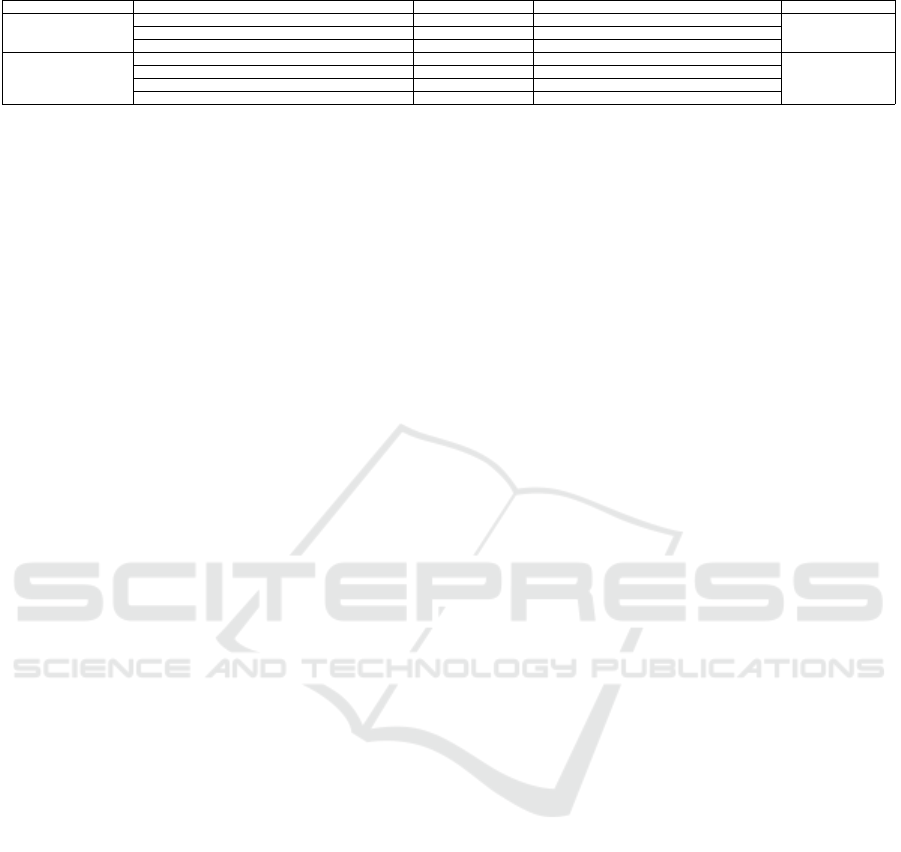
Table 3: Results on EJUST-SQUAT-21 Dataset and Single Individual Dataset.
Training Dataset Pretraining Dataset Number of Epochs Accuracy Type of classification
EJUST-SQUAT-21 dataset
- 83 (end-to-end training) 94% (5-Fold Cross Validation)
multi-label multi-classSingle Individual Dataset for 60 epochs (Ogata et al., 2019) 38 (fine-tuning) 90.3% (5-Fold Cross Validation)
MM-Fit Dataset (Str
¨
omb
¨
ack et al., 2020) for 25 epochs 200 (fine-tuning) 88% (5-Fold Cross Validation)
Single Individual Dataset
- 60 (end-to-end training) 87% (80%-10%-10% training-validation-test split)
multi-class
- 60 (end-to-end training) 80% (60%-10%-30% training-validation-test split)
EJUST-SQUAT-21 for 83 epochs 54 (fine-tuning) 80.2% (80%-10%-10% training-validation-test split)
MM-Fit Dataset (Str
¨
omb
¨
ack et al., 2020) for 25 epochs 120 (fine-tuning) 73% (80%-10%-10% training-validation-test split)
dataset. A wide range of tasks will need to be done,
including the recognition of the exercise, the level of
performance, the mistakes done, etc. Transfer learn-
ing will play a major role as well in the effectiveness
of developing such a virtual coaching system.
ACKNOWLEDGEMENTS
This work is funded by the Information Technol-
ogy Industry Development Agency (ITIDA), Infor-
mation Technology Academia Collaboration (ITAC)
Program, Egypt – Grant Number (ARP2020.R29.2
- VCOACH: Virtual Coaching for Indoors and Out-
doors Sporting).
REFERENCES
Abou Elmagd, M. (2016). Benefits, need and importance
of daily exercise. Int. J. Phys. Educ. Sports Health,
3(5), 22–27.
Bazarevsky, V., Grishchenko, I., Raveendran, K., Zhu, T.,
Zhang, F., & Grundmann, M. (2020). Blazepose:
On-device real-time body pose tracking. arXiv
preprint arXiv:2006.10204.
