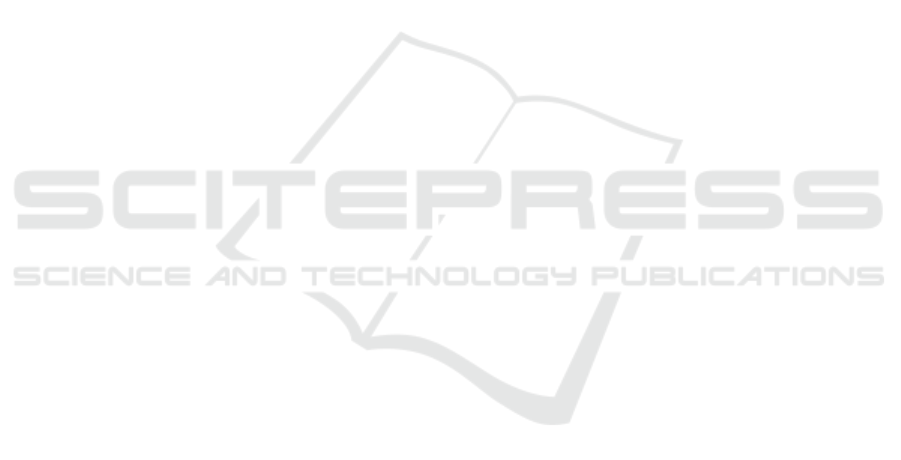
several analyses to explain the accuracy improve-
ments and our current hypothesis is that the complex-
ity analysis on the ECG signals provides enough in-
formation for accurate BP class estimation. If that is
indeed so, future BP measurements may be performed
using only an ECG sensor. However, to completely
confirm this hypothesis, our method should be evalu-
ated on a bigger dataset with a leave-one-subject-out
evaluation technique, including the publicly available
physiological signals from the Physionet databases
(Goldberger et al., 2000).
Our future work is towards the collection of ECG
signals encompassing various ECG sensors and dif-
ferent target groups, since the research community is
missing this kind of data. The goal is to create a bal-
anced database, covering the critical BP classes (e.g.,
hypertensive crisis), and to develop sensor indepen-
dent methodology for BP estimation. Finally, we plan
to improve the methodology to be able to estimate the
real SBP and DBP values (e.g., using regression tech-
niques), and thus to contribute to the ”single-sensor
fits all” paradigm of using as least equipment to de-
rive as much vital signs as possible.
ACKNOWLEDGEMENTS
This research is supported by SIARS, NATO multi-
year project NATO.EAP.SFPP 984753.
REFERENCES
AHA (2016). Understanding blood pressure readings.
Alvarez, J. M. (2015). Higuchi and katz fractal dimension
measures.
Armstrong, T. (2008). The Human Odyssey: Navigating the
Twelve Stages of Life. Sterling, New York.
Bhattacharya, J. et al. (2000). Complexity analysis of
spontaneous eeg. Acta neurobiologiae experimentalis,
60(4):495–502.
Bittium Biosignals (2016). emotion faros.
Cancio, L. C., Batchinsky, A. I., Salinas, J., Kuusela,
T., Convertino, V. A., Wade, C. E., and Holcomb,
J. B. (2008). Heart-rate complexity for prediction
of prehospital lifesaving interventions in trauma pa-
tients. Journal of Trauma and Acute Care Surgery,
65(4):813–819.
Cooking Hacks (2016). e-health sensor platform v2. 0 for
arduino and raspberry pi [biometric/medical applica-
tions].
Cosoli, G., Casacanditella, L., Pietroni, F., Calvaresi, A.,
Revel, G., and Scalise, L. (2015). A novel approach
for features extraction in physiological signals. In
Medical Measurements and Applications (MeMeA),
2015 IEEE International Symposium on, pages 380–
385. IEEE.
Costa, M., Goldberger, A. L., and Peng, C.-K. (2005). Mul-
tiscale entropy analysis of biological signals. Physical
review E, 71(2):021906.
Doyle, T. L., Dugan, E. L., Humphries, B., and Newton,
R. U. (2004). Discriminating between elderly and
young using a fractal dimension analysis of centre of
pressure. Int J Med Sci, 1(1):11–20.
Eke, A., Herman, P., Kocsis, L., and Kozak, L. (2002). Frac-
tal characterization of complexity in temporal physio-
logical signals. Physiological measurement, 23(1):R1.
Goldberger, A. L., Amaral, L. A., Glass, L., Hausdorff,
J. M., Ivanov, P. C., Mark, R. G., Mietus, J. E., Moody,
G. B., Peng, C.-K., and Stanley, H. E. (2000). Phys-
iobank, physiotoolkit, and physionet. Circulation,
101(23):e215–e220.
Goli, S. and T., J. (2014). Cuff less continuous non-invasive
blood pressure measurement using pulse transit time
measurement. International Journal of Recent Devel-
opment in Engineering and Technology, 2(1):86–91.
G
´
omez, C., Hornero, R., Ab
´
asolo, D., Fern
´
andez, A., and
L
´
opez, M. (2006). Complexity analysis of the magne-
toencephalogram background activity in alzheimer’s
disease patients. Medical engineering & physics,
28(9):851–859.
Grenwis, J., Bogie, H., and Main, B. (2012). A chronic
method for measuring real-time pulse wave veloc-
ity in conscious rodents. The FASEB Journal, 26(1
Supplement):870–10.
Hall, M., Frank, E., Holmes, G., Pfahringer, B., Reutemann,
P., and Witten, I. H. (2009). The weka data min-
ing software: an update. ACM SIGKDD explorations
newsletter, 11(1):10–18.
Hassan, M. K. B. A., Mashor, M., Nasir, N. M., and Mo-
hamed, S. (2008). Measuring of systolic blood pres-
sure based on heart rate. In 4th Kuala Lumpur Interna-
tional Conference on Biomedical Engineering 2008,
pages 595–598. Springer.
Heart and Vascular Team (2016). Busting 6 myths about
blood pressure and heart rate.
i Car
´
os, J. M. S. (2011). Continuous non-invasive
blood pressure estimation. PhD thesis, Universitat
Polit
`
ecnica de Catalunya.
Kugiumtzis, D. and Tsimpiris, A. (2010). Measures of anal-
ysis of time series (mats): A matlab toolkit for compu-
tation of multiple measures on time series data bases.
arXiv preprint arXiv:1002.1940.
Labadin, J. and Ahmadi, A. (2006). Mathematical mod-
eling of the arterial blood flow. In Proceedings
of the 2nd IMT-GT Regional Conference on Mathe-
matics, Statistics and Applications, Universiti Sains
Malaysia, Penang.
Lehocki, F., Kossaczky, I., Homola, M., Skalicky, D., My-
dliar, M., and Thurzo, A. (2014). Yet another hyper-
tension telehealth solution? the rules will tell you.
In Biomedical Engineering and Sciences (IECBES),
2014 IEEE Conference on, pages 510–515. IEEE.
McBride, J. C., Zhao, X., Munro, N. B., Smith, C. D.,
Jicha, G. A., Hively, L., Broster, L. S., Schmitt, F. A.,
ECG-derived Blood Pressure Classification using Complexity Analysis-based Machine Learning
291