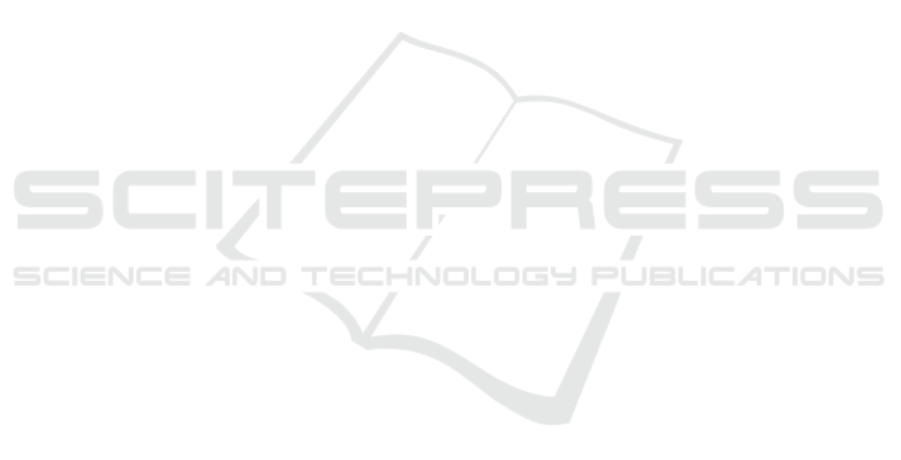
per resolution. IEEE transactions on pattern analysis
and machine intelligence, 36(2):346–360.
Liu, H., Ruan, Z., Zhao, P., Dong, C., Shang, F., Liu, Y.,
and Yang, L. (2020). Video super resolution based
on deep learning: A comprehensive survey. arXiv
preprint arXiv:2007.12928.
Liu, Z.-S., Siu, W.-C., and Chan, Y.-L. (2021). Efficient
video super-resolution via hierarchical temporal resid-
ual networks. IEEE Access, 9:106049–106064.
Lucas, A., Lopez-Tapia, S., Molina, R., and Katsaggelos,
A. K. (2019). Generative adversarial networks and
perceptual losses for video super-resolution. IEEE
Trans Image Process, 28(7):3312–3327.
Nah, S., Baik, S., Hong, S., Moon, G., Son, S., Timofte,
R., and Mu Lee, K. (2019). Ntire 2019 challenge
on video deblurring and super-resolution: Dataset and
study. In Proceedings of the IEEE/CVF Conference
on Computer Vision and Pattern Recognition (CVPR)
Workshops.
Paszke, A., Gross, S., Massa, F., Lerer, A., Bradbury, J.,
Chanan, G., Killeen, T., Lin, Z., Gimelshein, N.,
Antiga, L., Desmaison, A., Kopf, A., Yang, E., De-
Vito, Z., Raison, M., Tejani, A., Chilamkurthy, S.,
Steiner, B., Fang, L., Bai, J., and Chintala, S. (2019).
Pytorch: An imperative style, high-performance deep
learning library. In Wallach, H., Larochelle, H.,
Beygelzimer, A., d’Alch
´
e-Buc, F., Fox, E., and Gar-
nett, R., editors, Advances in Neural Information Pro-
cessing Systems 32, pages 8024–8035. Curran Asso-
ciates, Inc.
Sajjadi, M. S., Vemulapalli, R., and Brown, M. (2018).
Frame-recurrent video super-resolution. In Proceed-
ings of the IEEE Conference on Computer Vision and
Pattern Recognition, pages 6626–6634.
Tao, X., Gao, H., Liao, R., Wang, J., and Jia, J. (2017).
Detail-revealing deep video super-resolution. In Pro-
ceedings of the IEEE International Conference on
Computer Vision, pages 4472–4480.
Tian, Y., Zhang, Y., Fu, Y., and Xu, C. (2020). Tdan:
Temporally-deformable alignment network for video
super-resolution. In Proceedings of the IEEE/CVF
Conference on Computer Vision and Pattern Recog-
nition (CVPR).
Wang, H., Su, D., Liu, C., Jin, L., Sun, X., and Peng,
X. (2019a). Deformable non-local network for video
super-resolution. IEEE Access, 7:177734–177744.
Wang, X., Chan, K. C., Yu, K., Dong, C., and Change Loy,
C. (2019b). Edvr: Video restoration with enhanced
deformable convolutional networks. In Proceedings
of the IEEE/CVF Conference on Computer Vision and
Pattern Recognition Workshops, pages 0–0.
Wang, Z., Chen, J., and Hoi, S. C. (2020). Deep learning for
image super-resolution: A survey. IEEE transactions
on pattern analysis and machine intelligence.
Xue, T., Chen, B., Wu, J., Wei, D., and Freeman,
W. T. (2019). Video enhancement with task-oriented
flow. International Journal of Computer Vision,
127(8):1106–1125.
Zhu, X., Li, Z., Zhang, X.-Y., Li, C., Liu, Y., and Xue, Z.
(2019). Residual invertible spatio-temporal network
for video super-resolution. In Proceedings of the AAAI
conference on artificial intelligence, volume 33, pages
5981–5988.
SIGMAP 2022 - 19th International Conference on Signal Processing and Multimedia Applications
58