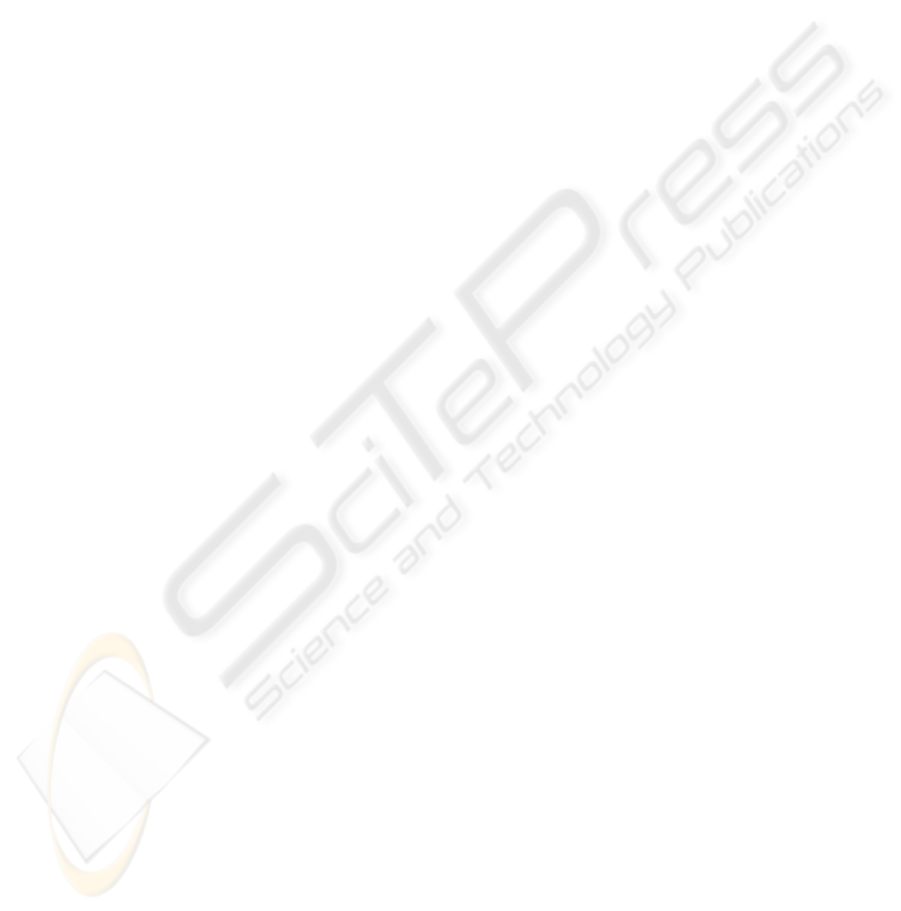
Discussion. LexInfo and LIR are both conceptualisa-
tions of lexicalising a domain ontology in the form of
an ontology structure and they both aim at integrating
the same existing standards (LMF). Still the resulting
ontological structures are quite different. Firstly they
are different from a syntactic point of view. Second,
they also differ semantically: LexInfo rather empha-
sises the representation of properties and in particular
the syntax ↔ semantics interface. For example with
LexInfo it would be possible to express that the sen-
tences The train speeds at 100 km/h. and The speed
of the train is 100km/h. are linguistic realisations of
the same meaning. LIR adopts a more traditional lex-
icographic position: one could express for example
that train and rame are both possible French transla-
tions of the English train, that they may be used as
synonyms in most cases but are not entirely synony-
mous. LexInfo is arguably more suitable at verbalis-
ing ontologies or translating natural language queries
to database or web search queries, whereas LIR would
be more useful to human knowledge engineers or lexi-
cographers for building and maintaining a domain and
lexicon ontology. It is in principle possible to trans-
late one lexicon format to the other, but the syntax –
semantics mapping information of LexInfo can not be
extracted from LIR and conversely, the lexicographic
aspects represented in LIR can not be generated from
LexInfo. On this note the two models are comple-
mentary. This difference in perception also implies
different (semi-)automatic acquisition methods from
texts: to enrich a LexInfo lexicon one needs deep
syntactic and semantic linguistic analysis whereas for
LIR one would make use of statistic semantic simi-
larity measures and high-quality linguistic semantic
resources as (Euro)WordNet or Wikipedia. From the
SEMbySEM position a point in favour of LexInfo is
that it generates a separate lexicon for each language
whereas the fact that SEMbySEM needs lie more on
the side of ontology building (by humans) speeks in
favour of LIR. These differences also entail different
APIs and differences in the manipulation of the lexi-
cal ontologies.
5 CONCLUSIONS
In this paper we presented the SEMbySEM seman-
tic model and explored ways of associating multilin-
gual information to its elements. Two state-of-the-
art techniques for representing and associating lin-
guistic information to ontological structures are Lex-
Info and LIR. We presented and compared the prin-
ciples of these models and investigated whether and
how they could be integrated with the SEMbySEM
semantic model. LexInfo and LIR both are designed
as lexical ontologies building on a domain ontology
represented in OWL. Although the SEMbySEM se-
mantic model will not be represented as an OWL on-
tology, it will be designed following the same basic
principles and it will therefore be possible to repre-
sent the linguistic information using LIR or LexInfo.
We showed that LIR and LexInfo take up different
positions mainly with respect to the kind of linguis-
tic information they focus on: the syntax – seman-
tics interface for LexInfo and more traditional lex-
icographic aspects for LIR. These differences entail
different acquisition methods, different APIs and dif-
ferences in the handling of the obtained lexical on-
tologies. Both present advantages compared to the
current way of representing linguistic information by
the rdfs:label and rdfs:comment elements, in that
they clearly separate domain knowledge from lexical
knowledge. Thus domain and lexicon ontology can
be developed separately by domain and linguistic ex-
perts and can be more easily maintained and reused.
However, at this stage of the project we have not yet
decided which of them to use.
ACKNOWLEDGEMENTS
This work is carried out by the EUREKA ITEA2
project SEMbySEM partly funded by French, Span-
ish, Finnish and Turkish governments. We would
also like to thank Elena Montiel-Ponsoda and Philipp
Cimiano for providing very helpful insights regarding
the LIR and LexInfo model respectively.
REFERENCES
Brunner, J.-S., Goudou, J.-F., Gatellier, P., Beck, J., and
Laporte, C.-E. (2009). SEMbySEM: a Framework for
Sensors Management. In 1st International Workshop
on the Semantic Sensor Web (SemSensWeb 2009).
Buitelaar, P., Cimiano, P., Haase, P., and Sintek, M. (2009).
Towards Linguistically Grounded Ontologies. In
The 6th Annual European Semantic Web Conference
(ESWC2009), Heraklion, Greece.
Buitelaar, P., Declerck, T., Frank, A., Racioppa, S., Kiesel,
M., Sintek, M., Engel, R., Romanelli, M., Sonntag,
D., Loos, B., Micelli, V., Porzel, R., and Cimiano,
P. (2006). Linginfo: Design and applications of a
model for the integration of linguistic information in
ontologies. In Proceedings of the OntoLex Workshop
at LREC, pages 28–32. ELRA.
Cimiano, P., Haase, P., Herold, M., Mantel, M., and Buite-
laar, P. (2007). LexOnto: A Model for Ontology
Lexicons for Ontology-based NLP. In Proceedings
KEOD 2009 - International Conference on Knowledge Engineering and Ontology Development
450