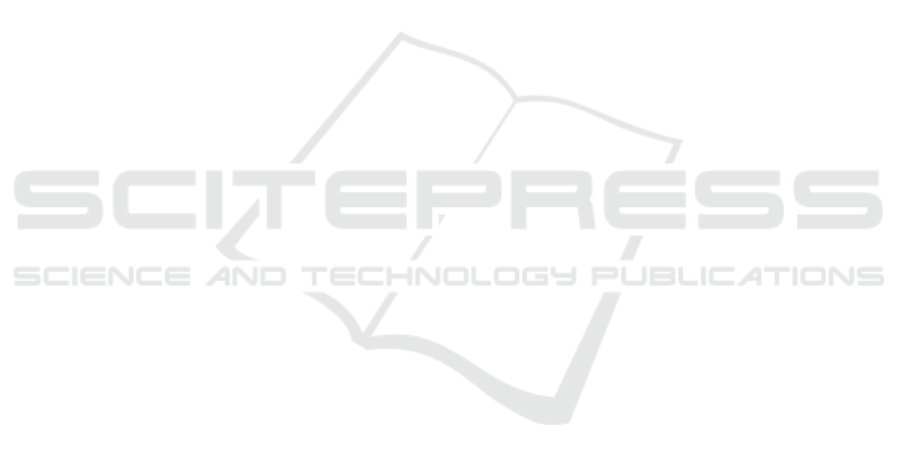
REFERENCES
Aggelopoulou, A., Bochtis, D., Fountas, S., Swain, K. C.,
Gemtos, T., and Nanos, G. (2011). Yield prediction in
apple orchards based on image processing. Precision
Agriculture, 12(3):448–456.
Barandela, R., S
´
anchez, J. S., Garcıa, V., and Rangel, E.
(2003). Strategies for learning in class imbalance pro-
blems. Pattern Recognition, 36(3):849–851.
Bishop, C. M. (2006). Pattern recognition and machine le-
arning. springer.
Brunelli, R. (2009). Template matching techniques in com-
puter vision: theory and practice. John Wiley & Sons.
Di Martino, M., Decia, F., Molinelli, J., and Fern
´
andez, A.
(2012). Improving electric fraud detection using class
imbalance strategies. In International Conference on
Pattern Recognition and Methods, 1st. ICPRAM., pa-
ges 135–141.
Di Martino, M., Fern
´
andez, A., Iturralde, P., and Le-
cumberry, F. (2013a). Novel classifier scheme for
imbalanced problems. Pattern Recognition Letters,
34(10):1146–1151.
Di Martino, M., Hern
´
andez, G., Fiori, M., and Fern
´
andez,
A. (2013b). A new framework for optimal classifier
design. Pattern Recognition, 46(8):2249–2255.
Dougherty, E. R. and Lotufo, R. A. (2003). Hands-on mor-
phological image processing, volume 59. SPIE press.
Garc
´
ıa, V., S
´
anchez, J., and Mollineda, R. (2012). On
the suitability of numerical performance measures for
class imbalance problems. International Conference
In Pattern Recognition Aplications and Methods, pa-
ges 310–313.
Gongal, A., Silwal, A., Amatya, S., Karkee, M., Zhang, Q.,
and Lewis, K. (2016a). Apple crop-load estimation
with over-the-row machine vision system. Computers
and Electronics in Agriculture, 120:26–35.
Gongal, A., Silwal, A., Amatya, S., Karkee, M., Zhang, Q.,
and Lewis, K. (2016b). Apple crop-load estimation
with over-the-row machine vision system. Computers
and Electronics in Agriculture, 120:26–35.
Hearst, M. A., Dumais, S. T., Osuna, E., Platt, J., and Schol-
kopf, B. (1998). Support vector machines. IEEE In-
telligent Systems and their Applications, 13(4):18–28.
Japkowicz, N. and Stephen, S. (2002). The class imbalance
problem: A systematic study. Intelligent data analy-
sis, 6(5):429–449.
