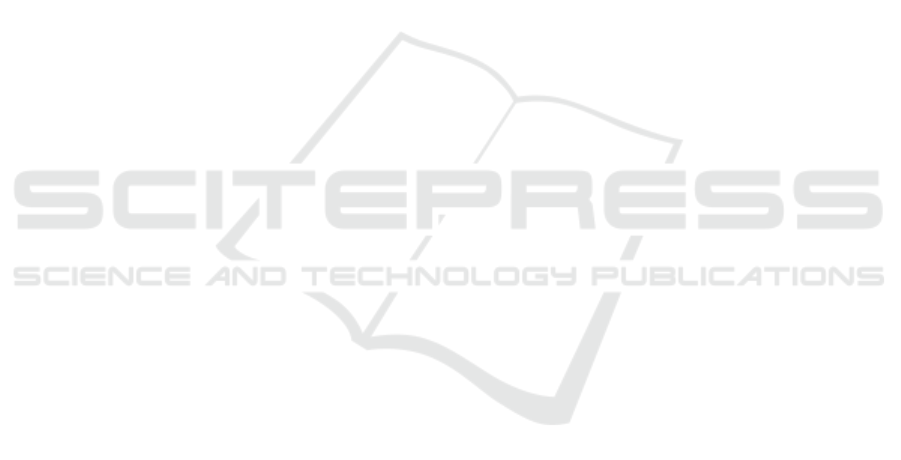
ACKNOWLEDGMENT
This research is supported by Mavinci Informatics
Inc. in Turkey, an R&D company in information and
communication technologies, security and defense ar-
eas with the capability of software development, arti-
ficial intelligence, and machine learning. This article
is a thesis article and produced from the Ph.D. thesis
(Abri, 2021).
REFERENCES
Abri, R. (2021). Modeling of the ionosphere’s disturbance
using deep learning techniques. Hacettepe University,
Graduate School of Science and Engineering.
Abri, R. and Artuner, H. (2022). Lstm-based deep learn-
ing methods for prediction of earthquakes using iono-
spheric data. Gazi University Journal of Science.
Arikan, F., Erol, C. B., and Arikan, O. (2003). Regular-
ized estimation of vertical total electron content from
global positioning system data. In Proceedings of
American Federation of Information Processing So-
cieties: 1977 National Computer Conference, number
108.
Biqiang, Z., Weixing, W., Libo, L., and Tian, M. (2007).
Morphology in the total electron content under ge-
omagnetic disturbed conditions: results from global
ionosphere maps. Annales Geophysicae. Copernicus
GmbH, 25(7):1555–1568.
Dem
ˇ
sara, U., Harris, P., Brunsdon, C., Fotheringham, A. S.,
and McLoone, S. (2013). Principal component analy-
sis on spatial data: an overview. Annals of the Associ-
ation of American Geographers, 103(1):106–128.
Khalid, S., Khalil, T., and Nasreen, S. (2014). In A survey of
feature selection and feature extraction techniques in
machine learning, 2014 science and information con-
ference, pages 372–378, London, UK. IEEE.
Kim, K. S., Choi, H. H., Moon, C. S., and Mun, C. W.
(2011). Comparison of k-nearest neighbor, quadratic
discriminant and linear discriminant analysis in clas-
sification of electromyogram signals based on the
wrist-motion directions. Current applied physics,
11(3):740–745.
Le, H., Liu, J.-Y., and Liu, L. (2011). A statistical analy-
sis of ionospheric anomalies before 736 m6. 0+ earth-
quakes during 2002–2010. Journal of Geophysical
Research: Space Physics, 116(A2).
Lin, E., Chen, Q., and Qi, X. (2020). Deep reinforcement
learning for imbalanced classification. Applied Intel-
ligence, 50(8):2488–2502.
Liu, J. Y., Chen, C. H., Chen, Y. I., Yang, W. H., Oyama,
K. I., and Kuo, K. W. (2010). A statistical study of
ionospheric earthquake precursors monitored by us-
ing equatorial ionization anomaly of gps tec in taiwan
during 2001–2007. Journal of Asian Earth Sciences,
39(1-2):76–80.
Liu, J.-Y., Chen, Y. I., Chuo, Y. J., and Chen, C.-S.
(2006). A statistical investigation of preearthquake
ionospheric anomaly. Journal of Geophysical Re-
search: Space Physics, 111(A5).
Liu, J. Y., Chuo, Y. J., Shan, S. J., Tsai, Y. B., Chen,
Y. I., Pulinets, S. A., and Yu, S. B. (2004). Pre-
earthquake ionospheric anomalies registered by con-
tinuous gps tec measurements. Annales Geophysicae,
22(5):1585–1593.
Lopez-Paz, D., Sra, S., Smola, A., Ghahramani, Z., and
Sch
¨
olkopf, B. (2014). In Randomized nonlinear com-
ponent analysis, In International conference on ma-
chine learning, pages 1359–1367. PLMR.
Makki, S., Assaghir, Z., Taher, Y., Haque, R., Hacid, M.-
S., and Zeineddine, H. (2019). In An experimental
study with imbalanced classification approaches for
credit card fraud detection, volume 7 of Access, pages
93010–93022. IEEE.
Nayir, H., Arikan, F. E. Z. A., Arikan, O., and Erol, C. B.
(2007). Total electron content estimation with reg-est.
In Space Physics, number 112.
Oikonomou, C., Haralambous, H., and Muslim, B. (2016).
Investigation of ionospheric tec precursors related to
the m7. 8 nepal and m8. 3 chile earthquakes in 2015
based on spectral and statistical analysis. Natural
Hazards, 83(1):97–116.
Pulinets, S., Ouzounov, D., Karelin, A.,
and Davidenko, D. D. (2018). Litho-
sphere–atmosphere–ionosphere–magnetosphere
coupling—a concept for pre-earthquake signals
generation.” Pre-Earthquake Processes: A Multidis-
ciplinary Approach to Earthquake Prediction Studies.
AGU Book, Wiley, New York.
Pulinets, S. A., Contreras, A. L., Bisiacchi-Giraldi, G., and
Ciraolo, L. (2003). Total electron content variations
in the ionosphere before the colima. Geof
´
ısica inter-
nacional, 44(4):369–377. earthquake of 21 January
2003.
Pundhir, D., Singh, B., Singh, O. P., Gupta, S. K., Karia,
S. P., and Pathak, K. N. (2017). Study of ionospheric
precursors using gps and gim-tec data related to earth-
quakes occurred on 16 april and 24 september. Ad-
vances in Space Research, 60(9):1978–1987. 2013 in
Pakistan region.
Rishbeth, H. and Garriott, O. K. (1969). Introduction to
ionospheric physics. volume 14, New York: Aca-
demic Press.
Shah, M. and Jin, S. (2015). Statistical characteristics
of seismo-ionospheric gps tec disturbances prior to
global mw≥ 5.0 earthquakes (1998–2014). Journal
of Geodynamics, 92:42–49.
Sharma, A. and Paliwal, K. K. (2015). Principal compo-
nent analysis on spatial data: an overview. Interna-
tional Journal of Machine Learning and Cybernetics,
6(3):443–454.
Tao, D., Cao, J., Battiston, R., Li, L., Ma, Y., Liu, W.,
Zhima, Z., Wang, L., and Dunlop, M. W. (2017).
Seismo-ionospheric anomalies in ionospheric tec and
plasma density before the 17 july 2006 m7. 7 south of
java earthquake. In Annales Geophysicae, 35(3):586–
598. Copernicus GmbH.
DATA 2022 - 11th International Conference on Data Science, Technology and Applications
166