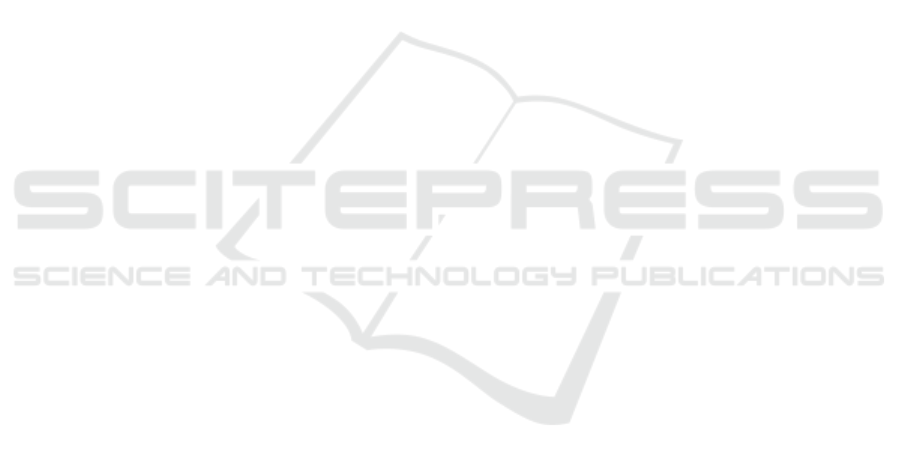
Donoho, D. L. (1995). De-noising by soft-thresholding.
IEEE transactions on information theory, 41(3):613–
627.
Du, W., Tian, X., and Sun, Y. (2011). A dynamic threshold
edge-preserving smoothing segmentation algorithm for
anterior chamber oct images based on modified his-
togram. In 4th International Congress on Image and
Signal Processing (CISP), volume 2, pages 1123–1126.
IEEE.
Gonzalez, R. C. and Woods, R. E. (2002). Digital image
processing. Pearson Prentice Hall.
Gu, S., Zhang, L., Zuo, W., and Feng, X. (2014). Weighted
nuclear norm minimization with application to image
denoising. In Proceedings of the IEEE Conference
on Computer Vision and Pattern Recognition, pages
2862–2869.
Lev, A., Zucker, S. W., and Rosenfeld, A. (1977). Iterative
enhancemnent of noisy images. IEEE Transactions on
Systems, Man, and Cybernetics, 7(6):435–442.
Lin, T.-Y., Maire, M., Belongie, S., Hays, J., Perona, P.,
Ramanan, D., Doll
´
ar, P., and Zitnick, C. L. (2014).
Microsoft coco: Common objects in context. In Euro-
pean Conference on Computer Vision, pages 740–755.
Springer.
Lindenbaum, M., Fischer, M., and Bruckstein, A. (1994). On
gabor’s contribution to image enhancement. Pattern
Recognition, 27(1):1–8.
Mairal, J., Bach, F., Ponce, J., Sapiro, G., and Zisserman, A.
(2009). Non-local sparse models for image restoration.
In IEEE 12th International Conference on Computer
Vision, pages 2272–2279. IEEE.
Muneyasu, M., Maeda, T., Yako, T., and Hinamoto, T.
(1995). A realization of edge-preserving smoothing
filters using layered neural networks. In IEEE Inter-
national Conference on Neural Networks, volume 4,
pages 1903–1906. IEEE.
Pandey, M., Bhatia, M., and Bansal, A. (2016). An ana-
tomization of noise removal techniques on medical
images. In International Conference on Innovation
and Challenges in Cyber Security (ICICCS-INBUSH),
pages 224–229.
Perona, P. and Malik, J. (1990). Scale-space and edge
detection using anisotropic diffusion. IEEE Tran-
sactions on pattern analysis and machine intelligence,
12(7):629–639.
Reich, S., Abramov, A., Papon, J., W
¨
org
¨
otter, F., and Dellen,
B. (2013). A novel real-time edge-preserving smoo-
thing filter. In International Conference on Computer
Vision Theory and Applications, pages 5 – 14.
Rudin, L. I., Osher, S., and Fatemi, E. (1992). Nonlinear
total variation based noise removal algorithms. Physica
D: Nonlinear Phenomena, 60(1):259–268.
Shao, L., Yan, R., Li, X., and Liu, Y. (2014). From heuristic
optimization to dictionary learning: A review and com-
prehensive comparison of image denoising algorithms.
IEEE Transactions on Cybernetics, 44(7):1001–1013.
Sonka, M., Hlavac, V., and Boyle, R. (2014). Image proces-
sing, analysis, and machine vision. Cengage Learning.
Tomasi, C. and Manduchi, R. (1998). Bilateral filtering for
gray and color images. In Sixth International Confe-
rence on Computer Vision, pages 839–846. IEEE.
Yang, Q., Wang, S., and Ahuja, N. (2010). Svm for edge-
preserving filtering. In IEEE Conference on Computer
Vision and Pattern Recognition (CVPR), pages 1775–
1782. IEEE.
Zoran, D. and Weiss, Y. (2011). From learning models of
natural image patches to whole image restoration. In
IEEE International Conference on Computer Vision
(ICCV), pages 479–486. IEEE.
VISAPP 2018 - International Conference on Computer Vision Theory and Applications
94