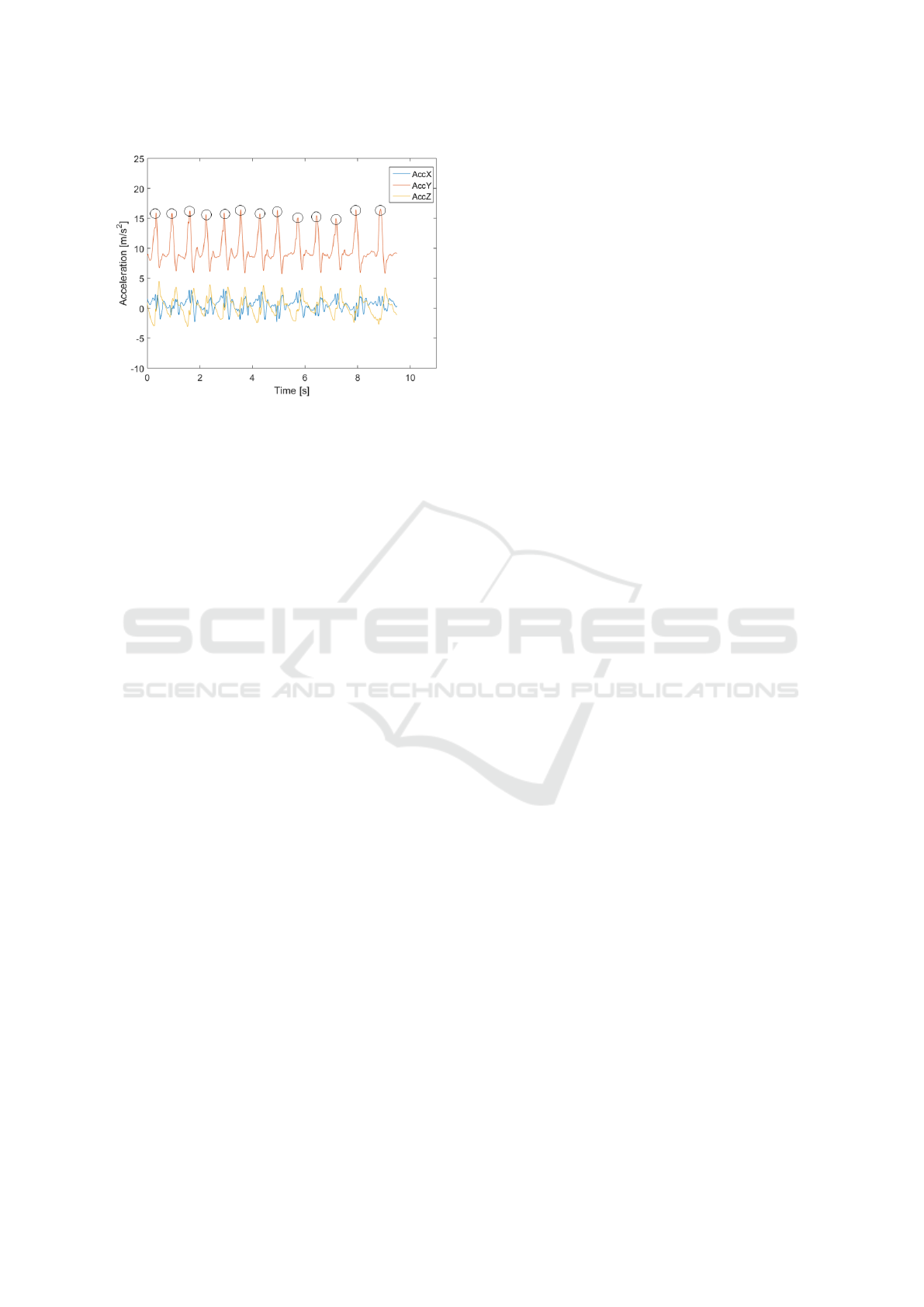
Figure 9: Acceleration in x-,y-, and z-direction during a
phase of climbing stairs in daily life.
steps and the estimated stair climb power of the ac-
tivity stair climbing. Regarding the medical mean-
ingfulness of unsupervised power measurements at
home, a comparison of the results in the laboratory
setting with results at home will follow. Within, es-
pecially the influence of diverging conditions such as
step number or the absence of a test situation will be
examined. For further validation of our stair climbing
detection in domestic environments, it is planned to
install ambient sensors at the stairways in the homes
of a subgroup of our participants.
