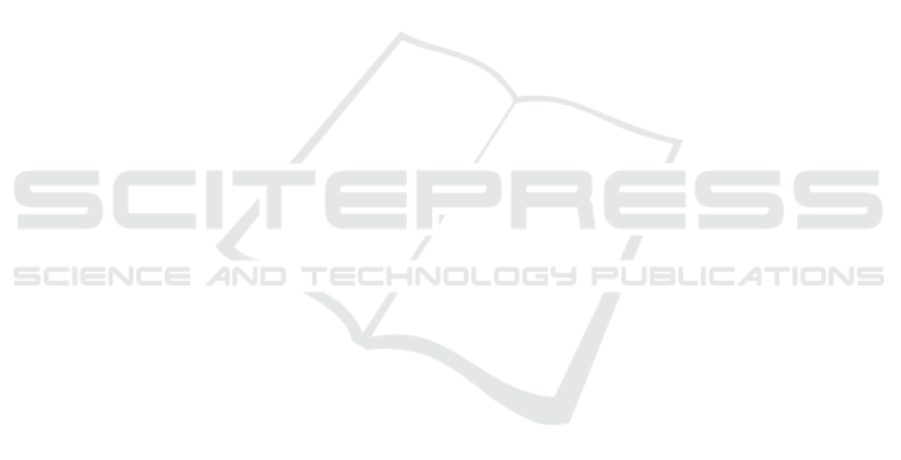
After the calculations of the likelihood per
phoneme, a scheme representing these likelihoods is
constructed (Geitgey, 2016). This is the last step as
shown in Figure 3. In this example, the word ‘hello’
is constructed. The predictions of this scheme are
sequenced with double characters and gaps in the
word. When these are filtered, three words are still
possible: ‘hello’, ‘hullo’ and ‘aullo’. Since ‘hello’ is
more likely because it occurs more often in the
database than the other two options, ‘hello’ is chosen
(Geitgey, 2016). In case another word was meant, the
user has to correct it manually. This improvement will
then be saved for future predictions (Renckens,
2009).
2.2 Pros and Cons of Speech
Technology
In this section we state the pros and cons according to
literature enlightened by interviewees. The main
advantage of speech technology is time reduction
(Ajami, 2016; Koivikko, Kauppinen, and Ahovuo,
2008). According to a study of Nuance, people can
type 40 words per minute at best, whereas people can
speak 120 words per minute (Nuance, 2008).
Furthermore, Nuance (2015) states that doctors are
documenting 13.3 hours a week on average. For
nurses, this is 8.7 hours per week (Nuance, 2015).
This concerns an estimated 30% of the working week,
therefore speech technology could be very profitable.
Different studies show that radiology and
pathology benefit most from speech technology
(Ajami, 2016; Johnson et al., 2014). This is clarified
by the fact that radiology and pathology can cut down
on their secretaries when they start using speech
recognition, which leads to a decrease in the report
turnaround time (RTT) (Koivikko, Kauppinen, and
Ahovuo, 2008). Other departments started working
with the Electronic Health Record (EHR) before
speech technology, and already cut down on their
secretaries. Because of this, speech technology lacks
this benefit for departments other than radiology and
pathology, including the decrease in RTT and the
financial benefits of the staffing costs (M1).
Before doctors can start using speech technology,
a profile must be prepared whereby the system gets
familiar with the user’s speech and vocabulary. This
can be done by reading a text aloud (Bosch, 2005).
This is beneficial for the accuracy of the system
(Vervoort, 2017), but takes time (Ajami, 2016;
Johnson, et al., 2014). Speech technology uses a
lexicon, as described in paragraph 2.1. For medical
staff, medical terminology is added, but not
terminology that is used in daily life (S2). A
disadvantage of this dictionary holds that words that
are not included, cannot be recognized by the system
(S2). Patient friendliness increases (Ajami, 2016).
When a doctor types during a conversation, he or she
has less attention for the patient. Using speech
technology, he or she can listen to the patient without
this distraction (U1). The doctor has to dictate during
the conversation, or afterwards, since it is not (yet)
possible for software to recognize two voices at once,
i.e. Advanced Voice Technology (Tuin, 2016).
Besides, reports are available faster (Ajami, 2016)
(Johnson, et al., 2014; Koivikko, Kauppinen, and
Ahovuo, 2008), therefore patients can be cured faster,
which leads to an increased quality of patient care
(Koivikko, Kauppinen, and Ahovuo, 2008; Parente,
Kock, and Sonsini, 2004). A challenge for
implementing speech technology is the human factor
(Ajami, 2016; Dawson et al., 2014; Parente, Kock,
and Sonsini, 2004). Doctors need to adapt their way
of working and this often leads to problems (Dawson,
et al., 2014). To avoid this, intensive support is
needed (S2; Ajami, 2016). An overview of all found
pros and cons in literature is represented in Table 1.
3 METHODS
For this study we performed a literature review and a
qualitative study. We searched PubMed, Springerlink
and Elsevier for finding the relevant articles. The
following key words and/or their combinations are
used: speech recognition, health care,
spraaktechnologie, spraakherkenning, zorg, medisch,
pros, advantages, cons, werking, neural network,
acoustic model, akoestisch model and Hidden Markov
Model. While selecting articles, we focused on the
publication date and Citation index.
The data for the qualitative study were gathered
by performing ten semi-structured interviews. We
used a structured topic list and an operational model
to establish the topics of the interviews and
corresponding questions. The participants consisted
of four managers working at two different hospitals,
four suppliers of speech technology working at
different companies, and two users of speech
technology with different professions. An overview
of the participants can be found in Table 2.
HEALTHINF 2018 - 11th International Conference on Health Informatics
342