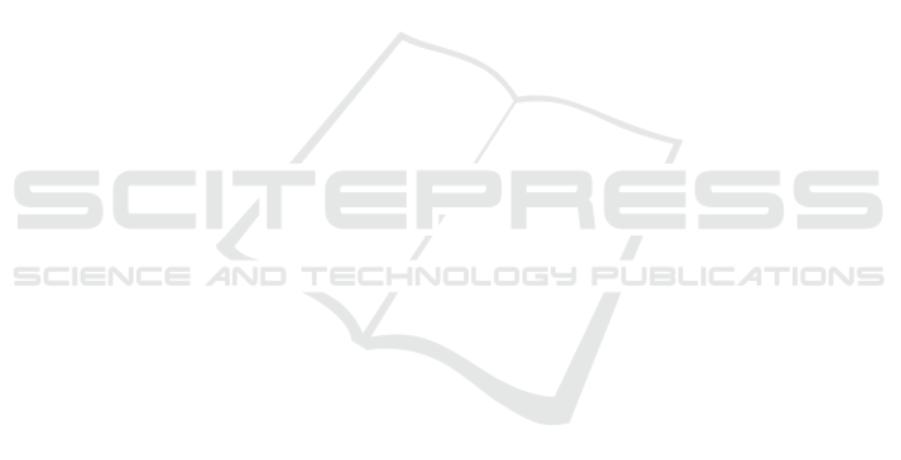
REFERENCES
Arora, M., Wiesmann, L., Chen, X., and Stachniss, C.
(2021). Mapping the Static Parts of Dynamic Scenes
from 3D LiDAR Point Clouds Exploiting Ground
Segmentation. In 2021 European Conference on Mo-
bile Robots (ECMR), pages 1–6. IEEE.
Behley, J., Garbade, M., Milioto, A., Quenzel, J., Behnke,
S., Gall, J., and Stachniss, C. (2021). Towards 3d
lidar-based semantic scene understanding of 3d point
cloud sequences: The semantickitti dataset. The Inter-
national Journal of Robotics Research, 40(8-9):959–
967.
Behley, J., Garbade, M., Milioto, A., Quenzel, J., Behnke,
S., Stachniss, C., and Gall, J. (2019). SemanticKITTI:
A dataset for semantic scene understanding of LiDAR
sequences. In Proceedings of the IEEE/CVF Interna-
tional Conference on Computer Vision, pages 9297–
9307.
Besl Paul, J. and McKay, N. D. (1992). A method for regis-
tration of 3-D shapes. IEEE Transactions on Pattern
Analysis and Machine Intelligence, 14(2):239–256.
Breiman, L. (2001). Random forests. Machine learning,
45(1):5–32.
Buitinck, L., Louppe, G., Blondel, M., Pedregosa, F.,
Mueller, A., Grisel, O., Niculae, V., Prettenhofer, P.,
Gramfort, A., Grobler, J., Layton, R., VanderPlas, J.,
Joly, A., Holt, B., and Varoquaux, G. (2013). API de-
sign for machine learning software: experiences from
the scikit-learn project. In ECML PKDD Workshop:
Languages for Data Mining and Machine Learning,
pages 108–122.
Chen, X., Vizzo, I., L
¨
abe, T., Behley, J., and Stach-
niss, C. (2021). Range image-based LiDAR local-
ization for autonomous vehicles. In 2021 IEEE In-
ternational Conference on Robotics and Automation
(ICRA), pages 5802–5808. IEEE.
Chollet, F. et al. (2015). Keras. https://keras.io.
Cortinhal, T., Tzelepi, G., and Aksoy, E. (2021). Sal-
saNext: Fast, Uncertainty-aware Semantic Segmen-
tation of LiDAR Point Clouds for Autonomous Driv-
ing. In 15th International Symposium, ISVC 2020, San
Diego, CA, USA, October 5–7, 2020, volume 12510.
Springer.
Dempster, A. P., Laird, N. M., and Rubin, D. B. (1977).
Maximum likelihood from incomplete data via the
EM algorithm. Journal of the Royal Statistical So-
ciety: Series B (Methodological), 39(1):1–22.
