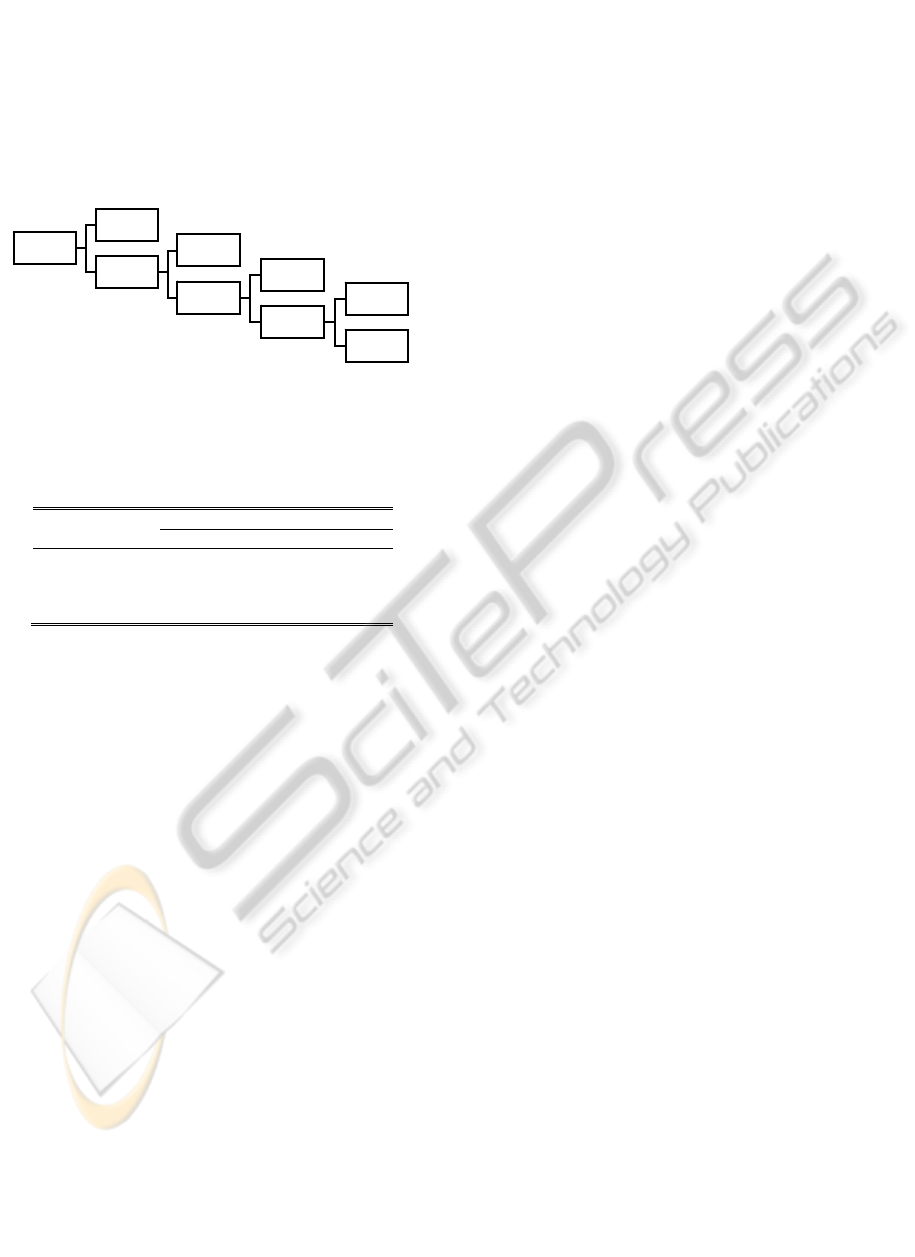
classification. The results were compared with and
without feature selection (Table 5). The
improvements from feature selection are evident. The
results obtained with our ASSC system are
comparable to the ones obtained in other methods
based on EEG only described in literature (zoubek et
al, 2007; Doroshenkov et al, 2007).
Figure 4: Decision tree based on 2-class detection.
Table 5: Disagreement obtained with using 19 most
discriminative features and all 204 in 2, 3, 4 and 5 sleep
stages classification.
