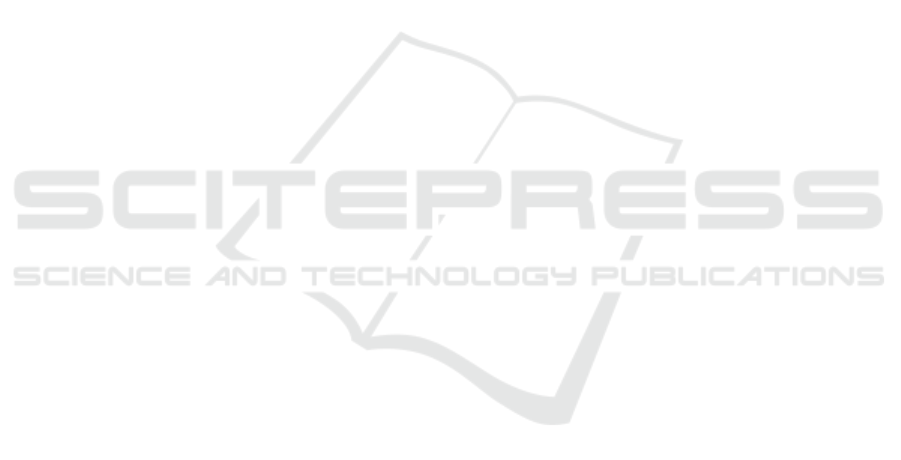
In figure 6, the contribution of each band is
evaluated in the construction of the RF model with all
bands. The Green G, the Saturation S, and the
Saturation neighbours S15 are among those who
contribute the most but the highest contribution is
made by the F11 fluorescence band. This band
expresses the chlorophyll content of the plant as
described in (Gitelson et al., 1999), which explains
the high contribution of this band in the classification
of plant versus soil with no chlorophyll and algae and
moss with different chlorophyll composition. The
bands H15, S15 and V15 are also highly contributing
to the RF models constructions. These bands could
also be interpreted as noise reduced HSV signals, and
we can notice that the contribution of S15 was even
bigger than the contribution of S which indicates the
importance of its use.
4 CONCLUSIONS
As a conclusion, both used supervised machine
learning algorithms: SVM and RF approaches
succeeded to provide a useful tool for the plant
segmentation in the presence of challenging
background containing algae and moss. RF
approaches gave better results than the SVM
methods. The use of multiple bands showed that the
performance of the algorithms improves the
segmentation results especially with the SVM
models. Within the RF approach, bands contribution
to the final results vary and the highest contribution is
the ratio fluorescence which highlights the role of
these bands in such machine learning approaches. In
addition, the neighbourhood information introduced
by the channels H15, S15, and V15 contributed
considerably to the construction of the model and to
the improvement of the segmentation results.
One limitation of this approach is that it is based
on the expert knowledge constructing the data needed
for training the model (supervised machine learning).
In this sense, bad quality labelled image (a bad expert
segmentation) will result in a bad model for
segmentation.
To avoid this dependence, we will develop, in
future work, unsupervised models for the segmenta-
tion of the plants. Moreover, more features will be
included in the classification parameters such as
texture features to improve the segmentation results.
We will also focus on the plant 3D acquisition to
obtain more data (such as height and volume)
describing its responses to the environment (drought,
nutrients, biotic stress). These 3D information will
also be helpful for the segmentation and the time
tracking of individual plant leaves.
ACKNOWLEDGEMENTS
The authors thank Joke Belza for her help with the
manual segmentation of the data.
This work was financed by TIMESCALE, a
HORIZON 2020 project, and Ghent University.
REFERENCES
Arend D., Lange M., Pape J-M., Weigelt-Fischer K.,
Fernando Arana-Ceballos, Mücke I., Klukas C.,
Altmann T., Scholz U., and Junker A. 2016, “Data
Descriptor: Quantitative monitoring of Arabidopsis
thaliana growth and development using high-
throughput plant phenotyping”, Scientific Data 3,
Article number: 160055.
Åstrand B., and Johansson M. 2006; Segmentation of
partially occluded plant leaves; 13th International
Conference on Systems, Signals and Image Processing;
September 21-23, 2006, Budapest, Hungary.
Baker N. R. 2008, Chlorophyll Fluorescence: A Probe of
Photosynthesis In Vivo, Annual Review of Plant
Biology, 59:89-113.
Breiman L. 2001, “Random Forests”, Machine Learning,
pp 5–32, October 2001, Volume 45, Issue 1.
Cortes C., Vapnik V. 1995, “Support-Vector Networks”,
Machine Learning, 20, 273-297.
Gitelson A. A., Buschmann, C., and Lichtenthaler H. K.
1999; The Chlorophyll Fluorescence Ratio F735/F700
as an Accurate Measure of the Chlorophyll Content in
Plants, Remote Sensing Environment 69:296–302.
Navarro P. J., Pérez F., Weiss J., and Egea-Cortines M.
2016, “Machine Learning and Computer Vision System
for Phenotype Data Acquisition and Analysis in
Plants”, Sensors (Basel). 2016 May; 16(5): 641.
Scharr H., Minervini M., French A. P., Klukas Ch., Kramer
D. M., Liu X., Luengo I., Pape J-M., Polder G.,
Vukadinovic D., Yin X., and Tsaftaris S. A. 2016, “Leaf
segmentation in plant phenotyping: a collation study”,
Machine Vision and Applications 27:585–606.
De Vylder J., Vandenbussche F., Hu Y., Philips W., and
Van Der Straeten D. 2012, “Rosette Tracker: An Open
Source Image Analysis Tool for Automatic
Quantification of Genotype Effects.”, Plant Physiology,
November 2012, Vol. 160, pp. 1149–1159.