8 CONCLUSION
Due to the importance of strength analysis to detect
functional decline in an early stage, we introduce stair
climb power measurements based on IMUs in a lab-
oratory setting. Therefore, we recognized the activity
of climbing stairs automatically via machine learning
and calculated the power based on the needed time
and covered height. Climbing stairs is correctly clas-
sified in 93% of the cases. For power calculations, we
achieved good results in comparison to conventional
measurements with a mean deviation of 2.35%. The
system’s sensitivity to detect the transition towards
frailty has been confirmed.
Additionally, we showed the general suitability of
sensor belt measurements at home and confirmed, that
phases of climbing stairs could also be recognized
during home-assessments However, further investiga-
tions of the stair usage behavior of our participants
and the recognition of stair ascending in domestic en-
vironments are planned based on one-week measure-
ments at home following the assessments. The use of
ambient sensors such as RFID technologies or light
barriers in the homes of a subgroup of our participants
is intended for further validations of the activity de-
tection and the determined duration of the stair climb-
ing activity. Especially regarding the medical rele-
vance of unsupervised home-assessments, further in-
vestigations and comparisons between laboratory and
home results are needed.
ACKNOWLEDGEMENTS
The study is funded by the German Federal
Ministry of Education and Research (Project No.
01EL1422D). The study has been approved by the
appropriate ethics committee (ethical vote: Hannover
Medical School No. 6948) and conducted in accor-
dance with the Declaration of Helsinki.
REFERENCES
Anguita, D., Ghio, A., Oneto, L., Parra, X., and Reyes-
Ortiz, J. L. (2012). Human activity recognition
on smartphones using a multiclass hardware-friendly
support vector machine. In International Workshop on
Ambient Assisted Living, pages 216–223. Springer.
Bean, J., Herman, S., Mph, D. K. K., Callahan, D., Mizer,
K., Md, W. R. F., and Fielding, R. A. (2002). Weighted
stair climbing in mobility-limited older people: a pi-
lot study. Journal of the American Geriatrics Society,
50(4):663–670.
Bean, J. F., Kiely, D. K., LaRose, S., Alian, J., and Fron-
tera, W. R. (2007). Is stair climb power a clinically
relevant measure of leg power impairments in at-risk
older adults? Archives of physical medicine and reha-
bilitation, 88(5):604–609.
Fareed, U. (2015). Smartphone sensor fusion based activ-
ity recognition system for elderly healthcare. In Pro-
ceedings of the 2015 Workshop on Pervasive Wireless
Healthcare, pages 29–34. ACM.
Fida, B., Bernabucci, I., Bibbo, D., Conforto, S., and
Schmid, M. (2015). Pre-processing effect on the accu-
racy of event-based activity segmentation and classifi-
cation through inertial sensors. Sensors, 15(9):23095–
23109.
Frenken, T., Lipprandt, M., Brell, M., G
¨
overcin, M., Wegel,
S., Steinhagen-Thiessen, E., and Hein, A. (2012).
Novel approach to unsupervised mobility assessment
tests: Field trial for atug. In Pervasive Comput-
ing Technologies for Healthcare (PervasiveHealth),
2012 6th International Conference on, pages 131–
138. IEEE.
Fudickar, S., Karth, C., Mahr, P., and Schnor, B. (2012).
Fall-detection simulator for accelerometers with in-
hardware preprocessing. In Proceedings of the 5th
International Conference on Pervasive Technologies
Related to Assistive Environments, PETRA ’12, pages
41:1–41:7. ACM.
HEALTHINF 2018 - 11th International Conference on Health Informatics
46