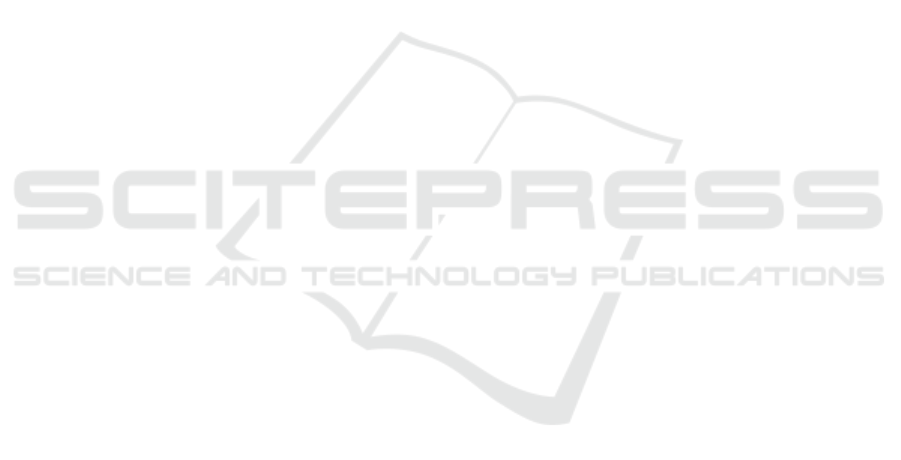
results of the Hybrid Tree may improve over time
when the size of the dataset increases (Kohavi, 1996).
Due to the nature of the problem the results do not
present very high predicting power. Nevertheless,
comparing this study with results from the state of the
art, the obtained results are satisfactory.
In the future, several actions are planned. First, the
presented classifiers will be trained with larger
amount of data as new patients are included into the
study. In parallel, the ambulatory patient monitored
data will be studied to determine whether the
presented predictive models could be improved.
Next, we aim to build an integrated telemonitoring
system that integrates these predictive models to
support both clinicians, to manage best the patients,
and patients, to empower them in their disease
management and prevent potential decompensations.
Finally, this system will be tested in a trial study to
determine its usability.
REFERENCES
Ponikowski, P., Voors, A. A., Anker, S. D. et al (2016).
2016 ESC Guidelines for the diagnosis and treatment of
acute and chronic heart failure: The Task Force for the
diagnosis and treatment of acute and chronic heart
failure of the European Society of Cardiology (ESC)
Developed with the special contribution of the Heart
Failure Association (HFA) of the ESC. European heart
journal, 37(27), 2129-2200.
Maggioni, A. P., Orso, F., Calabria, S. el al (2016). The
real‐world evidence of heart failure: findings from 41
413 patients of the ARNO database. European journal
of heart failure, 18(4), 402-410.
Mortazavi, B. J., Downing, N. S., Bucholz, E. M. et al
(2016). Analysis of machine learning techniques for
heart failure readmissions. Circulation:
Cardiovascular Quality and Outcomes,
CIRCOUTCOMES-116.
Zolfaghar, K., Meadem, N., Teredesai, A., Roy, S. B. et al
(2013, October). Big data solutions for predicting risk-
of-readmission for congestive heart failure patients. In
Big Data, 2013 IEEE International Conference on (pp.
64-71). IEEE.
Zheng, B., Zhang, J., Yoon, S. W. et al (2015). Predictive
modeling of hospital readmissions using metaheuristics
and data mining. Expert Systems with Applications,
42(20), 7110-7120.
Meadem, N., Verbiest, N., Zolfaghar, K. et al (2013).
Exploring preprocessing techniques for prediction of
risk of readmission for congestive heart failure patients.
In Data Mining and Healthcare (DMH), at
International Conference on Knowledge Discovery and
Data Mining (KDD) (Vol. 150).
Krumholz, H. M., Chen, Y. T., Wang, Y. et al (2000).
Predictors of readmission among elderly survivors of
admission with heart failure. American heart journal,
139(1), 72-77.
Amarasingham, R., Moore, B. J., Tabak, Y. P. et al (2010).
An automated model to identify heart failure patients at
risk for 30-day readmission or death using electronic
medical record data. Medical care, 48(11), 981-988.
Sudhakar, S., Zhang, W., Kuo, Y. F. et al (2015). Validation
of the readmission risk score in heart failure patients at
a tertiary hospital. Journal of cardiac failure, 21(11),
885-891.
Artetxe, A., Larburu, N., Murga, N. et al (2017, June). Heart
Failure Readmission or Early Death Risk Factor
Analysis: A Case Study in a Telemonitoring Program.
In International Conference on Innovation in Medicine
and Healthcare (pp. 244-253). Springer, Cham.
Ward Jr, J. H. (1963). Hierarchical grouping to optimize an
objective function. Journal of the American statistical
association, 58(301), 236-244.
Murphy, K. P. (2006). Naive bayes classifiers. University
of British Columbia.
Vapnik, V. (2013). The nature of statistical learning theory.
Springer science & business media.
Park, C. H., & Lee, M. (2009). A SVM-based discretization
method with application to associative classification.
Expert Systems with Applications, 36(3), 4784-4787.
Cramér, H. (2016). Mathematical Methods of Statistics
(PMS-9) (Vol. 9). Princeton university press.
Kohavi, R. (1996, August). Scaling Up the Accuracy of
Naive-Bayes Classifiers: A Decision-Tree Hybrid. In
KDD (Vol. 96, pp. 202-207).
Kearns, M., & Ron, D. (1999). Algorithmic stability and
sanity-check bounds for leave-one-out cross-validation.
Neural computation, 11(6), 1427-1453.
Zou, K. H., O’Malley, A. J., & Mauri, L. (2007). Receiver-
operating characteristic analysis for evaluating
diagnostic tests and predictive models. Circulation,
115(5), 654-657.
Zhang, H., & Sheng, S. (2004, November). Learning
weighted naive Bayes with accurate ranking. In Data
Mining, 2004. ICDM'04. Fourth IEEE International
Conference on (pp. 567-570). IEEE.
Predicting 30-day Readmission in Heart Failure using Machine Learning Techniques
315