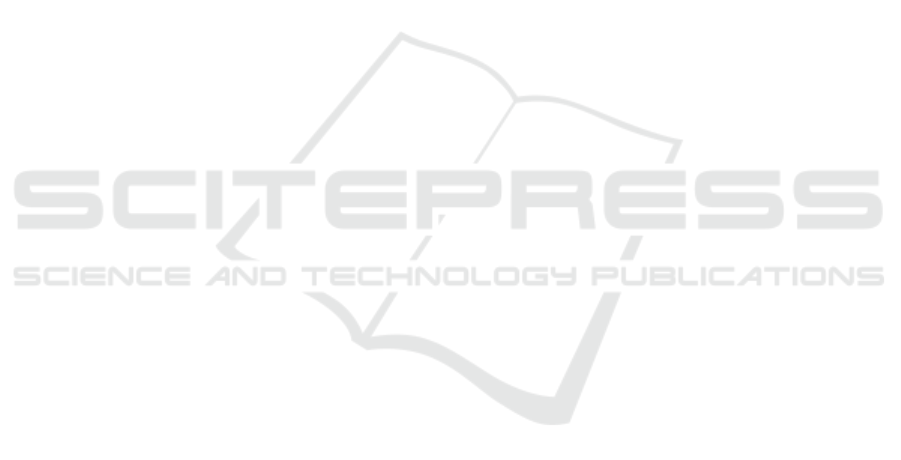
5 CONCLUSION
The present study has aimed to extend the GOATS to
multi-variate nonlinear dynamic systems, to develop a
new signal type iGOATS, to create a new space-filling
loss function MCUDSA, and to produce a compres-
sion algorithm to significantly speed up optimizations
of space-filling loss functions.
The GOATS has been successfully extended to
multi-variate nonlinear dynamic systems with a supe-
rior expectable model quality and space-filling prop-
erty.
Furthermore, a new signal type – iGOATS –
has been developed. The iGOATS combines the
good expectable model qualities of the GOATS with
the incremental feature of the OMNIPUS. Conse-
quently, the GOATS and iGOATS surpass the OM-
NIPUS, APRBS, and Multi-Sine significantly espe-
cially for short signal durations on the artificially two-
dimensional nonlinear dynamic process.
The new space-filling loss function MCUDSA for
the optimization of the GOATS slightly outperforms
the AE and FA loss functions in this investigation.
However, for greater and more complex systems the
AE might be interesting as well due to the faster eval-
uation speed and optimization.
The approach to accelerate the optimization speed
of space-filling loss functions for dynamic DoEs via
compressing the data shows that the evaluation can
be sped up between 3 −6 times according to the used
loss function including the computational effort of the
compression algorithm itself.
In future research, the GOATS and iGOATS have
to be examined for higher dimensional, higher order
and real world dynamic nonlinear systems.
REFERENCES
Audze, P. and Eglais, V. (1977). New approach for plan-
ning out of experiments. Problems of Dynamics and
Strengths, 35:104–107.
Bates, S. J., Sienz, J., and Toropov, V. V. (2004). Formula-
tion of the optimal latin hypercube design of experiments
using a permutation genetic algorithm. In Collection of
Technical Papers - AIAA/ASME/ASCE/AHS/ASC Struc-
tures, Structural Dynamics and Materials Conference,
volume 45.
Bezanson, J., Edelman, A., Karpinski, S., and Shah, V. B.
(2017). Julia: A fresh approach to numerical computing.
SIAM review, 59(1):65–98.
Bratley, P. and Fox, B. L. (1988). Algorithm 659: Im-
plementing Sobol’s Quasirandom Sequence Generator.
ACM Transactions on Mathematical Software (TOMS),
14(1):88–100.
Deb, K., Agrawal, S., Pratap, A., and Meyarivan, T. (2000).
A fast elitist non-dominated sorting genetic algorithm
for multi-objective optimization: NSGA-II. In Interna-
tional conference on parallel problem solving from na-
ture, pages 849–858.
Goldberg, D. E. and Deb, K. (1991). A Comparative Anal-
ysis of Selection Schemes Used in Genetic Algorithms.
Foundations of genetic algorithms, 1:69–93.
Heinz, T. O. and Nelles, O. (2017). Iterative Excitation Sig-
nal Design for Nonlinear Dynamic Black-Box Models.
Procedia Computer Science, pages 1054–1061.
Heinz, T. O., Schillinger, M., Hartmann, B., and Nelles, O.
(2017). Excitation signal design for nonlinear dynamic
systems with multiple inputs – A data distribution ap-
proach. In R
¨
opke, K. and G
¨
uhmann, C., editors, Inter-
national Calibration Conference - Automotive Data An-
alytics, Methods, DoE, pages 191–208. expertVerlag.
Hoagg, J. B., Lacy, S. L., Babu
ˇ
ska, V., and Bernstein, D. S.
(2006). Sequential multisine excitation signals for sys-
tem identification of large space structures. In Proceed-
ings of the American Control Conference, pages 418–
423.
Joe, S. and Kuo, F. Y. (2003). Remark on Algorithm 659:
Implementing Sobol’s quasirandom sequence generator.
ACM Transactions on Mathematical Software, 29(1):49–
57.
Lin, W. Y., Lee, W. Y., and Hong, T. P. (2003). Adapting
crossover and mutation rates in genetic algorithms. Jour-
nal of Information Science and Engineering, 19:889–
903.
Nelles, O. (2006). Axes-Oblique Partitioning Strategies for
Local Model Networks. In IEEE International Sympo-
sium on Intelligent Control, pages 2378–2383. IEEE.
Nelles, O. (2013). Nonlinear system identification: from
classical approaches to neural networks and fuzzy mod-
els. Springer Science & Business Media.
Peter, T. J. and Nelles, O. (2019). Fast and sim-
ple dataset selection for machine learning. at-
Automatisierungstechnik, 67(10):833–842.
Rackauckas, C., Ma, Y., Martensen, J., Warner, C., Zubov,
K., Supekar, R., Skinner, D., Ramadhan, A., and Edel-
man, A. (2021). Universal differential equations for sci-
entific machine learning.
Razali, N. M. and Geraghty, J. (2011). Genetic algorithm
performance with different selection strategiesin solving
TSP. In Proceedings of the World Congress on Engineer-
ing, volume 2, pages 1–6.
Schroeder, M. (1970). Synthesis of low-peak-factor sig-
nals and binary sequences with low autocorrelation
(corresp.). IEEE Transactions on Information Theory,
16(1):85–89.
Silverman, B. W. (1986). Density estimation for statistics
and data analysis. CRC press, 26.
Sivanandam, S. N. and Deepa, S. N. (2008). Introduction to
genetic algorithms. Berlin: Springer.
Smits, V. and Nelles, O. (2021). Genetic optimization of
excitation signals for nonlinear dynamic system identifi-
cation. In Proceedings of the 18th International Confer-
ence on Informatics in Control, Automation and Robotics
- ICINCO, pages 138–145. INSTICC, SciTePress.
Tietze, N. (2015). Model-based calibration of engine con-
trol units using gaussian process regression. PhD thesis,
Technische Universit
¨
at Darmstadt.
Ursem, R. (2002). Diversity-Guided Evolutionary Algo-
rithms. In Parallel Problem Solving from Nature —
PPSN VII, pages 462–471.
ICINCO 2022 - 19th International Conference on Informatics in Control, Automation and Robotics
262