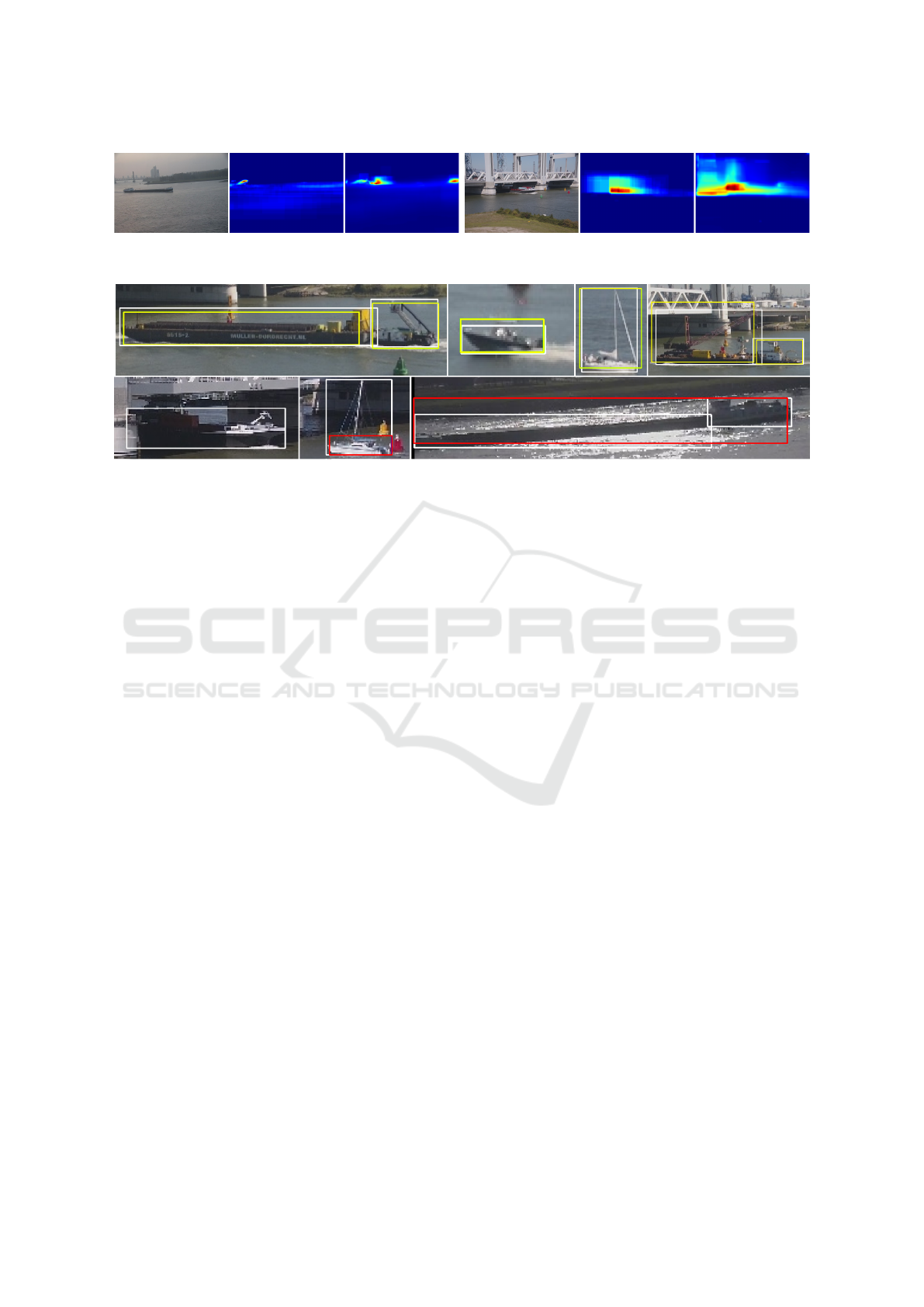
VP 2
VP 8
Missed
Missed
Incorrect
Incorrect
Figure 6: Heatmaps for missed objects and incorrect detections. Note that each heatmap is individually scaled, so colors are
not directly comparable. The two cases are taken from critical camera viewpoints, pointing to difficult conditions.
