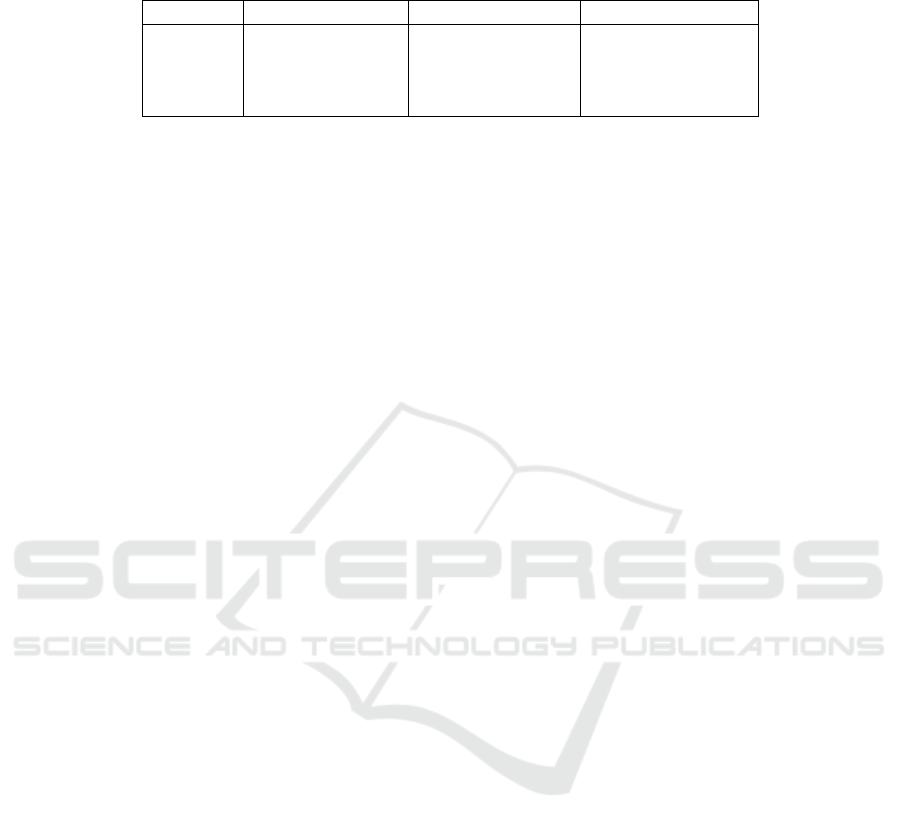
Table 1: Statistical evaluation of variability via similarity measures. * differences significant at the Holm-Bonferroni-corrected
level of p < 0.05 (for 4 tests performed).
Norms vs PD ON Norms vs PD OFF PD ON vs PD OFF
ω
vertical
0.45 0.26 < 0.01∗
ω
horizontal
0.90 0.33 0.12
ω
sagittal
< 0.01∗ < 0.01∗ 0.05
kωk 0.03 < 0.01∗ < 0.01∗
ple of subjects is sufficient. A second limitation in
this study is the number of measurements made of
each subject. Some patients had stability problems, as
is common in these patients, therefore only a limited
number of instrumented tests could be performed to
ensure that patients remained in a stable motor state.
5 CONCLUSIONS
This paper introduced and tested a new method of
stride-to-stride fluctuation using synchronized cyclo-
grams. The variability indicator is based on similarity
assessment of gait cycles. We can designate that this
method is suitable for the evaluation of gait variabil-
ity in practice. The proposed method is not limited
to gait variability assessment and would be benefi-
cial in different areas of cyclic movement variability
analysis. The quantitative analysis of wave form may
bring new knowledge of the variability with respect to
movement disorders.
ACKNOWLEDGEMENTS
This work was supported by Ministry of Health of the
Czech Republic, AZV Grant no. 16-28119a ”Analy-
sis of movement disorders for the study of extrapyra-
midal diseases mechanism using motion capture cam-
era systems”.
