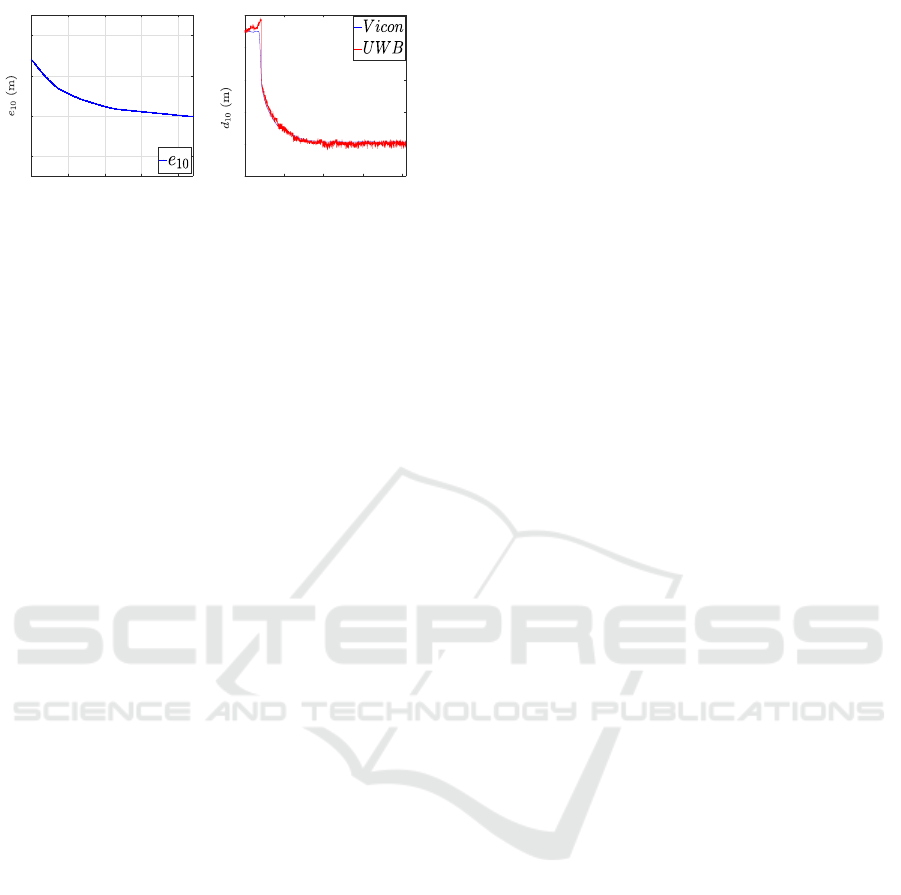
0 25 50 75 100
Time (s)
-1
0
1
2
(a)
0 100 200 300 400
Time (s)
0
0.5
1
1.5
2
2.5
(b)
Figure 12: a) The error on R
1
during a formation contrac-
tion without magnetometers, b) A Comparison of UWB
sensor data with ground truth from Vicon capture system.
focused on a three-robot setup in evaluations. When
there are more than one second-follower robots, i.e.,
when robots R
i
,i ∈ {3, . . ., N} are added to the sys-
tem, a more advanced LIDAR post-processing would
be necessary to discriminate the two leader robots for
each second-follower.
Moreover, although UWB sensors provide accu-
rate distance data in most environments, they may
generate low signal-to-noise ratio if not calibrated
properly. We suggest finding the correct bias and
noise variance values of the UWB sensors with a
proper calibration. In our experiments, we used a
basic calibration which requires to place the UWB
pair at locations with certain distances and collecting
data for a certain time. However, UWB distance mea-
surements may vary significantly with varying angu-
lar placements of the sensors and ambient factors.
We emphasize the importance of UWB calibration for
more precise evaluations.
6 CONCLUSIONS
We have investigated the practical formation control
problem for MRS using onboard sensors. We have
proposed a systematic framework including a local-
ization module and a control module. The local-
ization module consists of a bearing angle deriva-
tion based on a YOLOv3-Tiny architecture with a
monocular camera and a systematic clustering for a
2D LIDAR. The control module consists of a set of
distributed formation control laws for three types of
robots in the leader-follower hierarchy. Furthermore,
we have proposed a control method which does not
use of a magnetometer sensor which is known to
generate unreliable sensor readings in some environ-
ments. We have evaluated the framework on non-
holonomic ground robots for the formation contrac-
tion and expansion objectives in both simulations and
experiments. The high performance obtained in a se-
ries of experiments indicates the applicability of the
proposed solution. Future research may include ex-
tension of the framework for dynamic path planning
and obstacle avoidance for the whole swarm using on-
board resources. Also, LIDAR data post-processing
to discriminate the leader robots in the FOV needs to
be studied to scale up the swarm size.
ACKNOWLEDGEMENTS
This paper has been produced benefiting from
the 2232 International Fellowship for Outstanding
Researchers Program of T
¨
UB
˙
ITAK (Project No:
118C348). However, the entire responsibility of the
paper belongs to the owner of the paper. The financial
support received from T
¨
UB
˙
ITAK does not mean that
the content of the publication is approved in a scien-
tific sense by T
¨
UB
˙
ITAK.
REFERENCES
Abdelkader, M., G
¨
uler, S., Jaleel, H., and Shamma, J. S.
(2021). Aerial swarms: Recent applications and chal-
lenges. Current Robotics Reports, 2(3):309–320.
Almadhoun, R., Taha, T., Seneviratne, L., Dias, J., and Cai,
G. (2016). A survey on inspecting structures using
robotic systems. International Journal of Advanced
Robotic Systems, 13(6):1729881416663664.
Anderson, B. D., Yu, C., Fidan, B., and Hendrickx, J. M.
(2008). Rigid graph control architectures for au-
tonomous formations. IEEE Control Systems Maga-
zine, 28(6):48–63.
Choi, Y. H. and Kim, D. (2021). Distance-based forma-
tion control with goal assignment for global asymp-
totic stability of multi-robot systems. IEEE Robotics
and Automation Letters, 6(2):2020–2027.
Chung, S.-J., Paranjape, A. A., Dames, P., Shen, S., and
Kumar, V. (2018). A survey on aerial swarm robotics.
IEEE Transactions on Robotics, 34(4):837–855.
De Queiroz, M., Cai, X., and Feemster, M. (2019). Forma-
tion control of multi-agent systems: A graph rigidity
approach. John Wiley & Sons.
Fidan, B., Gazi, V., Zhai, S., Cen, N., and Karatas¸, E.
(2012). Single-view distance-estimation-based forma-
tion control of robotic swarms. IEEE Transactions on
Industrial Electronics, 60(12):5781–5791.
Guler, S., Abdelkader, M., and Shamma, J. S. (2020). Peer-
to-peer relative localization of aerial robots with ul-
trawideband sensors. IEEE Transactions on Control
Systems Technology, pages 1–16.
Kabore, K. M. and G
¨
uler, S. (2021a). Deep learning based
formation control of drones. In Deep Learning for
Unmanned Systems, pages 383–413. Springer.
Kabore, K. M. and G
¨
uler, S. (2021b). Distributed for-
mation control of drones with onboard perception.
IEEE/ASME Transactions on Mechatronics.
ICINCO 2022 - 19th International Conference on Informatics in Control, Automation and Robotics
338