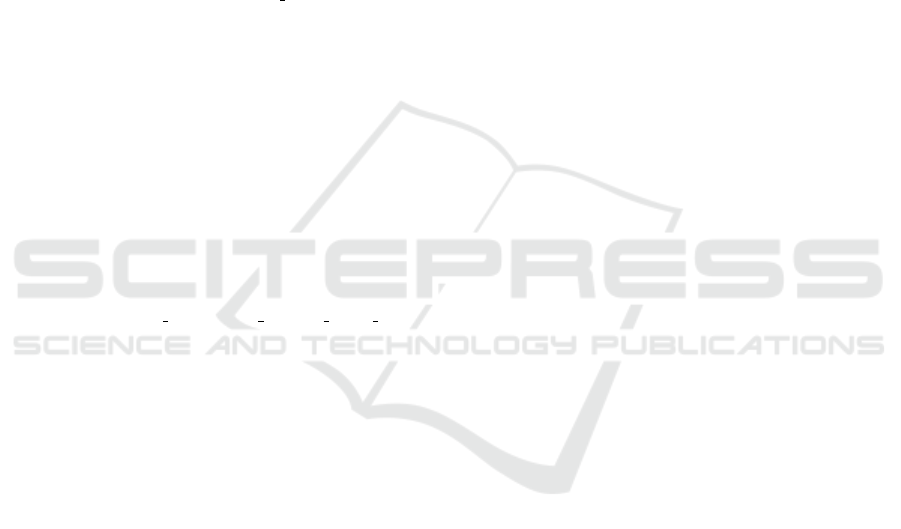
Duhigg, C. (Feb 16, 2012). How companies learn
your secrets. http://www.nytimes.com/2012/
02/19/magazine/shopping-habits.html. New York
Times.
Economist (Apr 7, 2016a). Facebook, the world’s
most addictive drug. http://www.economist.com/
blogs/graphicdetail/2016/04/daily-chart-5. The
Economist.
Economist (Apr 9, 2016b). The new face of face-
book: How to win friends and influence people.
http://www.economist.com/news/briefing/21696507-
social-network-has-turned-itself-one-worlds-most-
influential-technology-giants. The Economist.
Eggers, W. D., Hamill, R., and Ali, A. (2013). Data as
the new currency: Government’s role in facilitating
the exchange. http://deloitte.wsj.com/ riskandcompli-
ance/files/2013/11/DataCurrency report.pdf. Deloitte
Review.
Ezrachi, A. and Stucke, M. (2016). Virtual Competition:
The Promise and Perils of the Algorithm-Driven Econ-
omy. Harvard University Press.
Facebook (2017a). Graph API Explorer. https://develo
pers.facebook.com/tools/explorer/. Facebook for de-
velopers.
Facebook (2017b). How News Feed Works.
https://www.facebook.com/help/327131014036297.
Facebook.
FTC (Jan 2017). Cross-device tracking: A federal trade
commission staff report. https://www.ftc.gov/
system/files/documents/reports/cross-device-
tracking-federal-trade-commission-staff-report-
january-2017/ftc cross-device tracking report 1-23-
17.pdf. Technical report, FTC, USA.
Gao, H., Hu, J., Huang, T., Wang, J., and Chen, Y. (2011).
Security issues in online social networks. IEEE Inter-
net Computing, 15(4):56–63.
Guha, S., Cheng, B., and Francis, P. (2011). Privad: Practi-
cal privacy in online advertising. In Proceedings of the
8th USENIX Conference on Networked Systems De-
sign and Implementation, NSDI’11, pages 169–182.
USENIX Association.
Guha, S., Reznichenko, A., Tang, K., Haddadi, H., and
Francis, P. (2009). Serving ads from localhost for per-
formance, privacy, and profit. In In Proc. of the 8th
Workshop on Hot Topics in Networks (HotNets 09).
Halpern, S. (Dec 22, 2016). They have, right
now, another you. http://www.nybooks.com/
articles/2016/12/22/they-have-right-now-another-
you/. The New York Review of Books.