REFERENCES
Blin, O., Ferrandez, A., and Serratrice, G. (1990). Quanti-
tative analysis of gait in parkinson patients: increased
variability of stride length. Journal of the Neurologi-
cal Sciences, 98(1):91 – 97.
Brach, J. S., Berlin, J. E., VanSwearingen, J. M., Newman,
A. B., and Studenski, S. A. (2005). Too much or too
little step width variability is associated with a fall his-
tory in older persons who walk at or near normal gait
speed. Journal of NeuroEngineering and Rehabilita-
tion, 2:21–21.
Bryant, M. S., Rintala, D. H., Hou, J., Collins, R. L., and
Protas, E. J. (2016). Gait variability in parkinson’s
disease: levodopa and walking direction. Acta Neuro-
logica Scandinavica, 134(1):83–86.
Chau, T., Young, S., and Redekop, S. (2005). Manag-
ing variability in the summary and comparison of gait
data. Journal of NeuroEngineering and Rehabilita-
tion, 2:22–22.
Goswami, A. (2003). Kinematics quantification of gait
symmetry based on bilateral cyclograms. In P, M., B,
W., and Dunedin, Y. T., editors, Proceedings of XIX
Cong Int Soc Biomech, pages 34–43.
Grabiner, P. C., Biswas, S. T., and Grabiner, M. D. (2001).
Age-related changes in spatial and temporal gait vari-
ables. Archives of Physical Medicine and Rehabilita-
tion, 82(1):31–35.
Grieve, D. (1968). Gait patterns and the speed of walking.
Biomedical Engineering, 3(3):119–122.
Hausdorff, J. M. (2005). Gait variability: methods, model-
ing and meaning. Journal of NeuroEngineering and
Rehabilitation, 2(1):19.
Hausdorff, J. M., Cudkowicz, M. E., Firtion, R., Wei, J. Y.,
and Goldberger, A. L. (1998). Gait variability and
basal ganglia disorders: Stride-to-stride variations of
gait cycle timing in parkinson’s disease and hunting-
ton’s disease. Movement Disorders, 13(3):428–437.
Hausdorff, J. M., Forman, D. E., Ladin, Z., Goldberger,
A. L., Rigney, D. R., and Wei, J. Y. (1994). Increased
walking variability in elderly persons with congestive
heart failure. Journal of the American Geriatrics So-
ciety, 42(10):1056–1061.
Hausdorff, J. M., Herman, T., Baltadjieva, R., Gurevich, T.,
and Giladi, N. (2003). Balance and gait in older adults
with systemic hypertension *. American Journal of
Cardiology, 91(5):643–645.
Hoskovcov
´
a, M., Du
ˇ
sek, P., Sieger, T., Bro
ˇ
zov
´
a, H.,
Z
´
arubov
´
a, K., Bezd
´
ı
ˇ
cek, O.,
ˇ
Sprdl
´
ık, O., Jech, R.,
ˇ
Stochl, J., Roth, J., and R
˚
u
ˇ
zi
ˇ
cka, E. (2015). Predicting
falls in parkinson disease: What is the value of instru-
mented testing in off medication state? PLOS ONE,
10(10):1–13.
Jamour, M., Becker, C., Synofzik, M., and Maetzler, W.
(2012). Gait changes as an early indicator of de-
mentia. Zeitschrift f
¨
ur Gerontologie und Geriatrie,
45(1):40–44.
Kaipust, J. P., Huisinga, J. M., Filipi, M., and Stergiou, N.
(2012). Gait variability measures reveal differences
between multiple sclerosis patients and healthy con-
trols. Motor Control, 16(2):229–244.
Kutilek, P., Viteckova, S., Svoboda, Z., Socha, V., and
Smrcka, P. (2014). Kinematic quantification of gait
asymmetry based on characteristics of angle-angle di-
agrams. Acta Polytechnica Hungarica, 11(5):25–38.
A New Approach to Gait Variability Quantification using Cyclograms
131