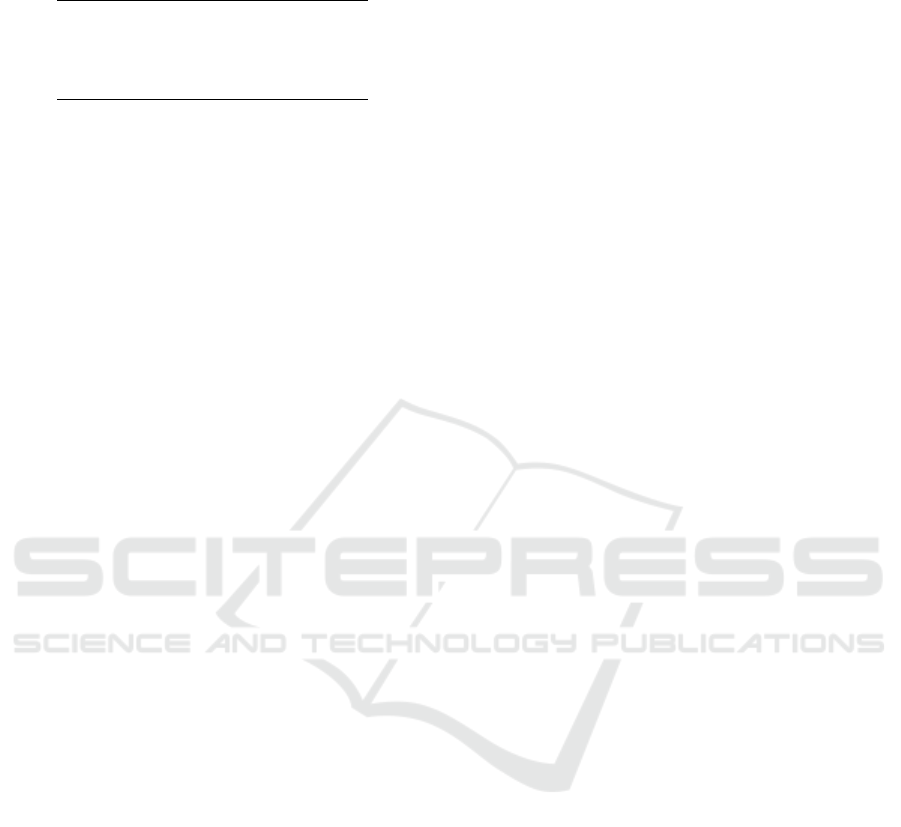
Table 10: Results for unleashed GTSRB datasets.
Prev Results Unleashed
SES 99.25 ± 0.06 99.41 ± 0.08
SER 99.32 ± 0.25 99.40 ± 0.10
SJS 99.39 ± 0.08 99.50 ± 0.09
SJR 99.41 ± 0.05 99.57 ± 0.04
5 CONCLUSION
In the proposed synthetic dataset generation pipeline,
besides traditional geometric and colour operations,
we introduced Perlin and Confetti Noise as operators
to craft synthetic samples. The usage of solid colour
backgrounds, as opposed to real backgrounds, was
also explored.
In a real scenario a traffic sign classifier must be
able to deal with a larger number of classes than those
present in the existing datasets. Adding a new class
with real data implies gathering a significant num-
ber of images where those signs are present, cropping
those images to obtain the ROI for the signs, and pro-
viding appropriate labels. On the other hand, with
synthetic data the addition of a new class amounts to
using a new template.
Based on the results we obtained, we strongly be-
lieve that creating synthetic datasets is an approach
worth pursuing. Using traditional methods, even
when no knowledge from real test sets was used, we
were able to clearly surpass synthetic dataset gener-
ation with previous approaches. While there is still
room for improvement, we were able to achieve re-
sults closer to real-world data with a standalone syn-
thetic training set on three distinct European test sets.
When considering merging and ensembles of real and
synthetic datasets, we surpassed previously reported
results with both real and synthetic data. Our cross
testing experiment also suggests that our synthetic
datasets provide a better generalisation ability com-
pared to real data.
As opposed to most other methods, we did not aim
at generating photo-realistic images. Yet, our results
clearly surpass previous attempts based on generat-
ing lifelike imagery, including those based on GANs.
Could this be interpreted as a hint that pursuing a sim-
ilarity with real imagery may not be the best option?
Further work is required to evaluate this.
Synthetic datasets have the potential to be able to
deal with different weather conditions such as fog,
snow and rain, as well as night time. This requires
increasing our pipeline to include these scenarios and
we expect to further explore the usage of synthetic
data in this direction.
ACKNOWLEDGEMENTS
This work has been supported by FCT – Fundac¸
˜
ao
para a Ci
ˆ
encia e Tecnologia within the RD Units
Project Scope: UIDB/00319/2020
REFERENCES
Araar, O., Amamra, A., Abdeldaim, A., and Vitanov, I.
(2020). Traffic sign recognition using a synthetic data
training approach. International Journal on Artificial
Intelligence Tools, 29.
Arcos-Garc
´
ıa, A., Alvarez-Garcia, J., and M. Soria-Morillo,
L. (2018). Deep neural network for traffic sign recog-
nition systems: An analysis of spatial transformers
and stochastic optimisation methods. Neural Net-
works, 99.
Cires¸an, D., Meier, U., Masci, J., and Schmidhuber, J.
(2012). Multi-column deep neural network for traffic
sign classification. Neural Networks, 32:333 – 338.
Selected Papers from IJCNN 2011.
ˇ
Segvic, S., Brki
´
c, K., Kalafati
´
c, Z., Stanisavljevi
´
c, V.,
ˇ
Sevrovi
´
c, M., Budimir, D., and Dadi
´
c, I. (2010). A
computer vision assisted geoinformation inventory for
traffic infrastructure. In 13th International IEEE Con-
ference on Intelligent Transportation Systems, pages
66–73.
Haloi, M. (2015). Traffic sign classification using deep
inception based convolutional networks. ArXiv,
abs/1511.02992.
Horn, D. and Houben, S. (2020). Fully automated traffic
sign substitution in real-world images for large-scale
data augmentation. In 2020 IEEE Intelligent Vehicles
Symposium (IV), pages 465–471.
Jain, A., Mishra, A., Shukla, A., and Tiwari, R. (2019). A
novel genetically optimized convolutional neural net-
work for traffic sign recognition: A new benchmark
on belgium and chinese traffic sign datasets. Neural
Processing Letters, pages 1–25.
Juri
ˇ
si
´
c, F., Filkovi
´
c, I., and Kalafati
´
c, Z. (2015). Multiple-
dataset traffic sign classification with onecnn. In 2015
3rd IAPR Asian Conference on Pattern Recognition
(ACPR), pages 614–618.
Liu, Y.-T., Chen, R.-C., and Dewi, C. (2021). Generate
realistic traffic sign image using deep convolutional
generative adversarial networks. In 2021 IEEE Con-
ference on Dependable and Secure Computing (DSC),
pages 1–6.
Luo, H., Kong, Q., and Wu, F. (2018). Traffic sign im-
age synthesis with generative adversarial networks.
In 2018 24th International Conference on Pattern
Recognition (ICPR), pages 2540–2545.
Mahmoud, M. A. B. and Guo, P. (2019). A novel method
for traffic sign recognition based on dcgan and mlp
with pilae algorithm. IEEE Access, 7:74602–74611.
Perlin, K. (1985). An image synthesizer. SIGGRAPH Com-
put. Graph., 19(3):287–296.
Bridging the Gap between Real and Synthetic Traffic Sign Repositories
53