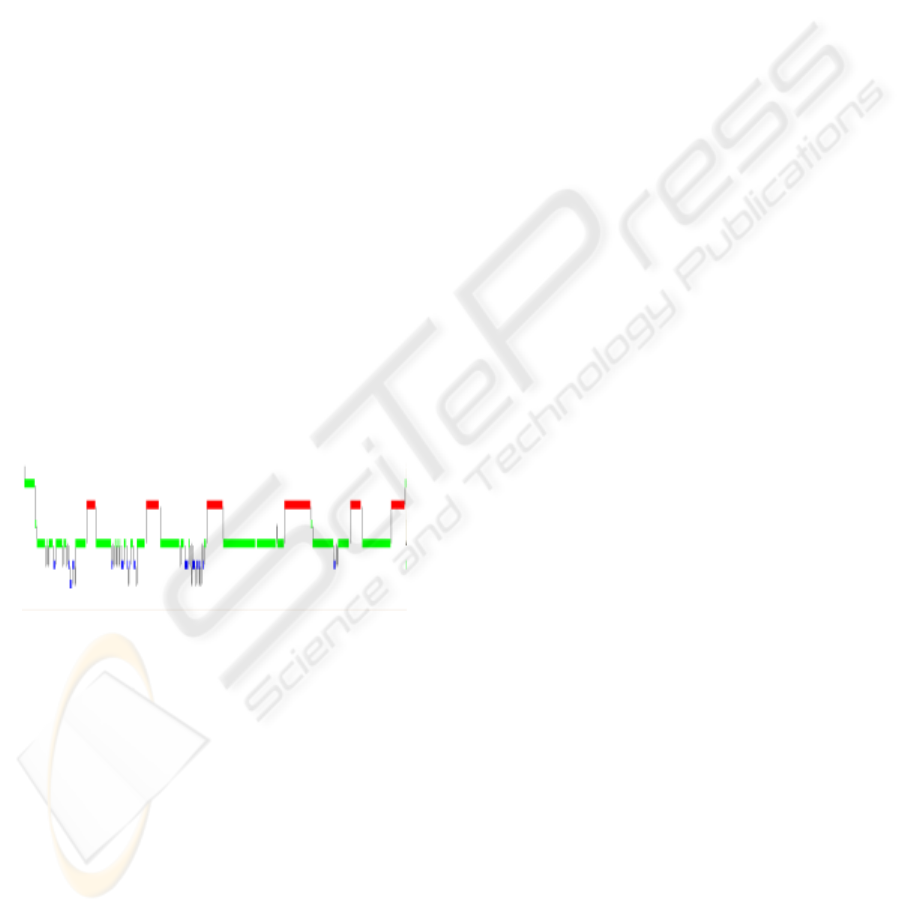
2 SLEEP DATA
Generally speaking, sleep is a kind of brain activity
and its purpose is recovery from brain fatigue.
Therefore, sleep state is measured mainly by EEG,
and is classified into several stages. Sleep state is
roughly divided into REM (rapid-eye movement)
sleep and NREM (Non-REM) sleep. NREM sleep is
divided into 4 stages. Stages 3 and 4 of NREM sleep
are so called deep sleep, and stages 1 and 2 are
shallow sleep. These stages are decided by a sleep
specialist using PSG data (Rechtschaffen, 1968), and
their change is shown in a graph called a hypnogram
(shown in Figure 1).
A doctor mainly uses a hypnogram for
evaluating a person’s sleep quality. For example, the
doctor checks the quantity of deep sleep if a patient
complains about oppressive drowsiness in the
daytime. If the patient frequently wakes up in the
night and experiences difficulty in breathing, he/she
might be suffering from sleep apnea syndrome. If
REM sleep always occurs soon after falling asleep,
there might be a problem concerning the patient’s
nervous system. From the viewpoint of healthcare, it
is important to check the balance of deep sleep,
REM sleep or sleep cycle. Therefore, a sleep
monitoring system for home use can also show the
result of one night’s data in a graph similar to a
hypnogram.
Figure 1: Sleep hypnogram.
There are many studies on the relationship between
physiological parameters and sleep stages. For
example, Baharav et al. stated that autonomic
nervous activity level derived from heart rate
variability (HRV) during sleep changes in response
to the sleep stages (Baharav, 1995). A value of
LF/HF shows the activity of the sympathetic nerve.
During a REM sleep, a value of LF/HF and the
variability of that are large, and the value of LF/HF
decreases during a NREM sleep, particularly in the
case of deep sleep (Slow Wave Sleep). Since the
brain stem controls both the cerebrum and the
autonomic nervous system, it may be possible to
estimate the sleep stage using HRV.
3 RELATED WORKS
A number of trials have been conducted with a view
to developing sleep monitors for home use. For
example, body/wrist motion has been used for
wake/sleep identification.
The amount of activity
(number of subtle wrist motions per minute)
measured
from acceleration sensors is often used for
monitoring wake/sleep rhythms (Sadeh, 1989)
although the sleep stages (ex. REM sleep / NREM
sleep) cannot be determined from the data.
More recently, researchers have focused on
measuring heart/pulse rate and analyzing its
variability: HRV (Watanabe, 2004, Michimori, 2003
and Wakuda, 2007). The sleep stages can be
calculated from HRV if the indices of HRV are
properly mapped for the sleep stages.
However, there are two problems in this
approach. One is that body/wrist motion often
disturbs heart/pulse sensing and the HRV value can
not be calculate correctly. The other is that the level
of autonomic nervous activity differs according to
age, sex and body/mental condition. For example,
the autonomic nervous system of the young is
generally more active than that of the old. Sleep
stages cannot be classified using static thresholds.
Our sensor measures both pulse wave interval
and wrist motion. The wrist motion data are used not
only for counting the amount of activity, but also for
detecting errors in HRV data. This solves the first
problem mentioned above.
For the second problem, we employ a statistical
method for deciding sleep stages (Suzuki, 2007). We
assume that there are several stages in a certain
period of sleeping time since the sleep stage
cyclically repeats about every 90 minutes. It means
that the data of autonomic nervous activity can be
classified into several groups if we have any 90-120
minute dataset. In this way, the thresholds for
dividing sleep stages are changed flexibly along with
the dataset.
4 THE OVERVIEW OF THE
SYSTEM
4.1 Wearable Physiological Sensor
Figure 2 shows our wearable physiological sensor.
The size of the sensor is 50mm*60mm*13mm and
the weight is only 35g. A rechargeable battery is
used as an electrical power source. It is possible to
measure physiological data for over 40 hours after
Wake
REM
Stage1
Stage2
Stage3
Stage4
DEVELOPMENT OF A SLEEP MONITORING SYSTEM WITH WEARABLE VITAL SENSOR FOR HOME USE
327