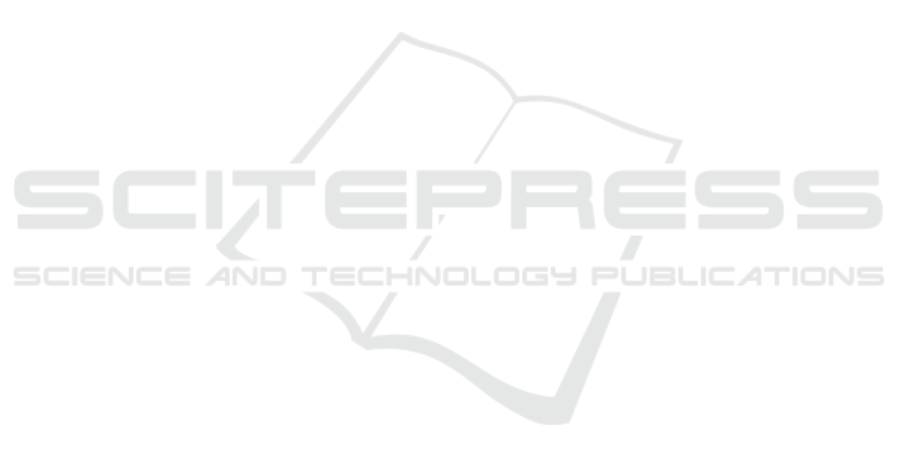
performance further.
Since histological verification was only present
for cancerous areas in both data sets, we can only as-
sume that clinically normal regions represent healthy
epithelium. Extraction of tissue from those regions
would however be ethically questionable and not re-
ceive approval of the review boards.
It is questionable, if an 100% accurate classifica-
tion of epithelial tissue is possible using CLE alone,
as even experts in the field of CLE were not able to
classify cancerous tissue perfectly (Goncalves et al.,
2017; Oetter et al., 2016). Due to the low penetration
depth of CLE, it is sometimes possible to overlook tu-
mors that spread within the submucosa. Such tumors
could be visualized only through histological section
or perhaps through Optical Coherence Tomography
(Betz et al., 2015).
One important aspect in automated inspection of
CLE images is the removal of artifact-tainted images
prior to training, since artifact occurrence is corre-
lated with the surface conditions of the epithelium.
This implies that it is also correlated to the malig-
nancy classification, a causal relationship between
artifact prevalence and tissue classification should,
however, be neglected. This step was done manu-
ally in this work and this problem is subject of future
work.
Even though our approach found good accuracy
ratings, generalization can not be claimed to be fully
shown with this study, due to the limited amount of
patient data. Because of this, future work of our re-
search group will concentrate on the acquisition of
imaging data in order to increase the variance in the
data set, which will presumably increase performance
and robustness of the algorithmic approach.
6 SUMMARY
In this work, we have shown the principal ability to
generalize patch-based CLE image classification with
convolutional networks of potentially cancerous ep-
ithelium from a more diversified data set (from the
oral cavity) to one of another anatomical location (the
vocal folds) with less variance. The second data set
was from a different clinic and a different team.
The generalization showed very promising re-
sults and concatenation of both sets did show fur-
ther improvements in a leave-one-patient-out cross-
validation scenario.
In total, we achieved an accuracy of 89.45% in
the generalization task, where the classification model
was trained on the oral cavity data set and applied on
the vocal cords data set. For the concatenated data
set with 17 patients, we achieved a total accuracy of
90.81% for the complete data set.
REFERENCES
Aubreville, M., Knipfer, C., Oetter, N., Jaremenko, C., Rod-
ner, E., Denzler, J., Bohr, C., Neumann, H., Stelzle,
F., and Maier, A. K. (2017). Automatic classification
of cancerous tissue in laserendomicroscopy images of
the oral cavity using deep learning. Scientific Reports
7:11979.
Betz, C. S., Kraft, M., Arens, C., Schuster, M., Pfef-
fer, C., R
¨
uhm, A., Stepp, H., Englhard, A., and
Volgger, V. (2015). Optische Diagnoseverfahren
zur Tumorfr
¨
uhdiagnostik im oberen Luft-Speise-Weg.
HNO, 64(1):41–48.
Chauhan, S. S., Dayyeh, B. K. A., Bhat, Y. M., Gottlieb,
K. T., Hwang, J. H., Komanduri, S., Konda, V., Lo,
S. K., Manfredi, M. A., Maple, J. T., et al. (2014).
Confocal laser endomicroscopy. Gastrointestinal en-
doscopy, 80(6):928–938.
Cikojevi
´
c, D., Glun
ˇ
ci
´
c, I., and Pe
ˇ
suti
´
c-Pisac, V. (2008).
Comparison of contact endoscopy and frozen section
histopathology in the intra-operative diagnosis of la-
ryngeal pathology. The Journal of Laryngology &
Otology, 122(8):836–839.
Dittberner, A., Rodner, E., Ortmann, W., Stadler, J.,
Schmidt, C., Petersen, I., Stallmach, A., Denzler, J.,
and Guntinas-Lichius, O. (2016). Automated analy-
sis of confocal laser endomicroscopy images to detect
head and neck cancer. Head & Neck, 38(S1):E1419–
E1426.
Ferlay, J., Soerjomataram, I., Dikshit, R., Eser, S., Mathers,
C., Rebelo, M., Parkin, D. M., Forman, D., and Bray,
F. (2014). Cancer incidence and mortality worldwide:
Sources, methods and major patterns in GLOBOCAN
2012. International Journal of Cancer, 136(5):E359–
E386.
Forastiere, A., Koch, W., Trotti, A., and Sidransky, D.
(2001). Head and Neck Cancer. The New England
Journal of Medicine, 345(26):1890–1900.
Goncalves, M., Iro, H., Dittberner, A., Agaimy, A., and
Bohr, C. (2017). Value of confocal laser endomi-
croscopy in the diagnosis of vocal cord lesions. Eu-
ropean Review for Medical and Pharmacological Sci-
ences, 21(18):3990-3997.
Jaremenko, C., Maier, A., Steidl, S., Hornegger, J., Oet-
ter, N., Knipfer, C., Stelzle, F., and Neumann, H.
(2015). Classification of Confocal Laser Endomicro-
scopic Images of the Oral Cavity to Distinguish Patho-
logical from Healthy Tissue. In Bildverarbeitung f
¨
ur
die Medizin 2015, pages 479–485. Springer Berlin
Heidelberg.
Luellmann-Rauch, R. (2015). Taschenbuch Histologie.
Thieme, Stuttgart u.a.
Muto, M., Nakane, M., Katada, C., Sano, Y., Ohtsu, A.,
Esumi, H., Ebihara, S., and Yoshida, S. (2004). Squa-
mous cell carcinoma in situ at oropharyngeal and hy-
Patch-based Carcinoma Detection on Confocal Laser Endomicroscopy Images - A Cross-site Robustness Assessment
33