Bernardino, A., Vismara, C., i Badia, S. B., Gouveia,
´
E.,
Baptista, F., Carnide, F., . . . Gamboa, H. (2016).
A dataset for the automatic assessment of func-
tional senior fitness tests using kinect and physiolog-
ical sensors. In 2016 1st international conference
on technology and innovation in sports, health and
wellbeing (tishw) (pp. 1–6).
Cao, Z., Hidalgo, G., Simon, T., Wei, S.-E., & Sheikh, Y.
(2019). Openpose: realtime multi-person 2d pose
estimation using part affinity fields. IEEE transac-
tions on pattern analysis and machine intelligence,
43(1), 172–186.
Capecci, M., Ceravolo, M. G., Ferracuti, F., Iarlori, S.,
Monteri
`
u, A., Romeo, L., & Verdini, F. (2019). The
kimore dataset: Kinematic assessment of movement
and clinical scores for remote monitoring of physical
rehabilitation. IEEE Transactions on Neural Systems
and Rehabilitation Engineering, 27(7), 1436–1448.
Ch
´
eron, G., Laptev, I., & Schmid, C. (2015). P-cnn:
Pose-based cnn features for action recognition. In
Proceedings of the ieee international conference on
computer vision (pp. 3218–3226).
Fayez, A., Sharshar, A., Hesham, A., Eldifrawi, I., & Go-
maa, W. (2022). Vals: A leading visual and inertial
dataset of squats. In 2022 16th international con-
ference on ubiquitous information management and
communication (imcom) (pp. 1–8).
F
¨
uz
´
eki, E., Groneberg, D. A., & Banzer, W. (2020). Physi-
cal activity during covid-19 induced lockdown: rec-
ommendations. Journal of Occupational Medicine
and Toxicology, 15(1), 1–5.
Garber, C., Blissmer, B., Deschenes, M., Franklin, B., Lam-
onte, M., Lee, I.-M., . . . Swain, D. (2011, 07). Quan-
tity and quality of exercise for developing and main-
taining cardiorespiratory, musculoskeletal, and neu-
romotor fitness in apparently healthy adults: Guid-
ance for prescribing exercise. Medicine and science
in sports and exercise, 43, 1334-59. doi: 10.1249/
MSS.0b013e318213fefb
He, K., Zhang, X., Ren, S., & Sun, J. (2016). Deep residual
learning for image recognition. In Proceedings of
the ieee conference on computer vision and pattern
recognition (pp. 770–778).
Hung, J.-S., Liu, P.-L., & Chang, C.-C. (2020). A deep
learning-based approach for human posture classifi-
cation. Proceedings of the 2020 2nd International
Conference on Management Science and Industrial
Engineering.
Iqbal, U., Garbade, M., & Gall, J. (2017). Pose for action -
action for pose. 2017 12th IEEE International Con-
ference on Automatic Face & Gesture Recognition
(FG 2017), 438-445.
Kanazawa, A., Black, M. J., Jacobs, D. W., & Malik, J.
(2018a). End-to-end recovery of human shape and
pose. In Proceedings of the ieee conference on
computer vision and pattern recognition (pp. 7122–
7131).
Kanazawa, A., Black, M. J., Jacobs, D. W., & Malik, J.
(2018b). End-to-end recovery of human shape and
pose. 2018 IEEE/CVF Conference on Computer Vi-
sion and Pattern Recognition, 7122-7131.
Kaur, H., Singh, T., Arya, Y. K., & Mittal, S. (2020). Phys-
ical fitness and exercise during the covid-19 pan-
demic: a qualitative enquiry. Frontiers in psychol-
ogy, 11, 2943.
Ke, Q., Bennamoun, M., An, S., Sohel, F., & Boussaid, F.
(2017). A new representation of skeleton sequences
for 3d action recognition. In Proceedings of the ieee
conference on computer vision and pattern recogni-
tion (pp. 3288–3297).
Kuehne, H., Jhuang, H., Garrote, E., Poggio, T., & Serre,
T. (2011). Hmdb: a large video database for human
motion recognition. In 2011 international confer-
ence on computer vision (pp. 2556–2563).
Liao, Y., Vakanski, A., & Xian, M. (2020, Feb.). A deep
learning framework for assessing physical rehabilita-
tion exercises. IEEE Transactions on Neural Systems
and Rehabilitation Engineering, 28(2), 468-477.
Lorenzetti, S., Ostermann, M., Zeidler, F., Zimmer, P.,
Analysis of the Squat Exercise from Visual Data
87