Fischler, M. A. and Bolles, R. C. (1981). Random sample
consensus: A paradigm for model fitting with appli-
cations to image analysis and automated cartography.
Communications of the ACM, 24(6):381–395.
Gigli, L., Kiran, B. R., Paul, T., Serna, A., Vemuri, N., Mar-
cotegui, B., and Velasco-Forero, S. (2020). Road Seg-
mentation on low resolution LIDAR point clouds for
autonomous vehicles. In XXIV International Society
for Photogrammetry and Remote Sensing Congress,
Nice, France. ISPRS 2020.
Guo, Y., Wang, H., Hu, Q., Liu, H., Liu, L., and Ben-
namoun, M. (2020). Deep learning for 3d point
clouds: A survey. IEEE transactions on pattern anal-
ysis and machine intelligence.
He, K., Zhang, X., Ren, S., and Sun, J. (2016). Deep resid-
ual learning for image recognition. In Proceedings of
the IEEE conference on computer vision and pattern
recognition, pages 770–778.
Hu, Q., Yang, B., Xie, L., Rosa, S., Guo, Y., Wang, Z.,
Trigoni, N., and Markham, A. (2020). Randla-net:
Efficient semantic segmentation of large-scale point
clouds. In Proceedings of the IEEE/CVF Conference
on Computer Vision and Pattern Recognition, pages
11108–11117.
Krizhevsky, A., Sutskever, I., and Hinton, G. E. (2012). Im-
agenet classification with deep convolutional neural
networks. Advances in neural information processing
systems, 25.
Kumari, B. and Sreevalsan-Nair, J. (2015). An interactive
visual analytic tool for semantic classification of 3D
urban LiDAR point cloud. In Proceedings of the 23rd
SIGSPATIAL International Conference on Advances
in Geographic Information Systems, pages 1–4.
Liu, Z., Liu, D., Chen, T., and Wei, C. (2013). Curb detec-
tion using 2D range data in a campus environment. In
2013 Seventh International Conference on Image and
Graphics, pages 291–296. IEEE.
Milioto, A., Vizzo, I., Behley, J., and Stachniss, C. (2019).
Rangenet++: Fast and accurate lidar semantic seg-
mentation. In 2019 IEEE/RSJ International Confer-
ence on Intelligent Robots and Systems (IROS), pages
4213–4220. IEEE.
Ouyang, Z., Dong, X., Cui, J., Niu, J., and Guizani, M.
(2021). PV-EncoNet: Fast Object Detection Based on
Colored Point Cloud. IEEE Transactions on Intelli-
gent Transportation Systems, Early Access:1–12.
Paigwar, A., Erkent,
¨
O., Sierra-Gonzalez, D., and Laugier,
C. (2020). Gndnet: Fast ground plane estimation and
point cloud segmentation for autonomous vehicles. In
2020 IEEE/RSJ International Conference on Intelli-
gent Robots and Systems (IROS), pages 2150–2156.
IEEE.
Rist, C. B., Schmidt, D., Enzweiler, M., and Gavrila, D. M.
(2020). SCSSnet: Learning Spatially-Conditioned
Scene Segmentation on LiDAR Point Clouds. In
2020 IEEE Intelligent Vehicles Symposium (IV), pages
1086–1093. IEEE.
Scott, G. J., England, M. R., Starms, W. A., Marcum, R. A.,
and Davis, C. H. (2017). Training deep convolutional
neural networks for land–cover classification of high-
resolution imagery. IEEE Geoscience and Remote
Sensing Letters, 14(4):549–553.
Shen, Z., Liang, H., Lin, L., Wang, Z., Huang, W., and Yu,
J. (2021). Fast Ground Segmentation for 3D LiDAR
Point Cloud Based on Jump-Convolution-Process. Re-
mote Sensing, 13(16):3239.
Shewchuk, J. R. (2002). Constrained Delaunay Tetrahe-
dralizations and Provably Good Boundary Recovery.
In Eleventh International Meshing Roundtable (IMR),
pages 193–204.
Stainvas, I. and Buda, Y. (2014). Performance evaluation
DeLTA 2022 - 3rd International Conference on Deep Learning Theory and Applications
66