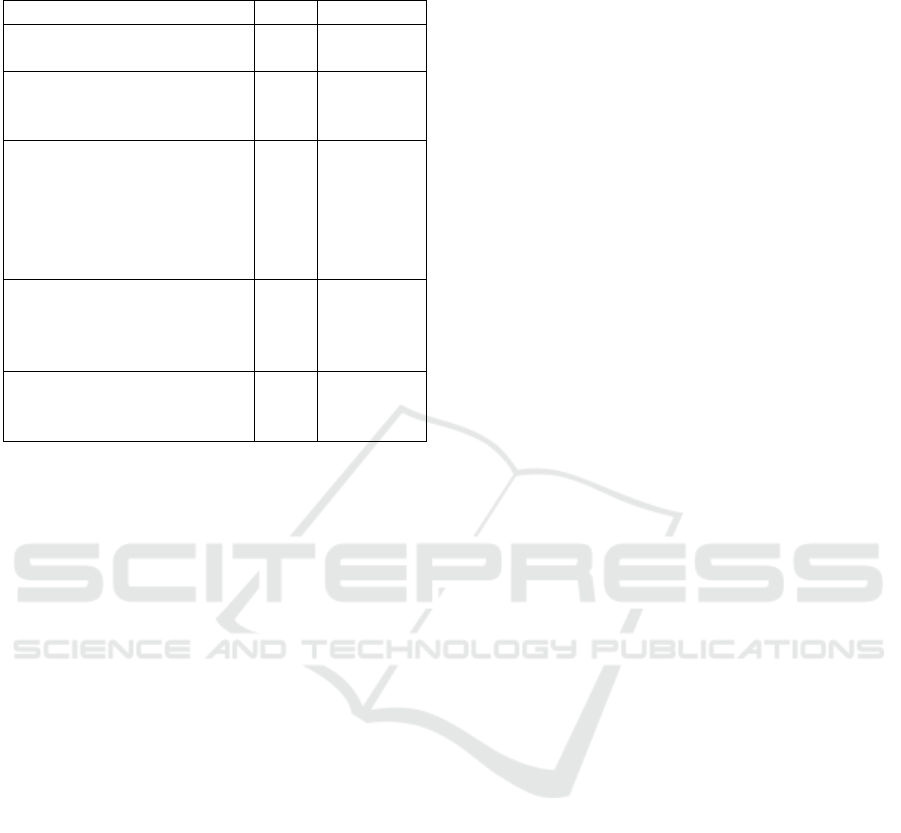
Table 5: Modified Medical Research Council Dyspnea
Scale Score.
Description of breathlessness Score Group
I get breathless only with
strenuous exercise.
0 A
I get short of breath when
hurrying on level ground or
walking up a slight hill.
1 A or B
On level ground, I walk
slower than other people my
age because of breathless-
ness, or I have to stop for
breath when walking at my
own pace.
2 B
I stop for breath after walk-
ing about 100 yards or af-
ter a few minutes on level
ground.
3 C
I am too breathless to leave
the house, or I am breathless
when getting dressed.
4 D
7 CONCLUSIONS
In this paper, we presented a smart-phone based sys-
tem to record cough, and then detect if the cough pat-
terns are indicative of COPD. Our proposed system
involves an application for recording cough, remov-
ing noise, an information gain approach for feature
selection, followed by a Random Forests based algo-
rithm for classification. We presented our results that
demonstrated high accuracy with good Precision, Re-
call and F-measure. We presented practical ideas to
further improve accuracy of classification of our algo-
rithm. Towards the end, we presented important clin-
ical applications of our proposed system for compre-
hensive in-home COPD monitoring by patients them-
selves.
ACKNOWLEDGEMENTS
This work was supported in part by the US National
Science Foundation under grants CNS 1205695, IIS
1559588 and CNS 1718071. Any opinions, thoughts
and findings are those of the authors and do not re-
flect views of the funding agency. The work was also
supported by The Florida-Georgia Louis Stokes Al-
liance for Minority Participation (FGLSAMP) Award
HRD #1612347. Furthermore, we thank the patients
and volunteers for providing cough sample data.
REFERENCES
Amalakuhan, B., Kiljanek, L., Parvathaneni, A., Hester, M.,
Cheriyath, P., and Fischman, D. (2012). A prediction
model for copd readmissions: catching up, catching
our breath, and improving a national problem. Journal
of Community Hospital Internal Medicine Perspec-
tives, 2(1).
Berkel, J. V., Dallinga, J., Mller, G., Godschalk, R., Moo-
nen, E., Wouters, E., and Schooten, F. V. (2010). A
profile of volatile organic compounds in breath dis-
criminates copd patients from controls. Respiratory
Medicine, 104(4):557 – 563.
Breiman, L. (2001). Random forests. Machine learning.
CDC (2016). Chronic obstructive pulmonary disease
(COPD). Center for Disease Control and Prevention
(CDC).
Cheffena, M. (2016). Fall detection using smartphone au-
dio features. IEEE Journal of Biomedical and Health
Informatics, 20(4):1073–1080.
Fletcher, C. M., Elmes, P. C., Fairbairn, A. S., and Wood,
C. H. (1959). Significance of respiratory symptoms
and the diagnosis of chronic bronchitis in a working
population. BMJ, 2(5147):257–266.
GOLD (2017). Global strategy for the diagnosis, manage-
ment and prevention of copd.
Hasan, M. R., Jamil, M., Rabbani, M. G., and Rahman,
M. S. (2004). Speaker identification using mel fre-
quency cepstral coefficients. variations, 1(4).
Lee, C. and Lee, G. G. (2006). Information gain
and divergence-based feature selection for machine
learning-based text categorization. Information pro-
cessing & management, 42(1):155–165.
Patel, S., Mancinelli, C., Healey, J., Moy, M., and Bonato,
P. (2009). Using wearable sensors to monitor physical
activities of patients with copd: A comparison of clas-
sifier performance. In 2009 Sixth International Work-
shop on Wearable and Implantable Body Sensor Net-
works, pages 234–239.
Phillips, C. O., Syed, Y., Parthalin, N. M., Zwiggelaar, R.,
Claypole, T. C., and Lewis, K. E. (2012). Machine
learning methods on exhaled volatile organic com-
pounds for distinguishing copd patients from healthy
controls. Journal of Breath Research, 6(3):036003.
Pramono, R. X. A., Imtiaz, S. A., and Rodriguez-Villegas,
E. (2016). A cough-based algorithm for automatic di-
agnosis of pertussis. PLOS ONE, 11(9):1–20.
Sun, X., Lu, Z., Hu, W., and Cao, G. (2015). Symdetector:
Detecting sound-related respiratory symptoms using
smartphones. In Proceedings of the 2015 ACM Inter-
national Joint Conference on Pervasive and Ubiqui-
tous Computing, UbiComp ’15, pages 97–108, New
York, NY, USA. ACM.
WHO (2016). Chronic obstructive pulmonary disease
(COPD).
Zhang X, Holt JB, L. H. e. a. (2014). Multilevel re-
gression and poststratification for small area estima-
tion of population health outcomes: a case study
of chronic obstructive pulmonary disease prevalence
using BRFSS. American Journal of Epidemiology,
179(8):1025–1033.
On Detecting Chronic Obstructive Pulmonary Disease (COPD) Cough using Audio Signals Recorded from Smart-Phones
337