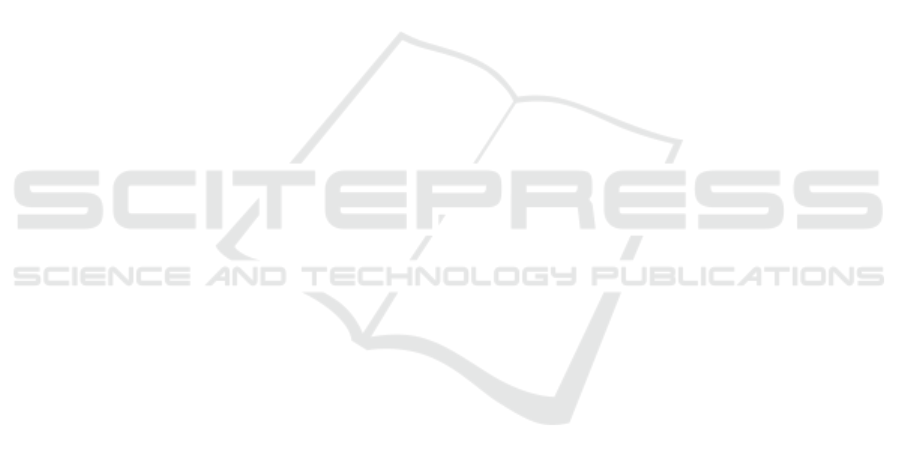
4 CONCLUSIONS
We have presented the software that forms the back-
bone of the ExpoLIS information system. We started
by listing the requirements and then proceeded with
the data model. The information system can handle a
heterogeneous mobile sensor network. We have also
presented a set of applications that use the collected
data. This has led us to select a set of air quality prop-
erties to be monitored and presented to users, either
from the general population or scientists interested
in studying and analysing air quality data. The core
database can be adapted to other geographical regions
and/or air quality measures. The data server scripts
can be easily configured to this goal. Future work has
been discussed in the previous section.
ACKNOWLEDGEMENTS
This work was supported by ExpoLIS project
(LISBOA-01-0145-FEDER-032088) funded by
FEDER, through Programa Operacional Regional
de Lisboa and national funds and FCT - Portuguese
Foundation for Science and Technology. Authors
also acknowledge the support of FCT through the
contract CEECIND/04228/2018 and the PhD grant
UI/BD/150996/2021.
REFERENCES
Becnel, T., Tingey, K., Whitaker, J., Sayahi, T., Lê, K.,
Goffin, P., Butterfield, A., Kelly, K., and Gaillardon,
P.-E. (2019). A distributed low-cost pollution mon-
itoring platform. IEEE Internet of Things Journal,
6(6):10738–10748.
Calbimonte, J.-P., Sarni, S., Eberle, J., and Aberer, K.
(2014). Xgsn: An open-source semantic sensing mid-
dleware for the web of things. In 7th International
Workshop on Semantic Sensor Networks SSN 2014, at
ISWC 2014, Riva del Garda, Italy.
Esquiagola, J., Manini, M., Aikawa, A., Yoshioka, L., and
Zuffo, M. (2018). Spiri: Low power iot solution for
monitoring indoor air quality. In Proceedings of the
3rd International Conference on Internet of Things,
Big Data and Security - IoTBDS, pages 285–290. IN-
STICC, SciTePress.
Hasenfratz, D., Saukh, O., Walser, C., Hueglin, C., Fierz,
M., Arn, T., Beutel, J., and Thiele, L. (2015). De-
riving high-resolution urban air pollution maps using
mobile sensor nodes. Pervasive and Mobile Comput-
ing, 16:268–285. Selected Papers from the Twelfth
Annual IEEE International Conference on Pervasive
Computing and Communications (PerCom 2014).
Kersting, J., Geierhos, M., Jung, H., and Kim, T. (2017).
Internet of things architecture for handling stream air
pollution data. In Proceedings of the 2nd International
Conference on Internet of Things, Big Data and Secu-
rity - IoTBDS, pages 117–124. INSTICC, SciTePress.
Kuuluvainen, H., Rönkkö, T., Järvinen, A., Saari, S., Kar-
jalainen, P., Lähde, T., Pirjola, L., Niemi, J. V.,
Hillamo, R., and Keskinen, J. (2016). Lung deposited
surface area size distributions of particulate matter
in different urban areas. Atmospheric Environment,
136:105–113.
Lin, Y.-C., Chi, W.-J., and Lin, Y.-Q. (2020). The im-
provement of spatial-temporal resolution of PM2.5
estimation based on micro-air quality sensors by us-
ing data fusion technique. Environment International,
134:105305.
Luxen, D. and Vetter, C. (2011). Real-time routing with
openstreetmap data. In Proceedings of the 19th ACM
SIGSPATIAL International Conference on Advances
in Geographic Information Systems, GIS ’11, pages
513–516, New York, NY, USA. Association for Com-
puting Machinery.
Maag, B., Hasenfratz, D., Saukh, O., Zhou, Z., Walser, C.,
Beutel, J., and Thiele, L. (2018). Ultrafine Particle
Dataset Collected by the OpenSense Zurich Mobile
Sensor Network.
Mariano, P., Almeida, S. M., and Santana, P. (2021). On the
automated learning of air pollution prediction models
from data collected by mobile sensor networks. En-
ergy Sources, Part A: Recovery, Utilization, and Envi-
ronmental Effects, 0(0):1–17.
MQTT (2019). MQTT Version 5.0. OASIS Standard.
https://docs.oasis-open.org/mqtt/mqtt/v5.0/os/mqtt-
v5.0-os.html. Latest version: https://docs.oasis-
open.org/mqtt/mqtt/v5.0/mqtt-v5.0.html.
Radanovic, G. and Faltings, B. (2015). Incentive schemes
for participatory sensing. In 14th International Con-
ference on Autonomous Agents and Multiagent Sys-
tems, Istanbul, Turkey.
Santana, P., Almeida, A., Mariano, P., Correia, C., Martins,
V., and Almeida, S. M. (2021). Air quality mapping
and visualisation: An affordable solution based on a
vehicle-mounted sensor network. Journal of Cleaner
Production, 315:128194.
Teles, B., Mariano, P., and Santana, P. (2020). Game-like
3d visualisation of air quality data. Multimodal Tech-
nologies and Interaction, 4(3).
Vital, D., Mariano, P., Santana, P., and Almeida, S. M.
(2021). A graphical tool for eliciting knowledge of
air pollution sources. In International Conference on
Graphics and Interaction – ICGI’2021.
Weissert, L., Alberti, K., Miles, E., Miskell, G., Feenstra,
B., Henshaw, G. S., Papapostolou, V., Patel, H., Poli-
dori, A., Salmond, J. A., and Williams, D. E. (2020).
Low-cost sensor networks and land-use regression:
Interpolating nitrogen dioxide concentration at high
temporal and spatial resolution in southern california.
Atmospheric Environment, 223:117287.
ICINCO 2022 - 19th International Conference on Informatics in Control, Automation and Robotics
246