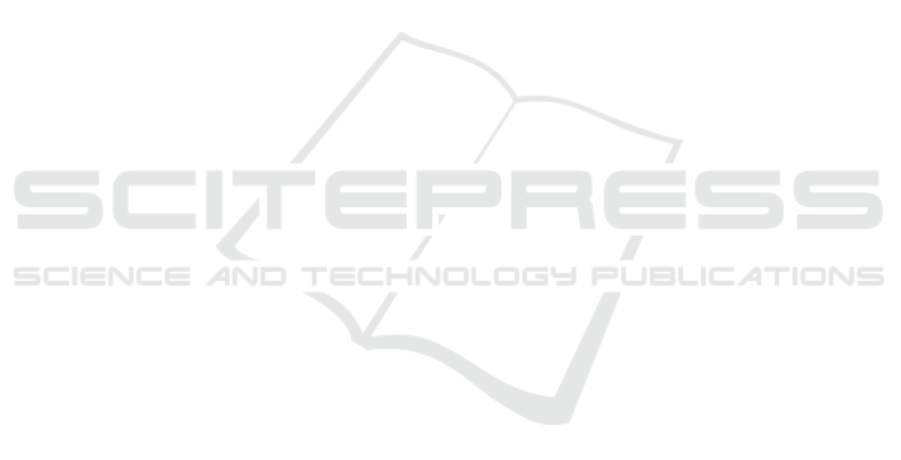
Canziani, A., Paszke, A., and Culurciello, E. (2016). An
analysis of deep neural network models for practical
applications. arXiv preprint arXiv:1605.07678.
Chen, H. (1995). Machine learning for information re-
trieval: Neural networks, symbolic learning, and ge-
netic algorithms. Journal of the American Society for
Information Science, 46(3):194–216.
Cohen, J. P., Dao, L., Morrison, P., Roth, K., Bengio, Y.,
Shen, B., Abbasi, A., Hoshmand-Kochi, M., Ghas-
semi, M., Li, H., et al. (2020). Predicting covid-19
pneumonia severity on chest x-ray with deep learning.
Cureus.
Cui, S. et al. (2020). Fish detection using deep learning.
Applied Computational Intelligence and Soft Comput-
ing.
Da Silva, F. L. and Costa, A. H. R. (2019). A survey on
transfer learning for multiagent reinforcement learn-
ing systems. Journal of Artificial Intelligence Re-
search, 64:645–703.
de Oliveira, R. P. d. C., Sganderla, G. R., Maur
´
ıcio, C.
R. M., and Peres, F. F. F. (2019). Classificac¸ao de
imagens de raio-x de torax com reconhecimento vi-
sual da ibm cloud para diagnostico de pneumonia. In
Anais Estendidos da XXXII Conference on Graphics,
Patterns and Images, pages 203–206. SBC.
de Sousa, O. L., Magalh
˜
aes, D. M., Vieira, P. d. A., and
Silva, R. (2020). Deep learning in image analysis for
covid-19 diagnosis: a survey. IEEE Latin America
Transactions, 100(1e).
dos Santos, Y. C. P., ESTABELECIDAS, C., and
DO NORTE, J. (2017). Desafios e impacto da in-
telig
ˆ
encia artificial na medicina.
Duarte, K. T. N., Gobbi, D. G., Frayne, R., and de Carvalho,
M. A. G. (2020). Detecting alzheimer’s disease based
on structural region analysis using a 3d shape descrip-
tor. In 2020 33rd SIBGRAPI Conference on Graphics,
Patterns and Images (SIBGRAPI), pages 180–187.
Hu, S., Gao, Y., Niu, Z., Jiang, Y., Li, L., Xiao, X., Wang,
M., Fang, E. F., Menpes-Smith, W., Xia, J., Ye, H.,
and Yang, G. (2020). Weakly supervised deep learn-
ing for covid-19 infection detection and classification
from ct images. IEEE Access.
Hu, T., Khishe, M., Mohammadi, M., Parvizi, G.-R.,
Taher Karim, S. H., and Rashid, T. A. (2021).
Real-time covid-19 diagnosis from x-ray images us-
ing deep cnn and extreme learning machines stabilized
by chimp optimization algorithm. Biomedical Signal
Processing and Control, 68:102764.
Ko, H., Chung, H., Kang, W. S., Kim, K. W., Shin, Y.,
Kang, S. J., Lee, J. H., Kim, Y. J., Kim, N. Y., Jung,
H., et al. (2020). Covid-19 pneumonia diagnosis us-
ing a simple 2d deep learning framework with a single
chest ct image: Model development and validation.
