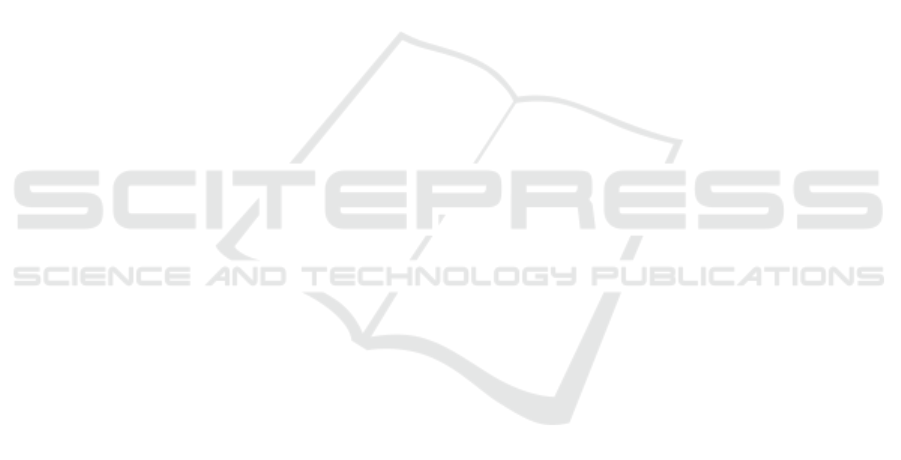
(NBC), Tampere, Finland, June 2017, pages 908–911.
Springer Singapore.
Billman, G. E. (2011). Heart rate variability - A historical
perspective. Frontiers in Physiology, 2.
Choi, J. and Gutierrez-Osuna, R. (2011). Removal of res-
piratory influences from heart rate variability in stress
monitoring. IEEE Sensors Journal, 11(11):2649.
Davari Dolatabadi, A., Khadem, S., and Asl, B. (2017). Au-
tomated diagnosis of coronary artery disease (CAD)
patients using optimized SVM. Computer Methods
and Programs in Biomedicine, 138:117–126.
Gates, K. M., Gatzke-Kopp, L. M., Sandsten, M., and Blan-
don, A. Y. (2015). Estimating time-varying RSA
to examine psychophysiological linkage of marital
dyads. Psychophysiology, 52(8):1059 – 1065.
Grossi, G., Perski, A., Eveng
˚
ard, B., Blomkvist, V., and
Orth-Gom
´
er, K. (2003). Physiological correlates of
burnout among women. Journal of Psychosomatic Re-
search, 55:309 – 316.
Hansen,
˚
A. M., Hogh, A., Persson, R., Karlson, B., Garde,
A. H., and Ørbæk, P. (2006). Original article: Bully-
ing at work, health outcomes, and physiological stress
response. Journal of Psychosomatic Research, 60:63
– 72.
Hansson-Sandsten, M. (2011). Optimal multitaper Wigner
spectrum estimation of a class of locally stationary
processes using Hermite functions. EURASIP Jour-
nal on Advances in Signal Processing, page 980805.
Hernando, A., Lazaro, J., Gil, E., Arza, A., Garzon, J.,
Lopez-Anton, R., De La Camara, C., Laguna, P.,
Aguilo, J., and Bailon, R. (2016). Inclusion of res-
piratory frequency information in heart rate variabil-
ity analysis for stress assessment. IEEE Journal
of Biomedical and Health Informatics, 20(4):1016 –
1025.
J
¨
onsson, P.,
¨
Osterberg, K., Wallerg
˚
ard, M., Hansen,
˚
A. M.,
Garde, A. H., Johansson, G., and Karlson, B. (2015).
Exhaustion-related changes in cardiovascular and cor-
tisol reactivity to acute psychosocial stress. Physiol-
ogy & Behavior, pages 327–337.
Khan, N. A., J
¨
onsson, P., and Sandsten, M. (2017). Per-
formance comparison of time-frequency distributions
for estimation of instantaneous frequency of heart rate
variability signals. Applied Sciences, 7(3).
Lennartsson, A., Jonsdottir, I., and Sj
¨
ors, A. (2016). Low
heart rate variability in patients with clinical burnout.
International Journal of Psychophysiology, 110:171–
178.
Lundgren-Nilsson,
˚
A., Jonsdottir, I. H., Pallant, J., and
Ahlborg, G. (2012). Internal construct validity of
the Shirom-Melamed burnout questionnaire (SMBQ).
BMC Public Health, 12(1).
Melamed, S., Kushnir, T., and Shirom, A. (1992). Burnout
and risk factors for cardiovascular diseases. Behav-
ioral Medicine, 18(2):53 – 60.
Melamed, S., Shirom, A., Toker, S., Berliner, S., and
Shapira, I. (2006). Burnout and risk of cardiovas-
cular disease: Evidence, possible causal paths, and
promising research directions. Psychological Bulletin,
132(3):327 – 353.
Persson, R. and Ørbæk, P. (2003). The influence of per-
sonality traits on neuropsychological test performance
and self-reported health and social context in women.
Personality and Individual Differences, 34:295 – 313.
Persson, R.,
¨
Osterberg, K., Karlson, B., and Ørbæk, P.
(2005). The meta-contrast technique: Relation-
ships with personality traits and cognitive abilities in
healthy women. Scandinavian Journal of Psychology,
46(2):169 – 177.
R Development Core Team (2008). R: A Language and
Environment for Statistical Computing. R Foundation
for Statistical Computing, Vienna, Austria. ISBN 3-
900051-07-0.
Rawlings, J. O., Pantula, S. G., and Dickey, D. A. (1998).
Applied Regression Analysis - A Research Tool. New
York:Springer-Verlag, London, 2nd edition.
RStudio Team (2015). RStudio: Integrated Development
Environment for R. RStudio, Inc., Boston, MA.
Shirom, A. (1989). Burnout in work organization. Interna-
tional Review of Industrial and Organizational Psy-
chology.
Shirom, A. (2003). Job-related burnout: A review. In Hand-
book of occupational health psychology, pages 245 –
264. American Psychological Association.
Silverman, R. (1957). Locally stationary random processes.
IRE Transactions on Information Theory, 3(3):182–
187.
Smith, S. (2005). Covariance, subspace, and intrinsic
Cram
´
er-Rao bounds. IEEE Transactions on Signal
Processing, 53(5):1610 – 1630.
Spielberger, C. D. and Gorsuch, R. L. (1983). Manual for
the state-trait anxiety inventory, STAI (form Y). Palo
Alto, CA, Consulting Psychologists Press.
Voss, A., Schroeder, R., Heitmann, A., Peters, A., and Perz,
S. (2015). Short-term heart rate variability - Influence
of gender and age in healthy subjects. PLoS ONE,
10(3):1 – 33.
Wahlberg, P. and Hansson, M. (2007). Kernels and multiple
windows for estimation of the Wigner-Ville spectrum
of Gaussian locally stationary processes. IEEE Trans-
actions on Signal Processing, 55(1):73 – 84.
Weippert, M., Behrens, K., Rieger, A., Kumar, M., and
Behrens, M. (2015). Effects of breathing patterns and
light exercise on linear and nonlinear heart rate vari-
ability. Applied Physiology Nutrition and Metabolism,
40(8):762 – 768.
Woo, J.-M. and Kim, T.-S. (2015). Gender plays signifi-
cant role in short-term heart rate variability. Applied
Psychophysiology & Biofeedback, 40(4):297 – 303.
Effects of Age, BMI, Anxiety and Stress on the Parameters of a Stochastic Model for Heart Rate Variability Including Respiratory
Information
25