Classification
Diasagreement (%)
All Features 19
2 Class
36 7
3 Class
62 18
4 Class
83 22
5 Class
83 29
7 CONCLUSIONS
In this paper, the use of several feature extraction
methods was investigated in the context of EEG-
based sleep staging. The first conclusion was that the
most discriminative features were determined by
RSP, SWI, Harmonic Parameters and Parameters of
Hjorth. All the 6-EEG channels provide useful
information. On the other hand, the application of
the feature selection method improved, in general,
the process of discrimination by selecting the set of
features that provided a lower percentage of
disagreement. One of the biggest problems in
automatic sleep staging based on EEG is the
similarity between patterns of different sleep stages
such as REM and NREM N1. This can be improved
recurring to other biosignals, such as EOG and
EMG. Another problem in ASSC is the high level of
variability between patients. Using an ambulatory
system, the patient can perform periodic recordings
at home. This way, the first session can be fully
analysed by the expert. The labelled data can be
used to obtain classification models specific to the
patient. Further sessions can then use these robust
user-dependent models. This approach is
under research presently.
REFERENCES
Ansari-Asl. K., Chanel, G., Pun, T., A channel Selection
Method for EEG Classification in Emotion
Assessment Based on Synchronization Likelihood. In
EUSIPCO’07, 1241-1245.
Doroshenkov, L., Konyshev, V., Selishchev, S., 2007,
Classification of Human Sleep Stages Based on EEG
Processing Using Hidden Markov Models. Biomedical
Engineering, 41(1), 25-28.
Ebrahimi, F., Mikaeili, M., Estrada, E., Nazeran, H., 2008,
Automatic Sleep Stage Classification Based on EEG
Signals by Using Neural Networks and Wavelet
Packet Coefficients. In IEEE EMBS’08, 1, 1151-1154.
Guyon, I., Elisseeff, A., 2003, An introduction to variable
and feature selection. Journal of Machine Learning
Research, 3, 1157-1182.
Heijden, F., Duin, R., Ridder, D., Tax, D., 2004,
Classification, Parameter Estimation and State
Estimation. John Wiley & Sons.
Hese, P., Philips, W., Koninck, J., Walle, R., Lemahieu, I.,
2001, Automatic Detection of Sleep Stages Using the
EEG. In IEEE EMBS’01,Proc. of, 1994-1947.
Iber., C., Ancoli-Israel, S., Chesson, A., Quan, S., 2007,
The AASM Manual for the scoring of Sleep and
Associated Events: Rules. Terminology and Technical
Specifications (1st ed.). Westchester, Illinois:
American Academy of Sleep Medicine.
Rechtscheffen, A., Kales, A., 1968, A Manual of
Standardized Terminology, Techniques ans Scoring
System for Sleep Stages of Human Subjects, US
Government Printing Office, National Institute of
Health Publications, Washington DC.
Schwaibold, M., Harms, R., Scholer, B., Pinnow, I.,
Cassel, W., Penzel, T., Becker, H., Bolz, A., 2003,
Knowledge-Based Automatic Sleep-Stage Recognition
– Reduction in the Interpretation Variability.
Somnologie, 7, 59-65.
Tang, W. C., Lu, S. W., Tsai. X. M., Kao. C. Y., Lee. H.
H., 2007, Harmonic Parameters with HHT and
Wavelet Transform for Automatic Sleep Stages
Scoring. Proc. of World Academy of Science.
Engineering and Technology, 22, 414-417.
Yilmaz, A., Alkan, A., Asyali, M., 2007, Applications of
parametric spectral estimation methods on detection of
power system harmonics. Electric Power Systems
Research (2008), 78, 683-693.
Zoubek, L., Charbonnier, S., Lesecq, S., Buguet, A.,
Chapolot, F., 2007, Feature selection for sleep/wake
stages classification using data driven methods.
Biomedical Signal Processing and Control, 2, 171-
179.
N3
Level 2
Level 3
Wake
Epoch
Sleep
REM
N1
N2/N3
NREM
N2
Level 1
Level 4
FEATURE EXTRACTION AND SELECTION FOR AUTOMATIC SLEEP STAGING USING EEG
133