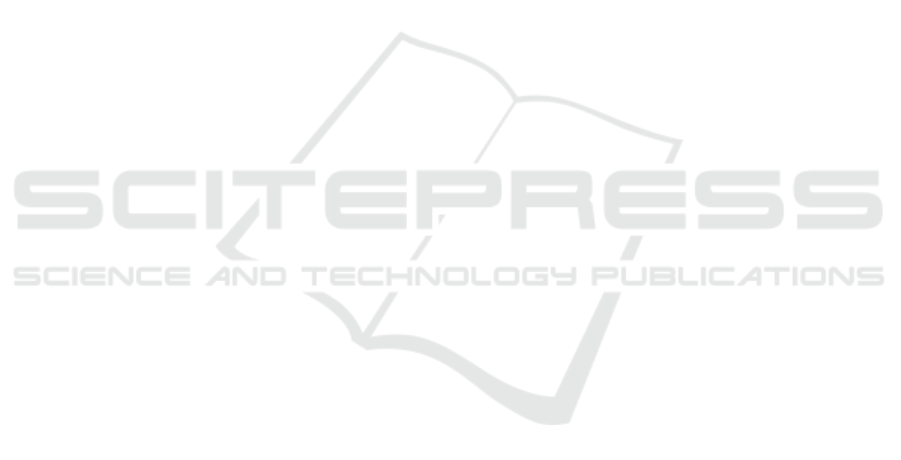
good: Why animated gifs engage us. In Proceedings
of the 2016 chi conference on human factors in com-
puting systems, pages 575–586, New York, NY, USA.
Carreira, J. and Zisserman, A. (2017). Quo vadis, action
recognition? a new model and the kinetics dataset.
In proceedings of the IEEE Conference on Computer
Vision and Pattern Recognition, pages 6299–6308.
Chen, W., Rudovic, O. O., and Picard, R. W. (2017).
Gifgif+: Collecting emotional animated gifs with
clustered multi-task learning. In 2017 Seventh Inter-
national Conference on Affective Computing and In-
telligent Interaction (ACII), pages 510–517.
Chollet, F. (2017). Xception: Deep learning with depthwise
separable convolutions. In Proceedings of the IEEE
conference on computer vision and pattern recogni-
tion, pages 1251–1258.
Deng, J., Dong, W., Socher, R., Li, L.-J., Li, K., and Fei-Fei,
L. (2009). Imagenet: A large-scale hierarchical image
database. In 2009 IEEE conference on computer vi-
sion and pattern recognition, pages 248–255.
Donahue, J., Anne Hendricks, L., Guadarrama, S.,
Rohrbach, M., Venugopalan, S., Saenko, K., and Dar-
rell, T. (2015). Long-term recurrent convolutional net-
works for visual recognition and description. In Pro-
ceedings of the IEEE conference on computer vision
and pattern recognition, pages 2625–2634.
Farha, Y. A. and Gall, J. (2019). Ms-tcn: Multi-stage tem-
poral convolutional network for action segmentation.
In Proceedings of the IEEE Conference on Computer
Vision and Pattern Recognition, pages 3575–3584.
FFmpeg (2020). Ffmpeg github page.
Heilbron, F. C., Barrios, W., Escorcia, V., and Ghanem, B.
(2017). Scc: Semantic context cascade for efficient
action detection. In 2017 IEEE Conference on Com-
puter Vision and Pattern Recognition (CVPR), pages
3175–3184.
Howard, A., Sandler, M., Chu, G., Chen, L.-C., Chen, B.,
Tan, M., Wang, W., Zhu, Y., Pang, R., Vasudevan, V.,
et al. (2019). Searching for mobilenetv3. In Proceed-
ings of the IEEE International Conference on Com-
puter Vision, pages 1314–1324.
Huang, G., Liu, Z., Van Der Maaten, L., and Weinberger,
K. Q. (2017). Densely connected convolutional net-
works. In Proceedings of the IEEE conference on
computer vision and pattern recognition, pages 4700–
4708.
Jiang, J. A., Fiesler, C., and Brubaker, J. R. (2018). ’the
perfect one’ understanding communication practices
and challenges with animated gifs. Proceedings of the
ACM on human-computer interaction, 2(CSCW):1–
20.
