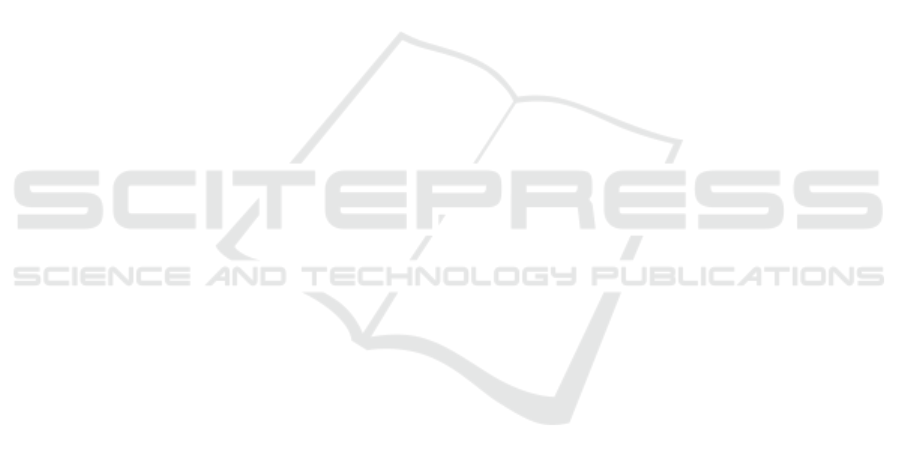
(2018). Deep personality recognition for deception
detection. In INTERSPEECH, pages 421–425.
Baik, J., Lee, K., Lee, S., Kim, Y., and Choi, J. (2016). Pre-
dicting personality traits related to consumer behavior
using sns analysis. New Review of Hypermedia and
Multimedia, 22(3):189–206.
Bakarov, A. (2018). A survey of word embeddings evalua-
tion methods. arXiv preprint arXiv:1801.09536.
Baumgartl, H., Dikici, F., Sauter, D., and Buettner, R.
(2020). Detecting antisocial personality disorder us-
ing a novel machine learning algorithm based on elec-
troencephalographic data. In PACIS, page 48.
Bernstein, D. P. and Useda, J. D. (2007). Paranoid person-
ality disorder.
Bleidorn, W. and Hopwood, C. J. (2019). Using ma-
chine learning to advance personality assessment and
theory. Personality and Social Psychology Review,
23(2):190–203.
Celli, F. and Lepri, B. (2018). Is big five better than mbti?
a personality computing challenge using twitter data.
In CLiC-it.
Cer, D., Yang, Y., Kong, S.-y., Hua, N., Limtiaco, N., John,
R. S., Constant, N., Guajardo-Cespedes, M., Yuan,
S., Tar, C., et al. (2018). Universal sentence encoder.
arXiv preprint arXiv:1803.11175.
Chawla, N. V., Bowyer, K. W., Hall, L. O., and Kegelmeyer,
W. P. (2002). Smote: synthetic minority over-
sampling technique. Journal of artificial intelligence
research, 16:321–357.
Eke, C. I., Norman, A. A., and Shuib, L. (2021). Context-
based feature technique for sarcasm identification
in benchmark datasets using deep learning and bert
model. IEEE Access, 9:48501–48518.
Ellouze, M., Mechti, S., and Belguith, L. H. (2020). Au-
tomatic profile recognition of authors on social media
based on hybrid approach. Procedia Computer Sci-
ence, 176:1111–1120.
Ellouze, M., Mechti, S., and Belguith, L. H. (2021a).
Approach based on ontology and machine learning
for identifying causes affecting personality disorder
disease on twitter. In International Conference on
Knowledge Science, Engineering and Management,
pages 659–669. Springer.
Ellouze, M., Mechti, S., Krichen, M., Belguith, L. H., et al.
(2021b). A deep learning approach for detecting the
behavior of people having personality disorders to-
wards covid-19 from twitter.
Feng, F., Yang, Y., Cer, D., Arivazhagan, N., and Wang, W.
