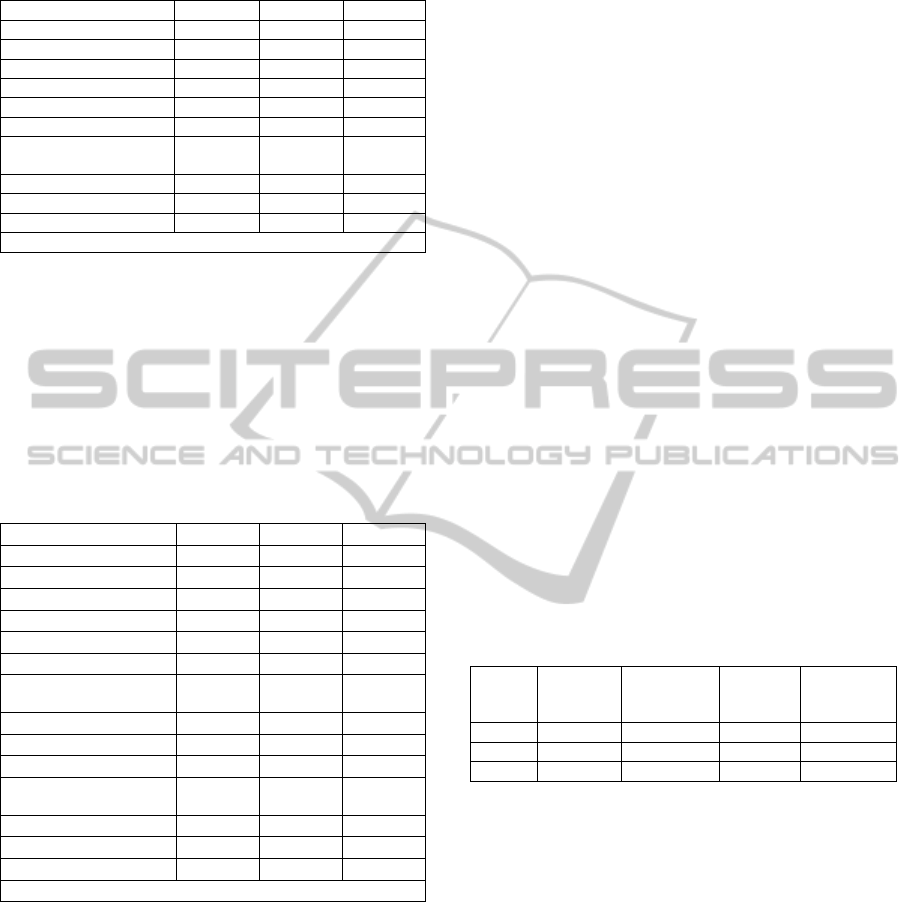
Table 4: Estimated coefficients of the quadratic regression
models, by group.
Group 1 Group 2 Group 3
Intercept 0.32*** 0.35*** 0.33***
Business density -0.18*** 0.18*** 0.01
Density of the local units 0.07 -0.11* 0.04*
Rate of evolution -0.11*** 0.09** 0.02
Export propensity -0.08*** -0.15*** 0.24***
Business density (^2) 0.05** -0.04* -0.01
Density of the local units
(^2)
-0.02* 0.02* -0.01
Rate of evolution (^2) -0.04*** 0.02** 0.01
Export propensity (^2) 0.01 0.03** -0.04***
Adjusted R
2
0.71*** 0.62*** 0.71***
Index of reliability of the discriminant model 0. 68
*** significant at 0.01 level ** significant at 0.05 level
* significant at 0.10 level
The simple average of the adjusted R
2
coefficients of determination still grows (0.77) in the
case of cubic regressions, because the latter ones
become even more flexible and allow a better
representation of awarding of the sampled provinces
to the three identified groups (Table 5).
Table 5: Estimated coefficients of the cubic regression
models, by group.
Group 1 Group 2 Group 3
Intercept 0,28*** 0,42*** 0,29***
Business density -0,25*** 0,27*** -0,03
Density of the local units 0,02 -0,15** 0,13**
Rate of evolution -0,13*** 0,10*** 0,03
Export propensity -0,07** -0,15*** 0,22***
Business density (^2) 0,01 0,00 -0,01
Density of the local units
(^2)
0,02 0,08* -0,09**
Rate of evolution (^2) -0,01 0,00 0,02
Export propensity (^2) 0,07* -0,12*** 0,05
Business density (^3) 0,04** -0,05*** 0,01
Density of the local units
(^3)
0,00 -0,01 0,01**
Rate of evolution (^3) 0,01 -0,01 0,00
Export propensity (^3) -0,01 0,04*** -0,02***
Adjusted R
2
0,76*** 0,79*** 0,77***
Index of reliability of the discriminant model 0. 77
*** significant at 0.01 level ** significant at 0.05 level
* significant at 0.10 level
Since the discriminant model defined via cubic
functions reaches a remarkable goodness of fit, we
prefer not to further increase the order of
polynomials, so as to maintain a parsimonious
number of the involved independent variables and to
allow an easier interpretability of the obtained
estimates.
The examination of the estimated regression
coefficients confirms what emerges from an
overview of the average profiles of the groups, since
the variables with a greater discriminating power in
the definition of degrees of membership seem to
correspond to those discussed above. In particular
the
business density and the rate of evolution are the
most significant variables in explaining the
assignment to the first two groups, while the
export
propensity and density of local units to the third
group (actually characterized by high average values
of the latter ones).
Note that the
business density is not significant at
all in determining the degree of membership of a
province to the third group, likely because the
corresponding average value is close to that of the
second one. Similar consideration can be done be
with reference both to the
density of the local units,
which is not significant in determining the degree of
membership to the first group, and to the
rate of
evolution
, which is not significant in determining the
degree of membership to the third group.
In general terms an independent variable,
expressed in cubic terms, helps in discriminating one
group from another when the gap between the
corresponding average values of the same
(standardized) independent variable is remarkable.
For clarity we present the average values of all
the standardized indicators for each group of
provinces in Table 6.
Table 6: Average values of the standardized indicators
weighted by the degrees of membership of each province,
by group.
Business
density
Density of
the local
units
Rate of
evolution
Export
propensity
Group 1 -0,46 0,06 -0,46 -0,20
Group 2 0,51 -0,04 0,20 -0,18
Group 3 0,20 0,23 0,10 0,68
On the basis of such an estimation procedure, it
is possible to determine the degrees of membership
to the three groups for each of the 20% of the
provinces not yet taken into account (Table 7).
Such degrees are compared with the analogue
posterior probabilities of assignment, deriving from
a classical discriminant analysis based on Bayes'
theorem, in order to evaluate their reliability. Note
that the used prior probabilities coincide with the
proportions of sampled provinces included in each
of the three groups within a classification procedure
based on the found maximum degree of
membership.
Before conducting such a classical discriminant
analysis, we have assessed – by means of the
coefficient of kurtosis defined by Mardia - the actual
IJCCI2013-InternationalJointConferenceonComputationalIntelligence
262