Jou, B., Bhattacharya, S., and Chang, S.-F. (2014). Predict-
ing viewer perceived emotions in animated gifs. In
Proceedings of the 22nd ACM international confer-
ence on Multimedia, pages 213–216, New York, NY,
USA.
Karpathy, A., Toderici, G., Shetty, S., Leung, T., Suk-
thankar, R., and Fei-Fei, L. (2014). Large-scale video
classification with convolutional neural networks. In
2014 IEEE Conference on Computer Vision and Pat-
tern Recognition, pages 1725–1732.
Liu, T., Wan, J., Dai, X., Liu, F., You, Q., and Luo, J. (2020).
Sentiment recognition for short annotated gifs using
visual-textual fusion. IEEE Transactions on Multime-
dia, 22(4):1098–1110.
Loshchilov, I. and Hutter, F. (2017). Decoupled weight de-
cay regularization.
Mujtaba, G., Lee, S., Kim, J., and Ryu, E.-S. (2021). Client-
driven animated gif generation framework using an
acoustic feature. Multimedia Tools and Applications.
Mujtaba, G. and Ryu, E.-S. (2020). Client-driven person-
alized trailer framework using thumbnail containers.
IEEE Access, 8:60417–60427.
Mujtaba, G. and Ryu, E.-S. (2021). Human character-
oriented animated gif generation framework. In 2021
Mohammad Ali Jinnah University International Con-
ference on Computing (MAJICC), pages 1–6. IEEE.
Peng, Y., Zhao, Y., and Zhang, J. (2018). Two-stream col-
laborative learning with spatial-temporal attention for
video classification. IEEE Transactions on Circuits
and Systems for Video Technology, 29(3):773–786.
Sandler, M., Howard, A., Zhu, M., Zhmoginov, A., and
Chen, L.-C. (2018). Mobilenetv2: Inverted residu-
als and linear bottlenecks. In Proceedings of the IEEE
conference on computer vision and pattern recogni-
tion, pages 4510–4520.
Shen, T., Lin, G., Shen, C., and Reid, I. (2017). Learning
multi-level region consistency with dense multi-label
networks for semantic segmentation. arXiv preprint
arXiv:1701.07122.
Shu, Y., Shi, Y., Wang, Y., Zou, Y., Yuan, Q., and Tian, Y.
(2018). Odn: Opening the deep network for open-set
action recognition. In 2018 IEEE International Con-
ference on Multimedia and Expo (ICME), pages 1–6.
Simonyan, K. and Zisserman, A. (2014). Two-stream con-
volutional networks for action recognition in videos.
Advances in neural information processing systems,
27:568–576.
Song, Y., Redi, M., Vallmitjana, J., and Jaimes, A. (2016).
To click or not to click: Automatic selection of beauti-
ful thumbnails from videos. In Proceedings of the 25th
ACM International on Conference on Information and
Knowledge Management, page 659–668, New York,
NY, USA.
Soomro, K., Zamir, A. R., and Shah, M. (2012). Ucf101:
A dataset of 101 human actions classes from videos in
the wild.
Xie, C.-W., Zhou, H.-Y., and Wu, J. (2018). Vortex pooling:
Improving context representation in semantic segmen-
tation.
Xu, Y., Bai, F., Shi, Y., Chen, Q., Gao, L., Tian, K.,
Zhou, S., and Sun, H. (2021). Gif thumbnails: At-
tract more clicks to your videos. In Proceedings of
the AAAI Conference on Artificial Intelligence, pages
3074–3082.
Yang, K., Shen, X., Qiao, P., Li, S., Li, D., and Dou, Y.
(2019). Exploring frame segmentation networks for
temporal action localization. Journal of Visual Com-
munication and Image Representation, 61:296–302.
Yuan, Y., Ma, L., and Zhu, W. (2019). Sentence specified
dynamic video thumbnail generation. In Proceedings
of the 27th ACM International Conference on Multi-
media, pages 2332–2340.
Client-driven Lightweight Method to Generate Artistic Media for Feature-length Sports Videos
111