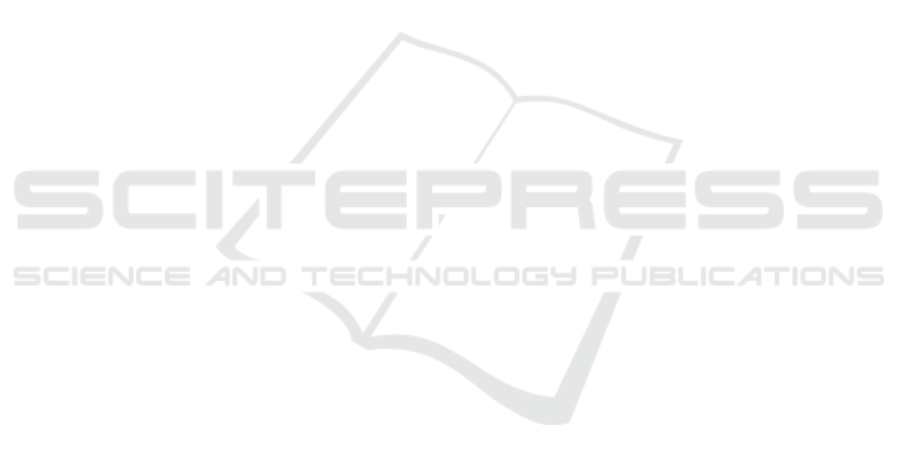
Chaudhry, R., Ofli, F., Kurillo, G., Bajcsy, R., and Vidal, R.
(2013). Bio-inspired dynamic 3d discriminative skele-
tal features for human action recognition. In CVPRW.
Dalal, N., Triggs, B., and Schmid, C. (2006). Human de-
tection using oriented histograms of flow and appea-
rance. In ECCV.
Davies, D. L. and Bouldin, D. W. (1979). A cluster separa-
tion measure. PAMI.
De Campos, T., Barnard, M., Mikolajczyk, K., Kittler, J.,
Yan, F., Christmas, W., and Windridge, D. (2011).
An evaluation of bags-of-words and spatio-temporal
shapes for action recognition. In WACV.
Du, Y., Fu, Y., and Wang, L. (2015a). Skeleton based
action recognition with convolutional neural network.
In ACPR.
Du, Y., Wang, W., and Wang, L. (2015b). Hierarchical re-
current neural network for skeleton based action re-
cognition. In CVPR.
Efros, A. A., Berg, A. C., Mori, G., Malik, J., et al. (2003).
Recognizing action at a distance. In ICCV.
Elhamifar, E., Sapiro, G., and Vidal, R. (2012). See all by
looking at a few: Sparse modeling for finding repre-
sentative objects. In CVPR.
Evangelidis, G., Singh, G., and Horaud, R. (2014). Skeletal
quads: Human action recognition using joint quadru-
ples. In ICPR.
Foggia, P., Saggese, A., Strisciuglio, N., and Vento, M.
(2014). Exploiting the deep learning paradigm for re-
cognizing human actions. In AVSS.
Frey, B. J. and Dueck, D. (2007). Clustering by passing
messages between data points. American Association
for the Advancement of Science.
Gavrila, D. (1999). The visual analysis of human mo-
vement. CVIU.
Gowayyed, M. A., Torki, M., Hussein, M. E., and El-
Saban, M. (2013). Histogram of oriented displace-
ments (hod): Describing trajectories of human joints
for action recognition. In IJCAI.
Han, F., Reily, B., Hoff, W., and Zhang, H. (2017). Space-
time representation of people based on 3d skeletal
data: A review. CVIU.
Huang, B., Tian, G., and Zhou, F. (2012). Human typical
action recognition using gray scale image of silhouette
sequence. Computers & Electrical Engineering.
Kapsouras, I. and Nikolaidis, N. (2014). Action recognition
on motion capture data using a dynemes and forward
differences representation. Journal of Visual Commu-
nication and Image Representation.
Karpathy, A., Toderici, G., Shetty, S., Leung, T., Sukthan-
kar, R., and Fei-Fei, L. (2014). Large-scale video
classification with convolutional neural networks. In
CVPR.
