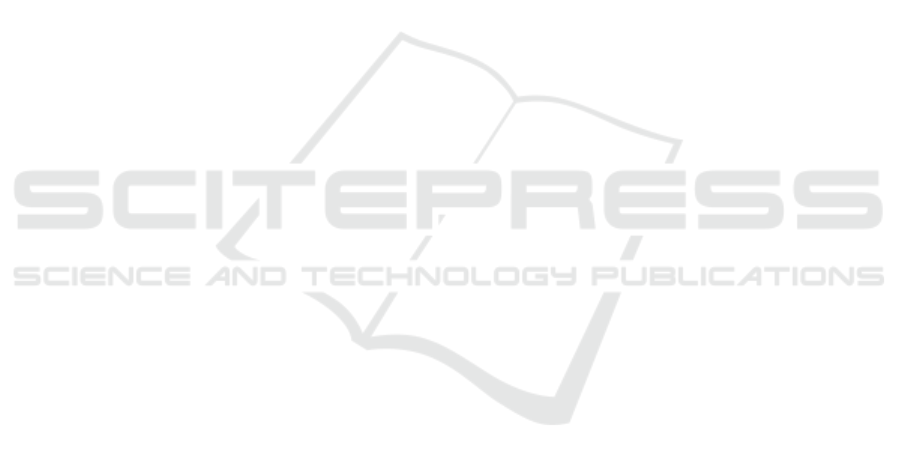
The research presented in this paper was partially
funded by the Regional Government of Andalusia
(Junta de Andalucía) under grant number TIC-7629
and Spanish Ministry of Education and Science
(Grant Number: TIN2009-14057-C03-03).
REFERENCES
Ferlay, J., Steliarova-Foucher, E., Lortet-Tieulent, J.,
Rosso, S., Coebergh, J.W.W., Comber, H., Forman, D.
and Bray, F., 2013. Cancer incidence and mortality
patterns in Europe: estimates for 40 countries in 2012.
European Journal of Cancer 49(6), 1374-1403.
American Cancer Society, September 2014.
http://www.cancer.org/cancer/
prostatecancer/detailedguide/prostate-cancer-key-
statistics.
Valeriani, M., Carnevale, A., Osti, M.F., de Sanctis, V.,
Agolli, L. and Enrici, R.M., 2013. Hypofractionated
intensity-modulated simultaneous integrated boost and
image-guided radiotherapy in the treatment of high-
risk prostate cancer patients: a preliminary report on
acute toxicity. Tumori 99(4), 474-479.
Morimoto, M., Yoshioka, Y., Konishi, K., Isohashi, F.,
Takahashi, Y., Ogata, T., Koizumi, M., Teshima, T.,
Bijl, H.P., van der Schaaf, A., Langendijk, J.A. and
Ogawa, K., 2014. Comparison of acute and subacute
genitourinary and gastrointestinal adverse events of
radiotherapy for prostate cancer using intensity-
modulated radiation therapy, three-dimensional
conformal radiation therapy, permanent implant
brachytherapy and high-dose-rate brachytherapy.
Tumori 100(3), 265-271.
Agrawal, R., Imielinski, T. and Swami, A., 1993. Mining
Association Rules between Sets of Items in Large
Databases. In Proceedings of ACM SIGMOD ICMD,
207-216.
Rudin, C., Letham, B. and Madigan, D., 2013. Learning
theory analysis for association rules and sequential
event prediction. Journal of Machine Learning
Research, 14:3441-3492.
Wrobel, S., 1997. An algorithm for multi-relational
discovery of subgroups. Principles of data mining and
knowledge discovery, 78-87.
Gamberger, D., Lavrac, N. and Krstacic, G., 2003. Active
Subgroup Mining: A Case Study in Coronary Heart
Disease Risk Group Detection. Artificial Intelligence
in Medicine 28, 27–57.
Domínguez-Olmedo, J. L., Mata, J. and Pachón, V., 2015.
Deterministic Extraction of Compact Sets of Rules for
Subgroup Discovery. In Proceedings of Intelligent
Data Engineering and Automated Learning – IDEAL,
138-145.
Hastie, T., Tibshirani, R. and Friedman, J., 2009. The
Elements of Statistical Learning: Data Mining,
Inference, and Prediction. Springer-Verlag.
Golhar, M., Iwahori, Y., K. Bhuyan, M., Funahashi, K.
and Kasugai, K., 2017. A Robust Method for Blood
Vessel Extraction in Endoscopic Images with SVM-
based Scene Classification. In Proceedings of the 6th
International Conference on Pattern Recognition
Applications and Methods: ICPRAM, 148-156.
Cortés, X., Serratosa, F. and Moreno-García, C., 2015. An
Interactive Model for Structural Pattern Recognition
based on the Bayes Classifier. In Proceedings of the
International Conference on Pattern Recognition
Applications and Methods: ICPRAM, 240-247.
Liu, B., Hsu, W. and Ma, Y., 1998. Integrating
classification and association rule mining. In
Proceedings of Fourth International Conference on
Knowledge Discovery and Data Mining.
Sun, Y., Wong, A.K.C., Kamel, M.S., 2009. Classification
of imbalanced data: a review. International Journal of
Pattern Recognition and Artificial Intelligence 23(4),
687–719.
Rastgoo, M., Lemaitre, G., Massich, J., Morel, O.,
Marzani, F., Garcia, R. and Meriaudeau, F.,
2016. Tackling the Problem of Data Imbalancing for
Melanoma Classification . In Proceedings of the 9th
International Joint Conference on Biomedical
Engineering Systems and Technologies:
BIOIMAGING (BIOSTEC).
Domínguez-Olmedo, J. L., Mata, J., Pachón, V. and Maña,
M., 2011. A deterministic approach to association rule
mining without attribute discretization. In Proceedings
of the International Conference on Digital Information
Processing and Communications, 188, 140–150.
Domínguez-Olmedo, J. L., Mata, J., Pachón, V. and Maña,
M., 2012. Rule extraction from medical data without
discretization of numerical attributes. In Proceedings
of the International Conference on Health Informatics
(HEALTHINF), 397-400.
Domínguez-Olmedo, J.L. and Mata, J., 2016. Comparison
of Standard Discretization with a New Method for
Quantitative Association Rules. IEEE Latin America
Transactions 14(4), 1879-1885.
Grosskreutz, H. and Ruping, S., 2009. On Subgroup
Discovery in Numerical Domains. Data Mining and
Knowledge Discovery 19(2), 210–226.
Frank, E. and Witten, I. H., 1998. Generating Accurate
Rule Sets without Global Optimization. In
Proceedings of the Fifteenth International Conference
on Machine Learning, 144-151.
Rumelhart, D. E., Hinton, G. E. and Williams, R. J., 1986.
Parallel distributed processing: explorations in the
microstructure of cognition. MIT Press Cambridge,
318-362.
Quinlan, R., 1993. C4.5: Programs for Machine Learning.
Morgan Kaufmann Publishers, San Mateo.
Breiman, L., 2001. Random Forests. Machine Learning
45(1), 5-32.
Pearl, J., 1985. Bayesian Networks: A Model of Self-
Activated Memory for Evidential Reasoning. UCLA
Computer Science Department.
John, G. H. and Langley, P., 1995. Estimating Continuous
Distributions in Bayesian Classifiers. In Proceedings
of the Eleventh Conference on Uncertainty in Artificial
Intelligence, 338-345.
HEALTHINF 2018 - 11th International Conference on Health Informatics
154