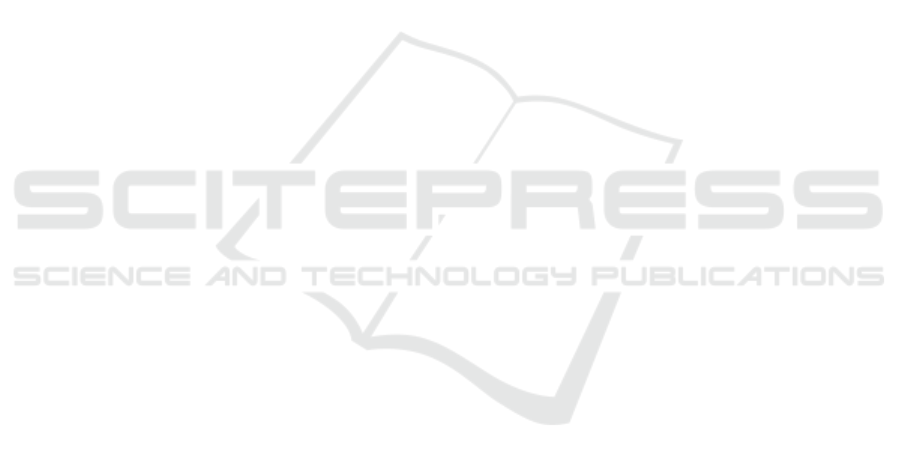
Angle Images. In SIGGRAPH.
Cehovin, L., Lukezic, A., Leonardis, A., and Kristan,
M. (2016). Beyond Standard Benchmarks: Para-
meterizing Performance Evaluation in Visual Object
Tracking.
Corbillon, X., Devlic, A., Simon, G., and Chakareski, J.
(2017). Viewport-Adaptive Navigable 360-degree Vi-
deo Delivery. In SIGGRAPH.
Danelljan, M., Robinson, A., Shahbaz Khan, F., and Fels-
berg, M. (2016). Beyond Correlation Filters: Le-
arning Continuous Convolution Operators for Visual
Tracking. ECCV 2016.
Ferryman, J. and Ellis, A. (2010). PETS2010: Dataset and
Challenge. Proceedings - IEEE International Confe-
rence on Advanced Video and Signal Based Surveil-
lance, AVSS 2010, pages 143–148.
Hare, S., Golodetz, S., Saffari, A., Vineet, V., Cheng,
M. M., Hicks, S. L., and Torr, P. H. S. (2016).
STRUCK: Structured Output Tracking with Kernels.
IEEE Transactions on Pattern Analysis and Machine
Intelligence, 38(10):2096–2109.
Held, D., Thrun, S., and Savarese, S. (2016). Learning to
Track at 100 FPS with Deep Regression Networks.
In Computer Vision and Pattern Recognition (CVPR),
2016 IEEE Conference on.
Henriques, J., Caseiro, R., Martins, P., and Batista, J.
(2014). High-Speed Tracking with Kernelized Cor-
relation Filters. Pattern Analysis and Machine Intelli-
gence, IEEE Transactions on, 37(3):1–14.
Kalal, Z., Mikolajczyk, K., and Matas, J. (2011). Tracking-
Learning-Detection. IEEE Transactions on Pattern
Analysis and Machine Intelligence, 34(7):1409–1422.
Kristan, M., Leonardis, A., Matas, J., Felsberg, M., Pflug-
felder, R., Cehovin, L., and Vojir, T. e. a. (2016a). The
Visual Object Tracking VOT2016 Challenge Results.
ECCV 2016 Workshops, pages 777–823.
Kristan, M., Matas, J., Leonardis, A., Felsberg, M., and Ce-
hovin, L. e. a. (2015). The Visual Object Tracking
VOT2015 Challenge Results. ICCV Workshop on Vi-
sual Object Tracking Challenge.
Kristan, M., Matas, J., Nebehay, G., Porikli, F., and Ce-
hovin, L. (2016b). A Novel Performance Evalua-
tion Methodology for Single-Target Trackers. IEEE
Transactions on Pattern Analysis and Machine Intel-
ligence, 38(11):2137–2155.
Kristan, M., Pflugfelder, R., Leonardis, A., Matas, J., Ce-
hovin, L., Nebehay, G., and Vojir, T. e. a. (2014). The
Visual Object Tracking VOT2014 Challenge Results.
ECCV Visual Object Tracking Challenge Workshop.
Nam, H. and Han, B. Learning Multi-Domain Convoluti-
onal Neural Networks for Visual Tracking. In Com-
puter Vision and Pattern Recognition (CVPR), 2016
IEEE Conference on.
Russakovsky, O., Deng, J., Su, H., Krause, J., Satheesh,
S., Ma, S., Huang, Z., Karpathy, A., Khosla, A.,
Bernstein, M., Berg, A. C., and Fei-Fei, L. (2015).
ImageNet Large Scale Visual Recognition Challenge.
International Journal of Computer Vision (IJCV),
115(3):211–252.
Smeulders, A. W. M., Chu, D. M., Cucchiara, R., Calde-
rara, S., Dehghan, A., and Shah, M. (2014). Visual
tracking: An experimental survey. IEEE Transacti-
ons on Pattern Analysis and Machine Intelligence,
36(7):1442–1468.
Su, Y.-C. and Grauman, K. (2017). Making 360 Video Wa-
tchable in 2D: Learning Videography for Click Free
Viewing. In Computer Vision and Pattern Recogni-
tion (CVPR), 2017 IEEE Conference on.
Su, Y.-C., Jayaraman, D., and Grauman, K. (2016).
Pano2Vid: Automatic Cinematography for Watching
360 Videos. In ACCV.
Vedaldi, A. and Lenc, K. (2015). MatConvNet – Convolu-
tional Neural Networks for MATLAB. In Proceeding
of the ACM Int. Conf. on Multimedia.
Wu, Y., Lim, J., and Yang, M. H. Online Object Tracking:
A Benchmark. In Computer Vision and Pattern Re-
cognition (CVPR), 2013 IEEE Conference on.
Xiao, J., Ehinger, K. A., Oliva, A., and Torralba, A. Recog-
nizing scene viewpoint using panoramic place repre-
sentation. Computer Vision and Pattern Recognition
(CVPR), 2012 IEEE Conference on.
VISAPP 2018 - International Conference on Computer Vision Theory and Applications
32