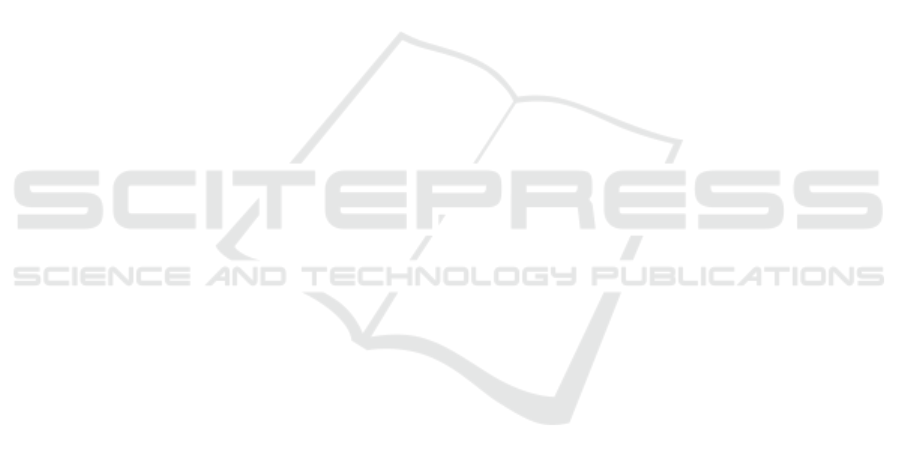
REFERENCES
Buxton, R. B. (2009). Introduction to functional magnetic
resonance imaging: principles and techniques.
Cambridge university press.
Cho, S., Jones, D., Reddick, W. E., Ogg, R. J., & Steen, R.
G. (1997). Establishing norms for age-related changes
in proton T1 of human brain tissue in vivo. Magnetic
resonance imaging, 15(10), 1133-1143.
Courchesne, E., Chisum, H. J., Townsend, J., Cowles, A.,
Covington, J., Egaas, B.& Press, G. A. (2000). Normal
brain development and aging: quantitative analysis at in
vivo MR imaging in healthy volunteers. Radiology,
216(3), 672-682.
Davatzikos, C., & Resnick, S. M. (2002). Degenerative age
changes in white matter connectivity visualized in vivo
using magnetic resonance imaging. Cerebral cortex,
12(7), 767-771.
Deoni, S. C., Peters, T. M., & Rutt, B. K. (2005). High‐
resolution T1 and T2 mapping of the brain in a
clinically acceptable time with DESPOT1 and
DESPOT2. Magnetic resonance in medicine, 53(1),
237-241.
Desmond, K. L., Al‐Ebraheem, A., Janik, R., Oakden, W.,
Kwiecien, J. M., Dabrowski, W. & Bock, N. A. (2016).
Differences in iron and manganese concentration may
confound the measurement of myelin from R1 and R2
relaxation rates in studies of dysmyelination. NMR in
Biomedicine, 29(7), 985-998.
Destrieux, C., Fischl, B., Dale, A., & Halgren, E. (2010).
Automatic parcellation of human cortical gyri and sulci
using standard anatomical nomenclature. Neuroimage,
53(1), 1-15.
Ertan, T., & Eker, E. (2000). Reliability, validity, and factor
structure of the geriatric depression scale in Turkish
elderly: are there different factor structures for different
cultures?. International Psychogeriatrics, 12(2), 163-
172.
Fischl, B., Salat, D. H., van der Kouwe, A. J., Makris, N.,
Ségonne, F., Quinn, B. T., & Dale, A. M. (2004).
Sequence-independent segmentation of magnetic
resonance images. Neuroimage, 23, S69-S84.
Ge, Y., Grossman, R. I., Babb, J. S., Rabin, M. L., Mannon,
L. J., & Kolson, D. L. (2002). Age-related total gray
matter and white matter changes in normal adult brain.
Part I: volumetric MR imaging analysis. American
journal of neuroradiology, 23(8), 1327-1333.