Hardt, M. and Nath, S. (2012). Privacy-aware personal-
ization for mobile advertising. In Proceedings of the
2012 ACM Conference on Computer and Communi-
cations Security, CCS ’12, pages 662–673. ACM.
Hartzog, W. and Rubinstein, I. (2017). The anonymization
debate should be about risk, not perfection. Commun.
of the ACM, 60(5):22–24.
ISOC (2017). Your digital footprint matters.
https://www.internetsociety.org/your-digital-
footprint. Internet Society.
Jagatic, T. N., Johnson, N. A., Jakobsson, M., and Menczer,
F. (2007). Social phishing. Commun. of the ACM,
50(10):94–100.
Juels, A. (2001). Targeted advertising ... and privacy too.
In Proceedings of the 2001 Conference on Topics in
Cryptology: The Cryptographer’s Track at RSA, CT-
RSA 2001, pages 408–424. Springer-Verlag.
Kainda, R., Flechais, I., and Roscoe, A. W. (2010). Security
and usability: Analysis and evaluation. In ARES 2010,
Fifth International Conference on Availability, Reli-
ability and Security, 15-18 February 2010, Krakow,
Poland, pages 275–282.
Kramer, A. D. I., Guillory, J. E., and Hancock, J. T. (2014).
Experimental evidence of massive-scale emotional
contagion through social networks. Proceedings of the
National Academy of Sciences, 111(24):8788–8790.
Lee, R., Nia, R., Hsu, J., Levitt, K. N., Rowe, J., Wu, S. F.,
and Ye, S. (2011). Design & implementation of faith,
an experimental system to intercept and manipulate
online social informatics. In Int. Conf. on Advances in
Social Networks Analysis & Mining, pages 195–202.
Lee, S., Wong, E. L., Goel, D., Dahlin, M., and Shmatikov,
V. (2013). πbox: A platform for privacy-preserving
apps. In Proceedings of the 10th USENIX Symposium
on Networked Systems Design and Implementation,
NSDI 2013, pages 501–514.
linkfluence (2017). Social media intelligence for brands and
agencies. https://linkfluence.com/en/.
Liu, Y., Gummadi, K. P., Krishnamurthy, B., and Mislove,
A. (2011). Analyzing facebook privacy settings: User
expectations vs. reality. In Proceedings of the 2011
ACM SIGCOMM Conference on Internet Measure-
ment Conference, IMC ’11, pages 61–70. ACM.
Manjoo, F. (Apr 5, 2017). The online ad indus-
try is undergoing self-reflection. that’s good news.
https://nyti.ms/2oHtaWc. New York Times.
Marshall, C. C. and Shipman, F. M. (2017). Who owns the
social web? Commun. of the ACM, 60(5):52–61.
Narendra K, N. V. and Shyamasundar, R. K. (2016). De-
centralized information flow securing method and sys-
tem for multilevel security and privacy domains. US
Patent 9,507,929.
Narendra, N. V. and Shyamasundar, R. K. (2017). A Com-
plete Generative Label Model for Lattice-based Ac-
cess Control Models. In The 15th International Con-
ference on Software Engineering and Formal Meth-
ods, SEFM 2017, Trento, Italy, September 4-8, 2017,
pages xx–xx. Springer.
O’Neil, C. (2016). Weapons of Math Destruction: How Big
Data Increases Inequality and Threatens Democracy.
Crown/Archetype.
Patil, V. T. and Shyamasundar, R. K. (2017). Undoing of
Privacy Policies on Facebook. In Proceedings of 31
st
Annual IFIP WG 11.3 Conference on Data and Appli-
cations Security and Privacy (DBSec 2017), 10359,
pages xx–xx. Springer.
Popper, N. (March 23, 2017). Banks and tech firms
battle over something akin to gold: Your data.
https://nyti.ms/2mTx7ov. New York Times.
SECRYPT 2017 - 14th International Conference on Security and Cryptography
594