Kovar, L. and Gleicher, M. (2004). Automated extraction
and parameterization of motions in large data sets. In
ACM SIGGRAPH.
Laptev, I., Marszalek, M., Schmid, C., and Rozenfeld, B.
(2008). Learning realistic human actions from mo-
vies. In CVPR.
Moeslund, T. B., Hilton, A., and Kr
¨
uger, V. (2006). A sur-
vey of advances in vision-based human motion cap-
ture and analysis. CVIU.
M
¨
uller, M., R
¨
oder, T., and Clausen, M. (2005). Efficient
content-based retrieval of motion capture data. In
ACM Trans. on Graphics.
Niebles, J. C. and Fei-Fei, L. (2007). A hierarchical model
of shape and appearance for human action classifica-
tion. In CVPR.
Ofli, F., Chaudhry, R., Kurillo, G., Vidal, R., and Bajcsy, R.
(2013). Berkeley mhad: A comprehensive multimodal
human action database. In WACV.
Ofli, F., Chaudhry, R., Kurillo, G., Vidal, R., and Baj-
csy, R. (2014). Sequence of the most informative
joints (smij): A new representation for human skeletal
action recognition. Journal of Visual Communication
and Image Representation.
Papoutsakis, K., Panagiotakis, C., and Argyros, A. A.
(2017). Temporal action co-segmentation in 3d mo-
tion capture data and videos. In CVPR.
Peng, X., Wang, L., Wang, X., and Qiao, Y. (2016). Bag of
visual words and fusion methods for action recogni-
tion: Comprehensive study and good practice. CVIU.
Poppe, R. (2010). A survey on vision-based human action
recognition. Image and Vision Computing.
Presti, L. L. and Cascia, M. L. (2016). 3d skeleton-based
human action classification: A survey. Pattern Recog-
nition.
Rius, I., Gonz
`
alez, J., Varona, J., and Roca, F. X. (2009).
Action-specific motion prior for efficient bayesian 3d
human body tracking. Pattern Recognition.
Schuldt, C., Laptev, I., and Caputo, B. (2004). Recognizing
human actions: A local svm approach. In ICPR. IEEE.
Scovanner, P., Ali, S., and Shah, M. (2007). A 3-
dimensional sift descriptor and its application to
action recognition. In Proc. ACM Int. Conference on
Multimedia.
Tao, L. and Vidal, R. (2015). Moving poselets: A discrimi-
native and interpretable skeletal motion representation
for action recognition. In ICCVW.
Theodorakopoulos, I., Kastaniotis, D., Economou, G., and
Fotopoulos, S. (2014). Pose-based human action re-
cognition via sparse representation in dissimilarity
space. Journal of Visual Communication and Image
Representation.
Tibshirani, R., Walther, G., and Hastie, T. (2001). Estima-
ting the number of clusters in a data set via the gap
statistic. Journal of the Royal Statistical Society: Se-
ries B.
Vantigodi, S. and Babu, R. V. (2013). Real-time hu-
man action recognition from motion capture data. In
NCVPRIPG.
Vantigodi, S. and Radhakrishnan, V. B. (2014). Action
recognition from motion capture data using meta-
cognitive rbf network classifier. In ISSNIP.
Vemulapalli, R. and Chellapa, R. (2016). Rolling rotations
for recognizing human actions from 3d skeletal data.
In CVPR.
Vijay, P. K., Suhas, N. N., Chandrashekhar, C. S., and Dha-
nanjay, D. K. (2012). Recent developments in sign
language recognition: A review. Int. J. Adv. Comput.
Eng. Commun. Technol.
Wang, H., Kl
¨
aser, A., Schmid, C., and Liu, C.-L. (2013).
Dense trajectories and motion boundary descriptors
for action recognition. IJCV.
Weinland, D., Ronfard, R., and Boyer, E. (2011). A sur-
vey of vision-based methods for action representation,
segmentation and recognition. CVIU.
Zhu, Y., Chen, W., and Guo, G. (2013). Fusing spatiotem-
poral features and joints for 3d action recognition. In
CVPRW.
VISAPP 2018 - International Conference on Computer Vision Theory and Applications
192