Gracien, R. M., Nürnberger, L., Hok, P., Hof, S. M., Reitz,
S. C., Rüb, U. & Baudrexel, S. (2017). Evaluation of
brain ageing: a quantitative longitudinal MRI study
over 7 years. European radiology, 27(4), 1568-1576.
Lu, H., Nagae‐Poetscher, L. M., Golay, X., Lin, D.,
Pomper, M., & van Zijl, P. (2005). Routine clinical
brain MRI sequences for use at 3.0 Tesla. Journal of
Magnetic Resonance Imaging, 22(1), 13-22.
Marques, J. P., Kober, T., Krueger, G., van der Zwaag, W.,
Van de Moortele, P. F., & Gruetter, R. (2010).
MP2RAGE, a self bias-field corrected sequence for
improved segmentation and T 1-mapping at high field.
Neuroimage, 49(2), 1271-1281.
Ogg, R. J., & Steen, R. G. (1998). Age‐related changes in
Brain T1 are correlated with iron concentration.
Magnetic resonance in medicine, 40(5), 749-753.
Okubo, G., Okada, T., Yamamoto, A., Fushimi, Y., Okada,
T., Murata, K., & Togashi, K. (2017). Relationship
between aging and T1 relaxation time in deep gray
matter: A voxel‐based analysis. Journal of Magnetic
Resonance Imaging.
Resnick, S. M., Goldszal, A. F., Davatzikos, C., Golski, S.,
Kraut, M. A., Metter, E. J., ... & Zonderman, A. B.
(2000). One-year age changes in MRI brain volumes in
older adults. Cerebral cortex, 10(5), 464-472.
Saito, N., Sakai, O., Ozonoff, A., & Jara, H. (2009). Relaxo-
volumetric multispectral quantitative magnetic
resonance imaging of the brain over the human lifespan:
global and regional aging patterns. Magnetic resonance
imaging, 27(7), 895-906.
Salat DH, Buckner RL, Snyder AZ, Greve DN, Desikan RS,
Busa E, Morris JC, Dale AM, and Fischl B. (2004).
Thinning of the cerebral cortex in aging. Cereb. Cortex
Salat, D. H., Lee, S. Y., Van der Kouwe, A. J., Greve, D.
N., Fischl, B., & Rosas, H. D. (2009). Age-associated
alterations in cortical gray and white matter signal
intensity and gray to white matter contrast.
Neuroimage, 48(1), 21-28.
Smith, S. M. (2002). Fast robust automated brain
extraction. Human brain mapping, 17(3), 143-155.
Stikov, N., Boudreau, M., Levesque, I. R., Tardif, C. L.,
Barral, J. K., & Pike, G. B. (2015). On the accuracy of
T1 mapping: searching for common ground. Magnetic
resonance in medicine, 73(2), 514-522.
Talairach J, Tournoux P (1988). Co-planar stereotaxic atlas
of the human brain. Thieme, New York.
Tau, G. Z., & Peterson, B. S. (2010). Normal development
of brain circuits. Neuropsychopharmacology, 35(1),
147-168..
Thambisetty, M., Wan, J., Carass, A., An, Y., Prince, J. L.,
& Resnick, S. M. (2010). Longitudinal changes in
cortical thickness associated with normal aging.
Neuroimage, 52(4), 1215-1223.
Van Waesberghe, J. H. T. M., Kamphorst, W., De Groot, C.
J., Van Walderveen, M. A., Castelijns, J. A., Ravid, R.
& Barkhof, F. (1999). Axonal loss in multiple sclerosis
lesions: magnetic resonance imaging insights into
substrates of disability. Annals of neurology, 46(5),
747-754.
Wansapura, J. P., Holland, S. K., Dunn, R. S., & Ball, W.
S. (1999). NMR relaxation times in the human brain at
3.0 tesla. Journal of magnetic resonance imaging, 9(4),
531-538.
Zhang, Y., Brady, M., & Smith, S. (2001). Segmentation of
brain MR images through a hidden Markov random
field model and the expectation-maximization
algorithm. IEEE transactions on medical imaging,
20(1), 45-57.
BIOSIGNALS 2018 - 11th International Conference on Bio-inspired Systems and Signal Processing
154