Journal of Medical Internet Research, 22(6):e19569.
Kopiler, A. A. et al. (2019). Redes neurais artificiais e suas
aplicac¸
˜
oes no setor el
´
etrico. Revista de Engenharias
da Faculdade Salesiana, (9):27–33.
Lau, S. L. H., Wang, X., Yang, X., and Chong, E. K. P.
(2020). Automated pavement crack segmentation us-
ing fully convolutional u-net with a pretrained resnet-
34 encoder. IEEE Access.
Lei, L., Zhu, H., Gong, Y., and Cheng, Q. (2018). A
deep residual networks classification algorithm of fe-
tal heart ct images. In Intl. Conference on Imaging
Systems and Techniques (IST), pages 1–4. IEEE.
Li, L., Qin, L., Xu, Z., Yin, Y., Wang, X., Kong, B., Bai,
J., Lu, Y., Fang, Z., Song, Q., et al. (2020). Artificial
intelligence distinguishes covid-19 from community
acquired pneumonia on chest ct. Radiology.
Negreiros, R. R. B., dos Santos, R. A., Alves, A. L. F., and
Firmino, A. A. (2020). Oil identification on beaches
using deep learning techniques. In Anais Estendidos
do XXXIII Conference on Graphics, Patterns and Im-
ages, pages 167–170. SBC.
Ohri, K. and Kumar, M. (2021). Review on self-
supervised image recognition using deep neural net-
works. Knowledge-Based Systems, 224:107090.
Os
´
oio, F. S. and Bittencourt, J. R. (2000). Sistemas in-
teligentes baseados em redes neurais artificiais apli-
cados ao processamento de imagens. In I Workshop
de intelig
ˆ
encia artificial.
Phankokkruad, M. (2020). Covid-19 pneumonia detection
in chest x-ray images using transfer learning of con-
volutional neural networks. In Proc. of the 3rd Intl.
Conf. on Data Science and Information Technology,
DSIT 2020, page 147–152, New York, NY, USA. As-
sociation for Computing Machinery.
Rajaraman, S., Siegelman, J., Alderson, P. O., Folio, L. S.,
Folio, L. R., and Antani, S. K. (2020). Iteratively
pruned deep learning ensembles for covid-19 detec-
tion in chest x-rays. IEEE Access.
Rodrigues, J. C. L. et al. (2020). Performance of radiolo-
gists in differentiating covid-19 from viral pneumonia
on chest ct. Public Health Emergency Collection.
Shi, F., Wang, J., et al. (2020). Review of artificial intel-
ligence techniques in imaging data acquisition, seg-
mentation and diagnosis for covid-19. IEEE reviews
in biomedical engineering.
Silva, I., Leoni, G., Sadok, D., and Endo, P. (2021). Clas-
sifying covid-19 positive x-ray using deep learning
models. IEEE Latin America Transactions, 19.
Singh, S., Ahuja, U., Kumar, M., Kumar, K., and Sachdeva,
M. (2021). Face mask detection using yolov3 and
faster r-cnn models: Covid-19 environment. Multi-
media Tools and Applications, 80(13):19753–19768.
Sp
¨
orl, C., Castro, E., and Luchiari, A. (2011). Aplicac¸
˜
ao
de redes neurais artificiais na construc¸
˜
ao de modelos
de fragilidade ambiental. Revista do Departamento de
Geografia, 21:113–135.
Voulodimos, A., Doulamis, N., Doulamis, A., and Protopa-
padakis, E. (2018). Deep learning for computer vi-
sion: A brief review. Computational Intelligence and
Neuroscience, 2018:7068349.
Wong, H. Y. F., Lam, H. Y. S., Fong, A. H., et al. (2020).
Frequency and distribution of chest radiographic find-
ings in patients positive for covid-19. Radiology.
Wynants, L., Van Calster, B., Collins, G. S., Riley, R. D.,
Heinze, G., Schuit, E., Bonten, M. M. J., Dahly, D. L.,
et al. (2020). Prediction models for diagnosis and
prognosis of covid-19: systematic review and critical
appraisal. BMJ, 369.
Zhu, X. and Goldberg, A. B. (2009). Introduction to semi-
supervised learning. Synthesis lectures on artificial
intelligence and machine learning, 3(1):1–130.
WINSYS 2022 - 19th International Conference on Wireless Networks and Mobile Systems
100