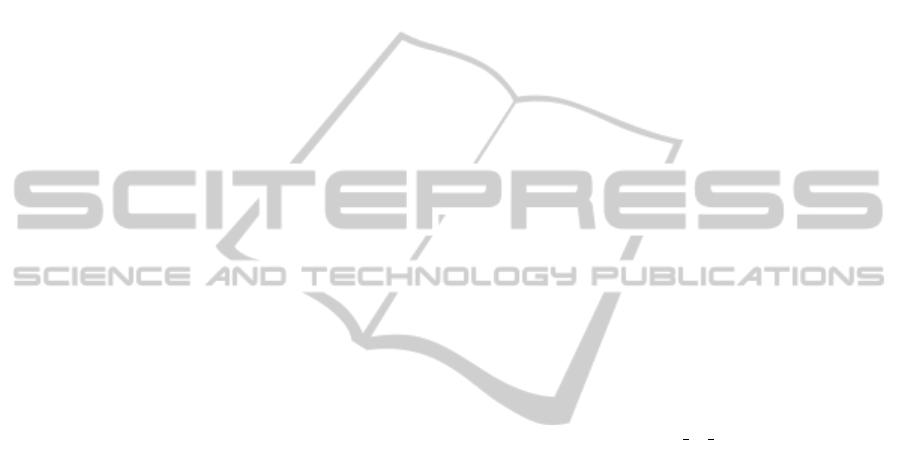
the suggested improvements by the users, we con-
cluded that the lack of safety and the difficulty in nav-
igating the powered wheelchair were the most limit-
ing factors, in general, and also the most suggested
(57,14%) to be improved. The ANS was tested both
in simulated environment conditions and in real con-
ditions. The promising results show that the collab-
orative controller and other modules of the ANS ar-
chitecture compose a structure on which it is worth
continuing to devote research effort.
Future work includes adding short range sensors
for close distances, improving the collaborative con-
trol methodology to allow safe navigation in human
environments, and also solving other remaining issues
pointed out by powered wheelchair users. Getting a
larger user sample could also point us toward future
research directions.
ACKNOWLEDGEMENTS
This work has been supported by the FCT project
”AMS-HMI2012 - RECI/EEI-AUT/0181/2012” and
project ”ProjB-Diagnosis and Assisted Mobility
- Centro-07-ST24-FEDER-002028” with FEDER
funding, programs QREN and COMPETE.
REFERENCES
Borenstein, J. and Koren, Y. (1991). The vector field
histogram-fast obstacle avoidance for mobile robots.
Robotics and Automation, IEEE Transactions on,
7(3):278–288.
Carona, C., Canavarro, M. C., Pereira, M., Vaz-Serra, A.,
Quartilho, M., Paredes, T., Rijo, D., Gameiro, S., and
Sim˜oes, M. (2010). Qualidade de vida de indiv´ıduos
adultos com paralisia cerebral e dos seus cuidadores
familiares. In Qualidade de vida e sa´ude – Uma
abordagem na perspectiva da Organizac¸˜ao Mundial
de Sa´ude (in Portuguese). Fundac¸˜ao Calouste Gul-
benkian.
Carona, C., Reis, P., Almeida, L., Pires, G., Lopes, A.,
Almeida, A., Machado, D., Vaz, L., Moita, F., Carid´a,
V., Castela-Lobo, J., Figueira, A., Antunes, F., Bran-
quinho, A., Elias, C., and Nunes, U. (2012). Mobili-
dade, acessibilidade e participac¸˜ao em indiv´ıduos com
paralisia cerebral (in portuguese). Technical report,
Institute of Systems and Robotics, Coimbra Cerebral
Palsy Association, Cognitive and Behavioural Center
for Research and Intervention - University of Coim-
bra.
Dickinson, H. O., Parkinson, K. N., Ravens-Sieberer, U.,
Schirripa, G., Thyen, U., Arnaud, C., Beckung, E.,
Fauconnier, J., McManus, V., Michelsen, S. I., Parkes,
J., and Colver, A. F. (2007). Self-reported qual-
ity of life of 8-12-year-old children with cerebral
palsy: a cross-sectional european study. Lancet,
369(9580):2171–2178.
Gonzalez-Jimenez, J., Ruiz-Sarmiento, J., and Galindo, C.
(2013). Improving 2d reactive navigators with kinect.
In Int. Conf. on Informatics in Control, Automation
and Robotics, ICINCO 2013.
Health Statistics, N. C. f. and Health & Human Services, U.
S. D. (1988). National health interview survey: Child
health supplement.
Kennes, J., Rosenbaum, P., Hanna, S. E., Walter, S., Rus-
sell, D., Raina, P., Bartlett, D., and Galuppi, B.
(2002). Health status of school-aged children with
cerebral palsy: information from a population-based
sample. Developmental Medicine & Child Neurology,
44(4):240–247.
Koman, L. A., Smith, B. P., and Balkrishnan, R. (2003).
Spasticity associated with cerebral palsy in children:
guidelines for the use of botulinum a toxin. Pediatric
Drugs, 5(1):11–23.
Lopes, A., Pires, G., and Nunes, U. (2012). Robchair:
Experiments evaluating brain-computer interface to
steer a semi-autonomous wheelchair. In Intelligent
Robots and Systems (IROS), 2012 IEEE/RSJ Interna-
tional Conference on, pages 5135–5136.
Lopes, A. C., Pires, G., and Nunes, U. (2013). Assisted
navigation for a brain-actuated intelligent wheelchair.
Robot. Auton. Syst., 61.
Palisano, R., Rosenbaum, P., Walter, S., Russell, D., Wood,
E., and Galuppi, B. (1997). Gross motor function clas-
sification system. Developmental Medicine & Child
Neurology, 39(4):214–223.
Rockey, C. (2013). depthimage
to laserscan - ros wiki.
Vargus-Adams, J. (2003). Health-related quality of life
in childhood cerebral palsy. Archives of Physical
Medicine Rehabilitation, 86(5):940–945.
Varni, J. W., Limbers, C. A., and Burwinkle, T. M. (2007).
Impaired health related quality of life in children
and adolescents with chronic conditions: A compar-
ative analysis of 10 disease clusters and 33 disease
categories/severities utilizing the pedsql 4.0 generic
core scales. Health and Quality of Life Outcomes,
5(43):673–681.
Wake, M., Salmon, L., and Reddihough, D. (2003). Health
status of australian children with mild to severe cere-
bral palsy: cross-sectional survey using the child
health questionnaire. Developmental Medicine and
Child Neurology, 45(3):194–199.
Zaffuto-Sforza, C. D. (2005). Aging with cerebral palsy.
Physical Medicine and Rehabilitation Clinics of North
America, 16(1):235–249.
Mobility,AccessibilityandSafetyofPeoplewithCerebralPalsy
275