(2020). Language-agnostic bert sentence embedding.
arXiv preprint arXiv:2007.01852.
Fernandes, E. R., de Carvalho, A. C., and Yao, X.
(2019). Ensemble of classifiers based on multiob-
jective genetic sampling for imbalanced data. IEEE
Transactions on Knowledge and Data Engineering,
32(6):1104–1115.
Gonz
´
alez-Gallardo, C. E., Montes, A., Sierra, G., N
´
unez-
Ju
´
arez, J. A., Salinas-L
´
opez, A. J., and Ek, J. (2015).
Tweets classification using corpus dependent tags,
character and pos n-grams. In CLEF (working notes).
Hall, M. and Caton, S. (2017). Am i who i say i am? unob-
trusive self-representation and personality recognition
on facebook. PloS one, 12(9):e0184417.
Haz, L., Rodr
´
ıguez-Garc
´
ıa, M.
´
A., and Fern
´
andez, A.
(2022). Detecting narcissist dark triad psychological
traits from twitter.
Ishaq, A., Sadiq, S., Umer, M., Ullah, S., Mirjalili, S., Ru-
papara, V., and Nappi, M. (2021). Improving the pre-
diction of heart failure patients’ survival using smote
and effective data mining techniques. IEEE access,
9:39707–39716.
K
˜
olves, K., V
¨
arnik, A., Schneider, B., Fritze, J., and Al-
lik, J. (2006). Recent life events and suicide: a case-
control study in tallinn and frankfurt. Social science
& medicine, 62(11):2887–2896.
Lin, H., Jia, J., Qiu, J., Zhang, Y., Shen, G., Xie, L., Tang,
J., Feng, L., and Chua, T.-S. (2017). Detecting stress
based on social interactions in social networks. IEEE
Transactions on Knowledge and Data Engineering,
29(9):1820–1833.
Mbarek, A., Jamoussi, S., Charfi, A., and Hamadou, A. B.
(2019). Suicidal profiles detection in twitter. In WE-
BIST, pages 289–296.
Muhammad, A., Hendrik, B., and Iswara, R. (2019). Ex-
pert system application for diagnosing of bipolar dis-
order with certainty factor method based on web and
android. In Journal of Physics: Conference Series,
volume 1339, page 012020. IOP Publishing.
Ombabi, A. H., Ouarda, W., and Alimi, A. M. (2020).
Deep learning cnn–lstm framework for arabic senti-
ment analysis using textual information shared in so-
cial networks. Social Network Analysis and Mining,
10(1):1–13.
Organization, W. H. (2001). The world health report 2001:
Mental health: new understanding, new hope.
Petz, G., Karpowicz, M., F
¨
urschuß, H., Auinger, A.,
St
ˇ
r
´
ıtesk
`
y, V., and Holzinger, A. (2015). Reprint of:
Computational approaches for mining user’s opinions
on the web 2.0. Information Processing & Manage-
ment, 51(4):510–519.
Pramodh, K. C. and Vijayalata, Y. (2016). Automatic per-
sonality recognition of authors using big five factor
model. In 2016 IEEE International Conference on
Advances in Computer Applications (ICACA), pages
32–37. IEEE.
Quan, Y., Zhong, X., Feng, W., Chan, J. C.-W., Li, Q., and
Xing, M. (2021). Smote-based weighted deep rotation
forest for the imbalanced hyperspectral data classifica-
tion. Remote Sensing, 13(3):464.
Reimers, N. and Gurevych, I. (2019). Sentence-bert: Sen-
tence embeddings using siamese bert-networks. arXiv
preprint arXiv:1908.10084.
Rekik, A., Jamoussi, S., and Hamadou, A. B. (2019). Vio-
lent vocabulary extraction methodology: Application
to the radicalism detection on social media. In In-
ternational Conference on Computational Collective
Intelligence, pages 97–109. Springer.
Salem, M. S., Ismail, S. S., and Aref, M. (2019). Personality
traits for egyptian twitter users dataset. In Proceedings
ICSOFT 2022 - 17th International Conference on Software Technologies
620