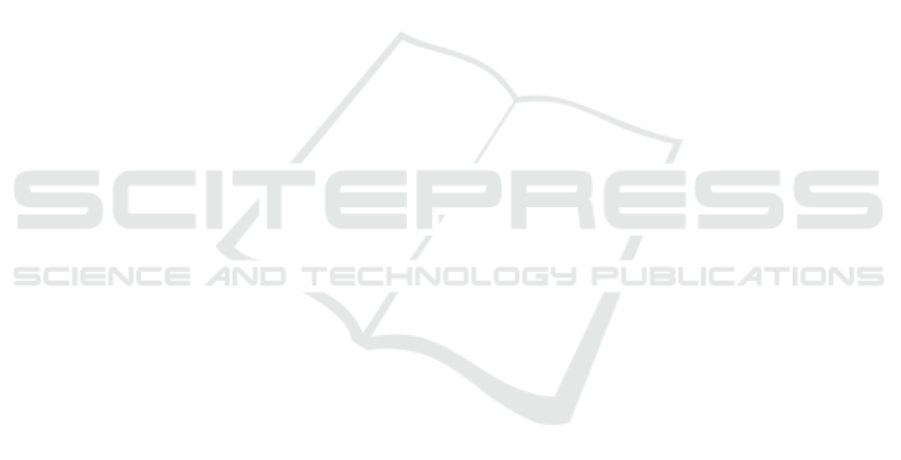
sed using an adaptive vertical median filter. Experi-
mental results conducted on the Middlebury datasets
have demonstrated the accuracy and the efficiency of
the proposed method.
REFERENCES
Achanta, R., Shaji, A., Smith, K., Lucchi, A., Fua, P., and
Ssstrunk, S. (2012). Slic superpixels compared to
state-of-the-art superpixel methods. IEEE Transacti-
ons on Pattern Analysis and Machine Intelligence,
34(11):2274–2282.
Bobick, A. F. and Intille, S. S. (1999). Large occlusion
stereo. International Journal of Computer Vision,
33(3):181–200.
Hawe, S., Kleinsteuber, M., and Diepold, K. (2011). Dense
disparity maps from sparse disparity measurements.
In 2011 International Conference on Computer Vi-
sion, pages 2126–2133.
Hirschmuller, H. (2008). Stereo processing by semiglobal
matching and mutual information. IEEE Transacti-
ons on Pattern Analysis and Machine Intelligence,
30(2):328–341.
Hirschmuller, H. and Scharstein, D. (2009). Evaluation
of stereo matching costs on images with radiometric
differences. IEEE Trans. Pattern Anal. Mach. Intell.,
31(9):1582–1599.
Hsieh, Y. C., McKeown, D. M., and Perlant, F. P. (1992).
Performance evaluation of scene registration and
stereo matching for cartographic feature extraction.
IEEE Transactions on Pattern Analysis and Machine
Intelligence, 14(2):214–238.
Kang, S. B., Webb, J. A., Zitnick, C. L., and Kanade, T.
(1995). A multibaseline stereo system with active illu-
mination and real-time image acquisition. In Procee-
dings of IEEE International Conference on Computer
Vision, pages 88–93.
Kim, S., Ham, B., Ryu, S., Kim, S. J., and Sohn, K. (2015).
Robust Stereo Matching Using Probabilistic Lapla-
cian Surface Propagation, pages 368–383. Springer
International Publishing, Cham.
Kolmogorov, V. and Zabih, R. (2001). Computing vi-
sual correspondence with occlusions using graph cuts.
In Computer Vision, 2001. ICCV 2001. Proceedings.
Eighth IEEE International Conference on, volume 2,
pages 508–515 vol.2.
Liu, L. K., Chan, S. H., and Nguyen, T. Q. (2015). Depth
reconstruction from sparse samples: Representation,
algorithm, and sampling. IEEE Transactions on Image
Processing, 24(6):1983–1996.
Miron, A., Ainouz, S., Rogozan, A., and Bensrhair, A.
(2014). A robust cost function for stereo matching of
road scenes. Pattern Recognition Letters, 38:70–77.
Mukherjee, S. and Guddeti, R. M. R. (2014). A hybrid al-
gorithm for disparity calculation from sparse disparity
estimates based on stereo vision. In 2014 Internatio-
nal Conference on Signal Processing and Communi-
cations (SPCOM), pages 1–6.
Scharstein, D. and Szeliski, R. (2002). A taxonomy and
evaluation of dense two-frame stereo correspondence
algorithms. International Journal of Computer Vision,
47:7–42.
Schauwecker, K., Klette, R., and Zell, A. (2012). A new
feature detector and stereo matching method for accu-
rate high-performance sparse stereo matching. In
2012 IEEE/RSJ International Conference on Intelli-
gent Robots and Systems, pages 5171–5176.
Stentoumis, C., Grammatikopoulos, L., Kalisperakis, I.,
and Karras, G. (2014). On accurate dense stereo-
matching using a local adaptive multi-cost appro-
ach. {ISPRS} Journal of Photogrammetry and Remote
Sensing, 91:29 – 49.
Sun, J., Zheng, N.-N., and Shum, H.-Y. (2003). Stereo
matching using belief propagation. IEEE Transacti-
ons on Pattern Analysis and Machine Intelligence,
25(7):787–800.
Sun, X., Mei, X., Jiao, S., Zhou, M., and Wang, H. (2011).
Stereo matching with reliable disparity propagation.
In 2011 International Conference on 3D Imaging, Mo-
deling, Processing, Visualization and Transmission,
pages 132–139.
Veksler, O. (2005). Stereo correspondence by dynamic pro-
gramming on a tree. In 2005 IEEE Computer Society
Conference on Computer Vision and Pattern Recogni-
tion (CVPR’05), volume 2, pages 384–390 vol. 2.
Yamaguchi, K., Hazan, T., McAllester, D., and Urtasun, R.
(2012). Continuous markov random fields for robust
stereo estimation. In Proceedings of the 12th Euro-
pean Conference on Computer Vision - Volume Part V,
ECCV’12, pages 45–58, Berlin, Heidelberg. Springer-
Verlag.
Yamaguchi, K., McAllester, D., and Urtasun, R. (2014). Ef-
ficient Joint Segmentation, Occlusion Labeling, Stereo
and Flow Estimation, pages 756–771. Springer Inter-
national Publishing, Cham.
Yan, J., Yu, Y., Zhu, X., Lei, Z., and Li, S. Z. (2015). Object
detection by labeling superpixels. In 2015 IEEE Con-
ference on Computer Vision and Pattern Recognition
(CVPR), pages 5107–5116.
Yoon, K.-J. and Kweon, I. S. (2006). Adaptive support-
weight approach for correspondence search. IEEE
Transactions on Pattern Analysis and Machine Intel-
ligence, 28(4):650–656.
Zabih, R. and Woodfill, J. (1994). Non-parametric lo-
cal transforms for computing visual correspondence.
In Proceedings of the Third European Conference on
Computer Vision (Vol. II), ECCV ’94, pages 151–158,
Secaucus, NJ, USA. Springer-Verlag New York, Inc.
Zhang, K., Lu, J., and Lafruit, G. (2009). Cross-based lo-
cal stereo matching using orthogonal integral images.
IEEE Trans. Circuits Syst. Video Techn., 19(7):1073–
1079.
VISAPP 2018 - International Conference on Computer Vision Theory and Applications
540