Ji, W., Zhao, D., Cheng, F., Xu, B., Zhang, Y., and Wang, J.
(2012). Automatic recognition vision system guided
for apple harvesting robot. Computers & Electrical
Engineering, 38(5):1186–1195.
Jim
´
enez, A. R., Jain, A. K., Ceres, R., and Pons, J. (1999).
Automatic fruit recognition: a survey and new results
using range/attenuation images. Pattern recognition,
32(10):1719–1736.
Kubat, M., Matwin, S., et al. (1997). Addressing the curse
of imbalanced training sets: one-sided selection. In
ICML, volume 97, pages 179–186. Nashville, USA.
Kuncheva, L. I. (2004). Combining pattern classifiers: met-
hods and algorithms. John Wiley & Sons.
Linker, R., Cohen, O., and Naor, A. (2012). Determination
of the number of green apples in rgb images recorded
in orchards. Computers and Electronics in Agricul-
ture, 81:45–57.
Liu, X.-Y., Wu, J., and Zhou, Z.-H. (2009). Exploratory un-
dersampling for class-imbalance learning. IEEE Tran-
sactions on Systems, Man, and Cybernetics, Part B
(Cybernetics), 39(2):539–550.
Manjunath, B. S., Ohm, J.-R., Vasudevan, V. V., and Ya-
mada, A. (2001). Color and texture descriptors. IEEE
Transactions on circuits and systems for video techno-
logy, 11(6):703–715.
Marzoa, C. (2017a). Apple data base. Website. last chec-
ked: 17.07.2017.
Marzoa, C. (2017b). Web of the apple detection proyect.
Website. last checked: 17.07.2017.
Muja, M. and Lowe, D. G. (2009). Fast approximate nearest
neighbors with automatic algorithm configuration. VI-
SAPP (1), 2(331-340):2.
OpenCV (2017). Opencv. Website. last checked:
17.07.2017.
Rakun, J., Stajnko, D., and Zazula, D. (2011). Detecting
fruits in natural scenes by using spatial-frequency ba-
sed texture analysis and multiview geometry. Compu-
ters and Electronics in Agriculture, 76(1):80–88.
Robinson, T. (2006). The evolution towards more competi-
tive apple orchard systems in the usa. In XXVII Inter-
national Horticultural Congress-IHC2006: Internati-
onal Symposium on Enhancing Economic and Envi-
ronmental 772, pages 491–500.
Safren, O., Alchanatis, V., Ostrovsky, V., and Levi, O.
(2007). Detection of green apples in hyperspectral
images of apple-tree foliage using machine vision.
Transactions of the ASABE, 50(6):2303–2313.
Scholkopf, B. and Smola, A. J. (2001). Learning with ker-
nels: support vector machines, regularization, optimi-
zation, and beyond. MIT press.
Stajnko, D., Lakota, M., and Ho
ˇ
cevar, M. (2004). Estima-
tion of number and diameter of apple fruits in an or-
chard during the growing season by thermal imaging.
Computers and Electronics in Agriculture, 42(1):31–
42.
Szeliski, R. (2010). Computer vision: algorithms and ap-
plications. Springer Science & Business Media.
Tabb, A. L., Peterson, D. L., and Park, J. (2006). Segmen-
tation of apple fruit from video via background mo-
deling. In 2006 ASAE Annual Meeting, page 1. Ameri-
can Society of Agricultural and Biological Engineers.
Wang, Q., Nuske, S., Bergerman, M., and Singh, S. (2013).
Automated crop yield estimation for apple orchards.
In Experimental robotics, pages 745–758. Springer.
Zhao, J., Tow, J., and Katupitiya, J. (2005). On-tree fruit
recognition using texture properties and color data.
In Intelligent Robots and Systems, 2005.(IROS 2005).
2005 IEEE/RSJ International Conference on, pages
263–268. IEEE.
Computer Vision based System for Apple Detection in Crops
249