Figure 7: Examples of correct detections (top rows) and incorrect/missed detections (bottom rows). Correct detections are
shown in yellow, incorrect detections in red and ground-truth annotations in white.
ries, incorrect merging of multiple ships and the influ-
ence of surrounding infrastructure like bridges.
The SSD detector trained on the proposed surveil-
lance dataset significantly outperforms the detector
trained on the PASCAL and COCO datasets. This
shows that the dataset statistics for the commonly
used generic object detection datasets are quite dif-
ferent from our real-life surveillance dataset, specifi-
cally dedicated to harbours and ships.
The obtained performance and robustness of the
developed ship detector proves to be valuable for sur-
veillance in harbour infrastructure, where radar is al-
ready used. The location of the detected vessels is
complementing the positioning information of the ra-
dar system, leading to a higher accuracy of the Vessel
Tracking System (VTS). Moreover, the use of a ca-
mera enables visual feedback on details of the ships
and provides the operator with a visual cue about the
considered vessels.
REFERENCES
Arshad, N., Moon, K.-S., and Kim, J.-N. (2010). Multiple
ship detection and tracking using background registra-
tion and morphological operations. In Signal Proces-
sing and Multimedia, pages 121–126. Springer.
Bao, X., Javanbakhti, S., Zinger, S., Wijnhoven, R., and
de With, P. H. N. (2013). Context modeling combined
with motion analysis for moving ship detection in port
surveillance. Journal of Electronic Imaging, 22(4).
Brouwers, G. M. Y. E., Zwemer, M. H., Wijnhoven, R. G. J.,
and de With, P. H. N. (2016). Automatic calibration of
stationary surveillance cameras in the wild. In Proc.
ECCV 2016 Workshops, pages 743–759. Springer.
Everingham, M. et al. (2007). The PASCAL Visual Object
Classes Challenge 2007 (VOC2007) Results.
Everingham, M. et al. (2012). The PASCAL Visual Object
Classes Challenge 2012 (VOC2012) Results.
Girshick, R. (2015). Fast R-CNN. In ICCV.
Girshick, R., Donahue, J., Darrell, T., and Malik, J. (2014).
Rich feature hierarchies for accurate object detection
and semantic segmentation. In Proc. IEEE CVPR.
Hoiem, D., Chodpathumwan, Y., and Dai, Q. (2012). Diag-
nosing Error in Object Detectors, pages 340–353.
Hu, W.-C., Yang, C.-Y., and Huang, D.-Y. (2011). Ro-
bust real-time ship detection and tracking for visual
surveillance of cage aquaculture. Journal of Visual
Comm. and Image Representation, 22(6):543–556.
Lin, T. et al. (2014). Microsoft COCO: common objects in
context. CoRR, abs/1405.0312.
Liu, W., Anguelov, D., Erhan, D., Szegedy, C., Reed, S.,
Fu, C.-Y., and Berg, A. C. (2016). Ssd: Single shot
multibox detector. In ECCV, pages 21–37. Springer.
Redmon, J., Divvala, S. K., Girshick, R. B., and Farhadi, A.
(2015). You only look once: Unified, real-time object
detection. CoRR, abs/1506.02640.
Russakovsky, O. et al. (2015). ImageNet Large Scale Visual
Recognition Challenge. IJCV, 115(3):211–252.
Shi, J. and Tomasi, C. (1994). Good features to track. In
1994 Proceedings of IEEE CVPR, pages 593–600.
Simonyan, K. and Zisserman, A. (2014). Very deep convo-
lutional networks for large-scale image recognition.
Uijlings, J. R., Van De Sande, K. E., Gevers, T., and Smeul-
ders, A. W. (2013). Selective search for object recog-
nition. Int. journal of comp. vision, 104(2):154–171.
Wijnhoven, R., Van Rens, K., Jaspers, E. G., and de With,
P. H. (2010). Online learning for ship detection in
maritime surveillance. In Proc. of 31th Symposium on
Information Theory in the Benelux, pages 73–80.
VISAPP 2018 - International Conference on Computer Vision Theory and Applications
160