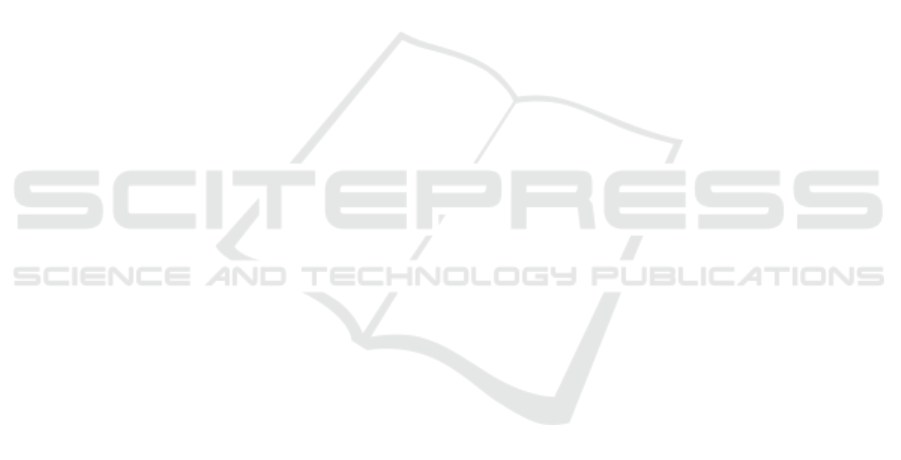
Gu, C., Arbelaez, P., Lin, Y., Yu, K., and Malik, J. (2012).
Multi-component models for object detection. In
ECCV.
Hillel, A. and Weinshall, D. (2006). Subordinate class re-
cognition using relational object models. In NIPS.
Isola, P., Lim, J., and Adelson, E. (2015). Discovering states
and transformations in image collections. In CVPR.
Johnson, J., Krishna, R., Stark, M., Li, L.-J., Shamma, D.,
Bernstein, M., and Fei-Fei, L. (2015). Image retrieval
using scene graphs. In CVPR.
Kim, G., Faloutsos, C., and Hebert, M. (2008). Unsupervi-
sed modeling of object categories using link analysis
techniques. In CVPR.
Krause, J., Jin, H., Yang, J., and Fei-Fei, L. (2015).
Fine-grained recognition without part annotations. In
CVPR.
Li, X., Snoek, G., Worring, M., D.Koelma, and Smeulders,
A. (2013). Bootstrapping visual categorization with
relevant negatives. IEEE Trans. on Multimedia, 15(4).
Malisiewicz, T., Gupta, A., and Efros, A. (2011). Ensemble
of exemplar-SVMs for object detection and beyond.
In ICCV.
Ng, A., Jordan, M., and Weiss, Y. (2001). On spectral clus-
tering: Analysis and an algorithm. In NIPS.
Ouyang, W., Wang, X., Zhang, C., and Yang, X. (2016).
Factors in finetuning deep model for object detection
with long-tail distribution. In CVPR.
Philbin, J. and Zisserman, A. (2008). Object mining using
a matching graph on very large image collections. In
Indian Conference on Computer Vision, Graphics and
Image Processing.
Pu, J., Jiang, Y.-G., Wang, J., and Xue, X. (2014). Which
looks like which: Exploring inter-class relationships
in fine-grained visual categorization. In ECCV.
Redmon, J., Divvala, S., Girshick, R., and Farhadi, A.
(2016). You only look once: Unified, real-time ob-
ject detection. In CVPR.
Rematas, K., Fernando, B., Dellaert, F., and Tuytelaars, T.
(2015). Dataset fingerprints: Exploring image col-
lections through data mining. In CVPR.
Ren, S., He, K., Girshick, R., and Sun, J. (2015). Faster R-
CNN: Towards real-time object detection with region
proposal networks. In NIPS.
Rubinstein, M., Liu, C., and Freeman, W. (2016). Joint in-
ference in weakly-annotated image datasets via dense
correspondence. Int J Comp Vis, 119:23–45.
Russakovsky, O., Deng, J., Su, H., Krause, J., Satheesh, S.,
Ma, S., Huang, Z., Karpathy, A., Khosla, A., Bern-
stein, M., Berg, A. C., and Fei-Fei, L. (2015). Image-
Net Large Scale Visual Recognition Challenge. Int J
Comp Vis, 115(3):211–252.
Salakhutdinov, R., Torralba, A., and Tenenbaum, J. (2011).
Learning to share visual appearance for multiclass ob-
ject detection. In CVPR.
Shokrollahi Yancheshmeh, F., Chen, K., and K
¨
am
¨
ar
¨
ainen,
J.-K. (2015). Unsupervised visual alignment with si-
milarity graphs. In CVPR.
Wan, L., Eigen, D., and Fergus, R. (2015). End-to-end inte-
gration of a convolutional network, deformable parts
model and non-maximum suppression. In CVPR.
Yu, A. and Grauman, K. (2014). Predicting useful neig-
hborhoods for lazy local learning. In NIPS.
Zelnik-Manor, L. and Perona, P. (2004). Self-tuning
spectral clustering. In NIPS.
Zhou, T., Lee, Y., Yu, S., and Efros, A. (2015). Flow-
Web: Joint image set alignment by weaving consis-
tent, pixel-wise correspondences. In CVPR.
Zhu, X., Anguelov, D., and Ramanan, D. (2014). Captu-
ring long-tail distributions of object subcategories. In
CVPR.
Hierarchical Deformable Part Models for Heads and Tails
53